Dissociable default-mode subnetworks subserve childhood attention and cognitive flexibility: Evidence from deep learning and stereotactic electroencephalography.
biorxiv(2023)
摘要
Cognitive flexibility encompasses the ability to efficiently shift focus and forms a critical component of goal-directed attention. The neural substrates of this process are incompletely understood in part due to difficulties in sampling the involved circuitry. We leverage stereotactic intracranial recordings to directly resolve local-field potentials from otherwise inaccessible structures to study moment-to-moment attentional activity in children with epilepsy performing a flexible attentional task. On an individual subject level, we employed deep learning to decode neural features predictive of task performance indexed by single-trial reaction time. These models were subsequently aggregated across participants to identify predictive brain regions based on AAL atlas and FIND functional network parcellations. Through this approach, we show that fluctuations in beta (12-30 Hz) and gamma (30-80 Hz) power reflective of increased top-down attentional control and local neuronal processing within relevant large-scale networks can accurately predict single-trial task performance. We next performed connectomic profiling of these highly predictive nodes to examine task-related engagement of distributed functional networks, revealing exclusive recruitment of the dorsal default mode network during shifts in attention. The identification of distinct substreams within the default mode system supports a key role for this network in cognitive flexibility and attention in children. Furthermore, convergence of our results onto consistent functional networks despite significant inter-subject variability in electrode implantations supports a broader role for deep learning applied to intracranial electrodes in the study of human attention.
更多查看译文
关键词
Deep learning,ADHD,Attention,Shapley values,Default mode network
AI 理解论文
溯源树
样例
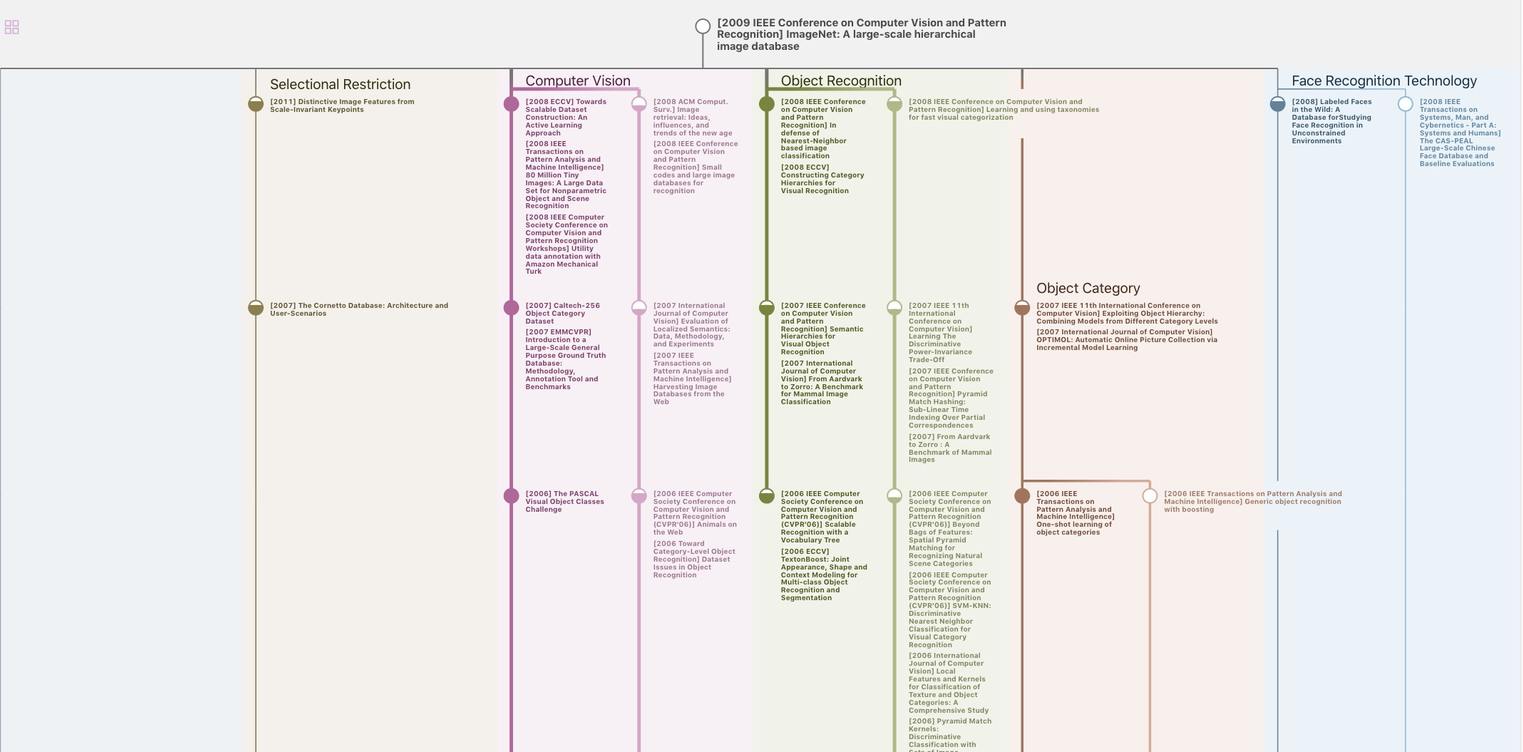
生成溯源树,研究论文发展脉络
Chat Paper
正在生成论文摘要