LazyPair: scalable prediction of protein-protein interactions and interaction types
biorxiv(2022)
摘要
Motivation Almost all cellular processes require protein-protein interactions. Common interaction types include binding, post-translational modifications, and catalysis. However, existing prediction tools do not take these interaction types into account and do not scale well on proteome-wide prediction.
Results Here we show that a random forest classifier trained on per-residue physicochemical and biochemical properties is useful for predicting protein-protein interactions. Counterintuitively, we find that training random forests by individual interaction types improves accuracy. Furthermore, a combination of these specialised classifiers improves generalisability. We call our protein-protein interaction prediction tool LazyPair. More importantly, LazyPair outperforms the state-of-the-art in accuracy, generalisability and scalability.
Availability and implementation LazyPair and the source code and data for reproducing our analysis are freely available at [https://github.com/Gardner-BinfLab/PPI\_Analysis\_2022][1] and . The web server version and the source code are freely available at and , respectively.
### Competing Interest Statement
The authors have declared no competing interest.
[1]: https://github.com/Gardner-BinfLab/PPI_Analysis_2022
更多查看译文
关键词
interactions,scalable prediction,protein-protein
AI 理解论文
溯源树
样例
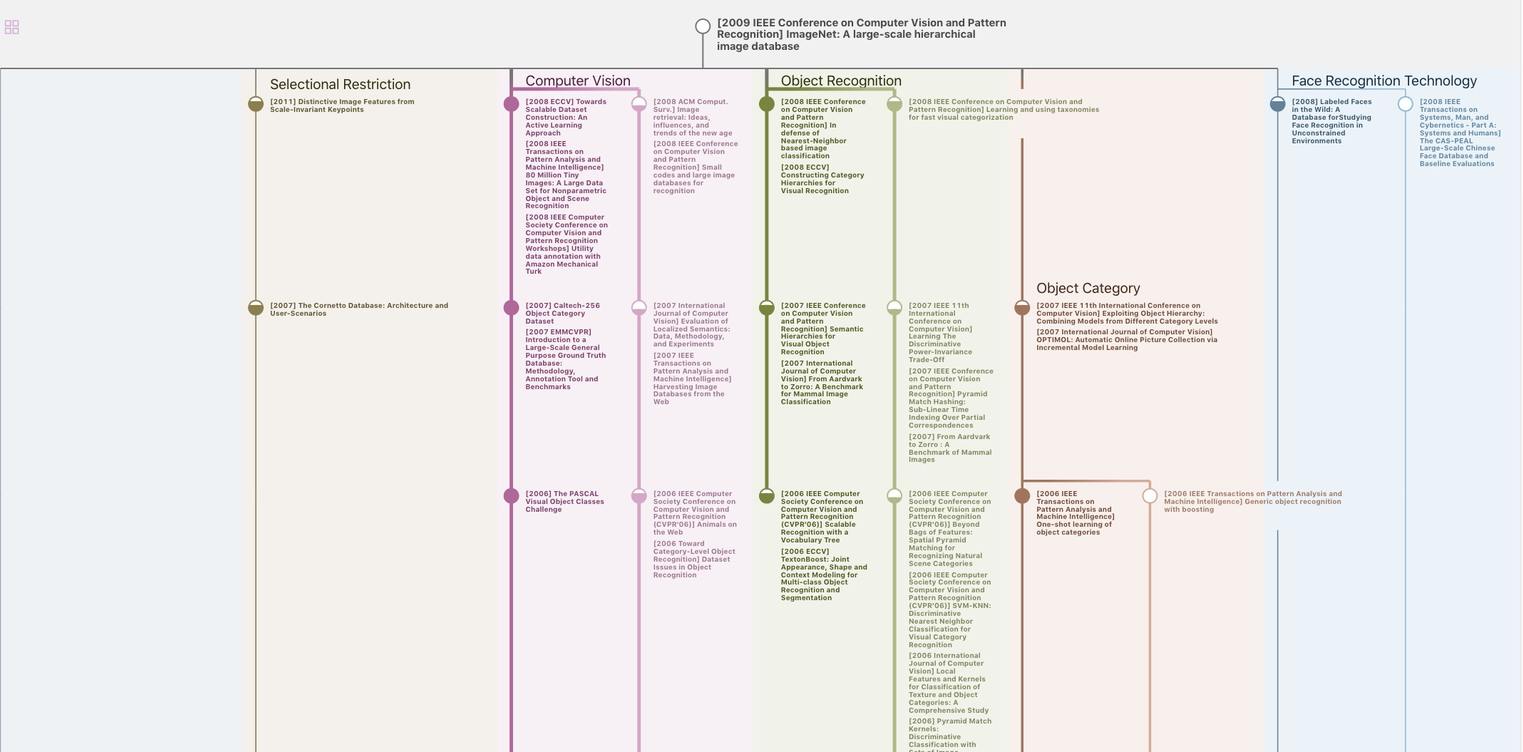
生成溯源树,研究论文发展脉络
Chat Paper
正在生成论文摘要