Assessing base-resolution DNA mechanics on the genome scale
biorxiv(2023)
摘要
Intrinsic DNA properties including bending play a crucial role in diverse biological systems. A recent advance in a high-throughput technology called loop-seq makes it possible to determine the bendability of hundred thousand 50-bp DNA duplexes in one experiment. However, it's still challenging to assess base-resolution sequence bendability in large genomes such as human, which requires thousands of such experiments. Here, we introduce 'BendNet'-a deep neural network to predict the intrinsic DNA bending at base-resolution by using loop-seq results in yeast as training data. BendNet can predict the DNA bendability of any given sequence from different species with high accuracy. To explore the utility of BendNet, we applied it to the human genome and observed DNA bendability is associated with chromatin features and disease risk regions involving transcription/enhancer regulation, DNA replication, transcription factor binding and extrachromosomal circular DNA generation. These findings expand our understanding on DNA mechanics and its association with transcription regulation in mammals. Lastly, we built a comprehensive resource of genomic DNA bendability profiles for 307 species by applying BendNet, and provided an online tool to assess the bendability of user-specified DNA sequences (http://www.dnabendnet.com/). Graphical Abstract
更多查看译文
关键词
genome,dna,scale,base-resolution
AI 理解论文
溯源树
样例
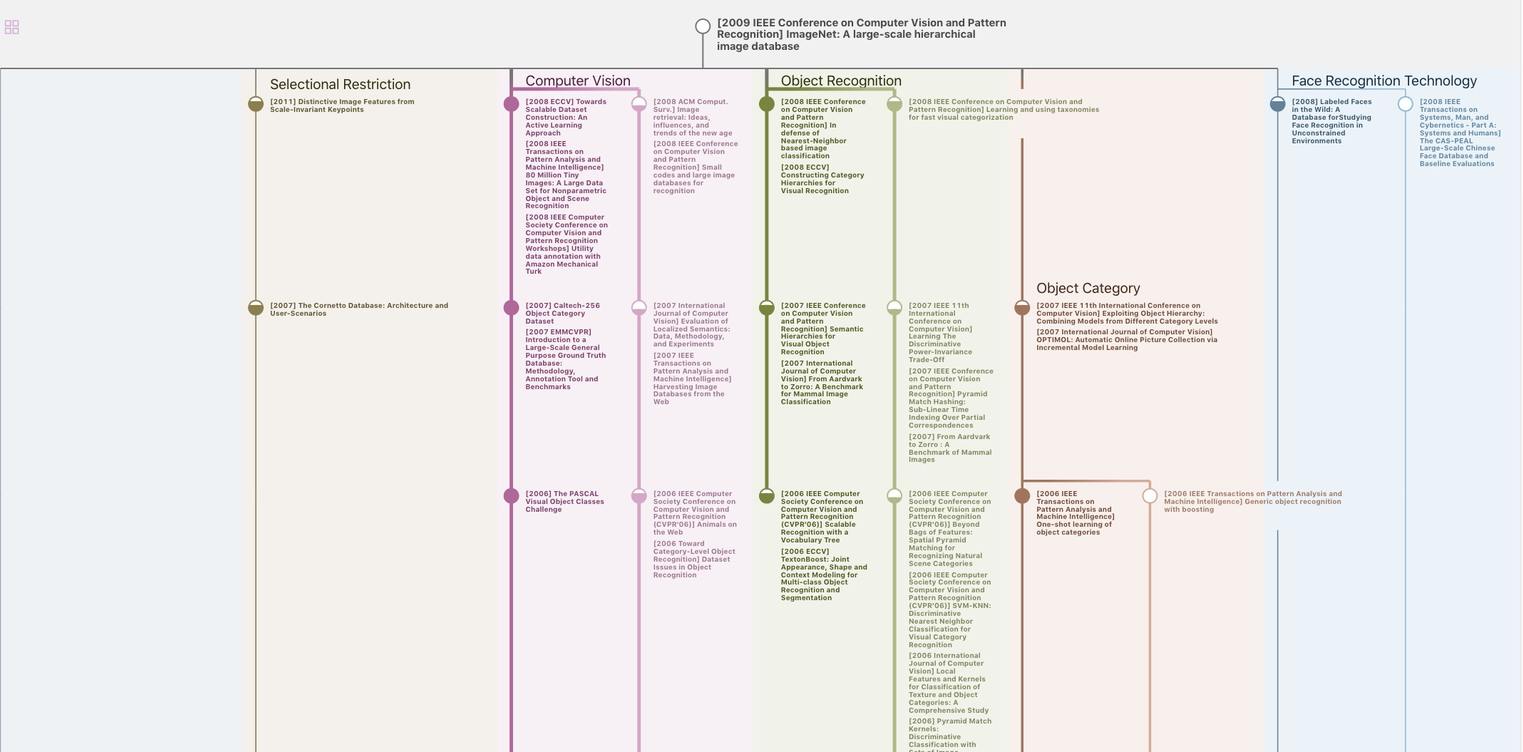
生成溯源树,研究论文发展脉络
Chat Paper
正在生成论文摘要