Maps, trends, and temperature sensitivities—phenological information from and for decreasing numbers of volunteer observers
International Journal of Biometeorology(2021)
摘要
Phenology serves as a major indicator of ongoing climate change. Long-term phenological observations are critically important for tracking and communicating these changes. The phenological observation network across Germany is operated by the National Meteorological Service with a major contribution from volunteering activities. However, the number of observers has strongly decreased for the last decades, possibly resulting in increasing uncertainties when extracting reliable phenological information from map interpolation. We studied uncertainties in interpolated maps from decreasing phenological records, by comparing long-term trends based on grid-based interpolated and station-wise observed time series, as well as their correlations with temperature. Interpolated maps in spring were characterized by the largest spatial variabilities across Bavaria, Germany, with respective lowest interpolated uncertainties. Long-term phenological trends for both interpolations and observations exhibited mean advances of −0.2 to −0.3 days year −1 for spring and summer, while late autumn and winter showed a delay of around 0.1 days year −1 . Throughout the year, temperature sensitivities were consistently stronger for interpolated time series than observations. Such a better representation of regional phenology by interpolation was equally supported by satellite-derived phenological indices. Nevertheless, simulation of observer numbers indicated that a decline to less than 40% leads to a strong decrease in interpolation accuracy. To better understand the risk of declining phenological observations and to motivate volunteer observers, a Shiny app is proposed to visualize spatial and temporal phenological patterns across Bavaria and their links to climate change–induced temperature changes.
更多查看译文
关键词
Phenological season, Map interpolation, Multiple linear regression, Inverse distance weighting, Leave-one-out cross validation, Citizen science
AI 理解论文
溯源树
样例
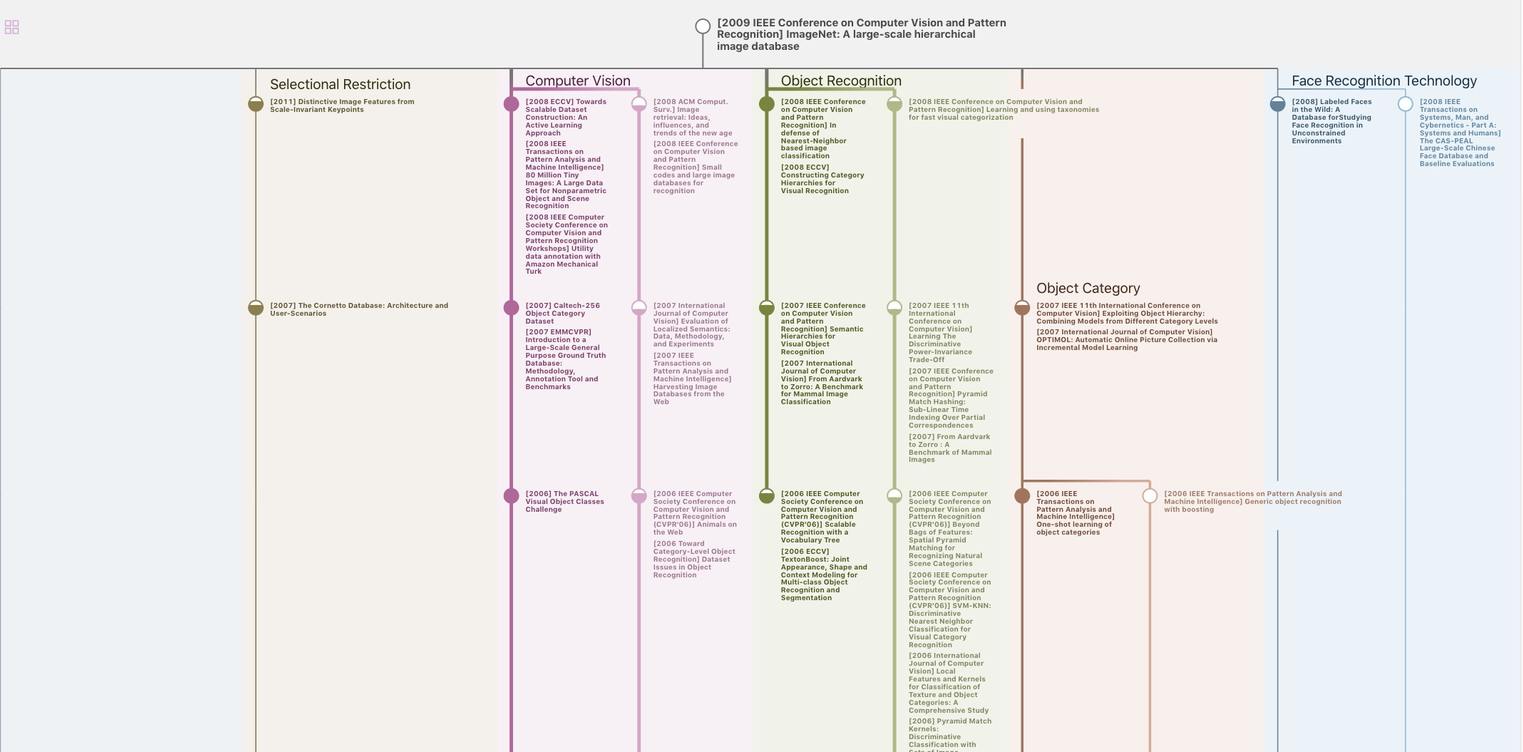
生成溯源树,研究论文发展脉络
Chat Paper
正在生成论文摘要