Prediction of TBM cutterhead speed and penetration rate for high-efficiency excavation of hard rock tunnel using CNN-LSTM model with construction big data
Arabian Journal of Geosciences(2022)
摘要
Cutterhead speed and penetration rate are two key operating parameters that hard-rock tunnel boring machine (TBM) operators control to minimize the risks associated with high capital costs and scheduling for tunnel excavation. This paper introduces a predictive model of the TBM cutterhead speed and penetration rate by using the one-dimensional convolutional neural networks and long short-term memory network (CNN-LSTM) that have outstanding ability in learning the time-sequential and multidimensional construction big data. A multi-source database including geological data of rock types and rock mass classes and 4.08 billion records of in situ TBM construction data of 199 parameters from the YinSong water division project in Jilin province was constructed to establish the CNN-LSTM model. The features for predicting the cutterhead speed and penetration rate in the CNN-LSTM model were extracted by the Pearson correlation coefficient and LightGBM method. The results show that the proposed CNN-LSTM model can predict the TBM cutterhead speed and penetration rate with high accuracy and has superior predictive performance to the CNN, LSTM, Lasso, SVM, and decision tree models. The model is useful by suggesting the TBM operators the cutterhead speed and penetration rate values in each excavation cycle during hard rock tunneling in different construction conditions.
更多查看译文
关键词
Hard rock tunneling, Artificial intelligence, Construction big data, Tunnel boring machine, Convolutional neural network, Long short-term memory
AI 理解论文
溯源树
样例
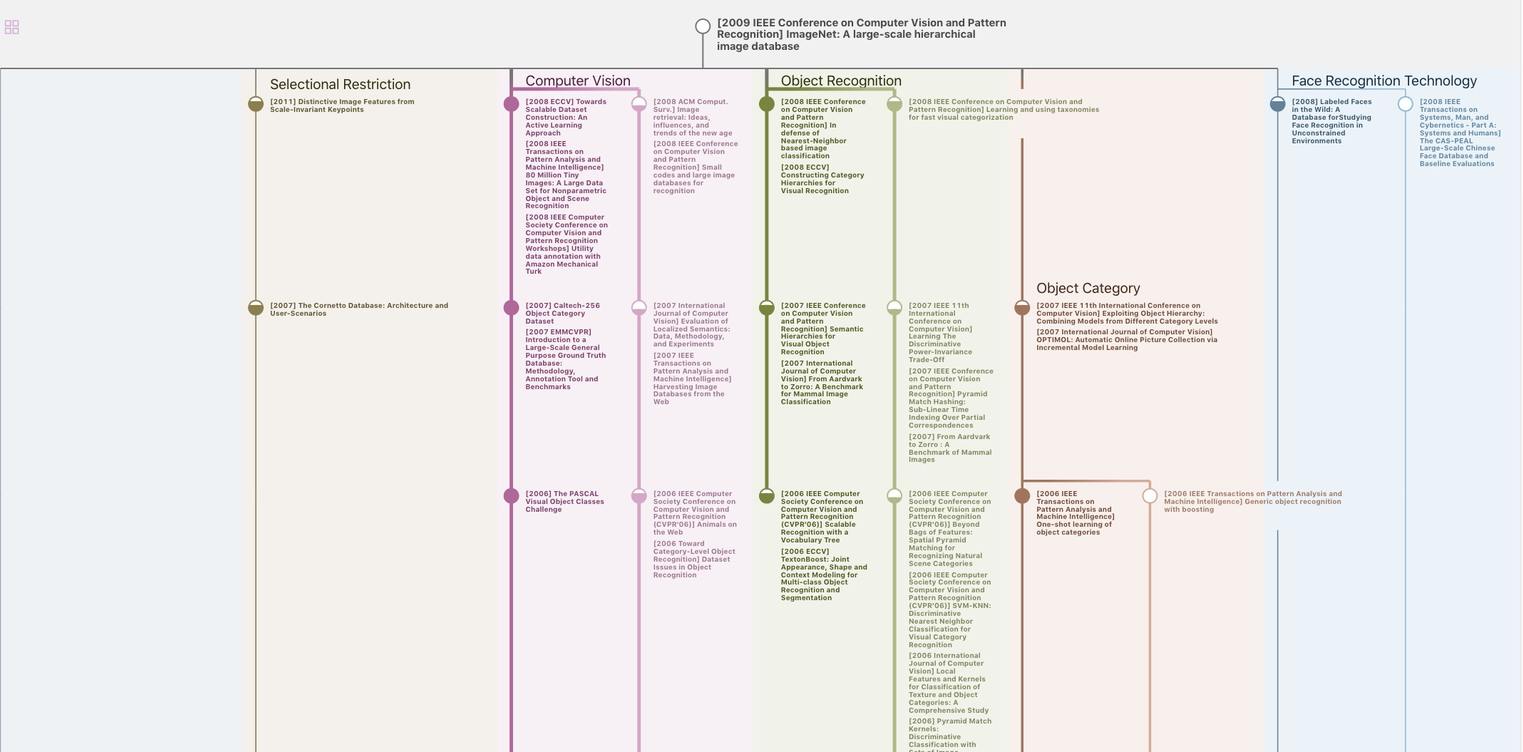
生成溯源树,研究论文发展脉络
Chat Paper
正在生成论文摘要