Impact of Data Cleansing for Urban Bus Commercial Speed Prediction
SN Computer Science(2021)
摘要
Public Transportation Information Systems (PTIS) are widely used for public bus services amongst cities in the world. These systems gather information about trips, bus stops, bus speeds, ridership, etc. This massive data are an inviting source of information for machine learning predictive tools. However, it most often suffers from quality deficiencies, due to multiple data sets with multiple structures, to different infrastructures using incompatible technologies, to human errors or hardware failures. In this paper, we consider the impact of data cleansing on a classical machine-learning task: predicting urban bus commercial speed. We show that simple, transport specific business and quality rules can drastically enhance data quality, whereas more sophisticated rules may offer little improvements despite a high computational cost.
更多查看译文
关键词
Prediction,Machine learning,Data cleansing,Public transportation
AI 理解论文
溯源树
样例
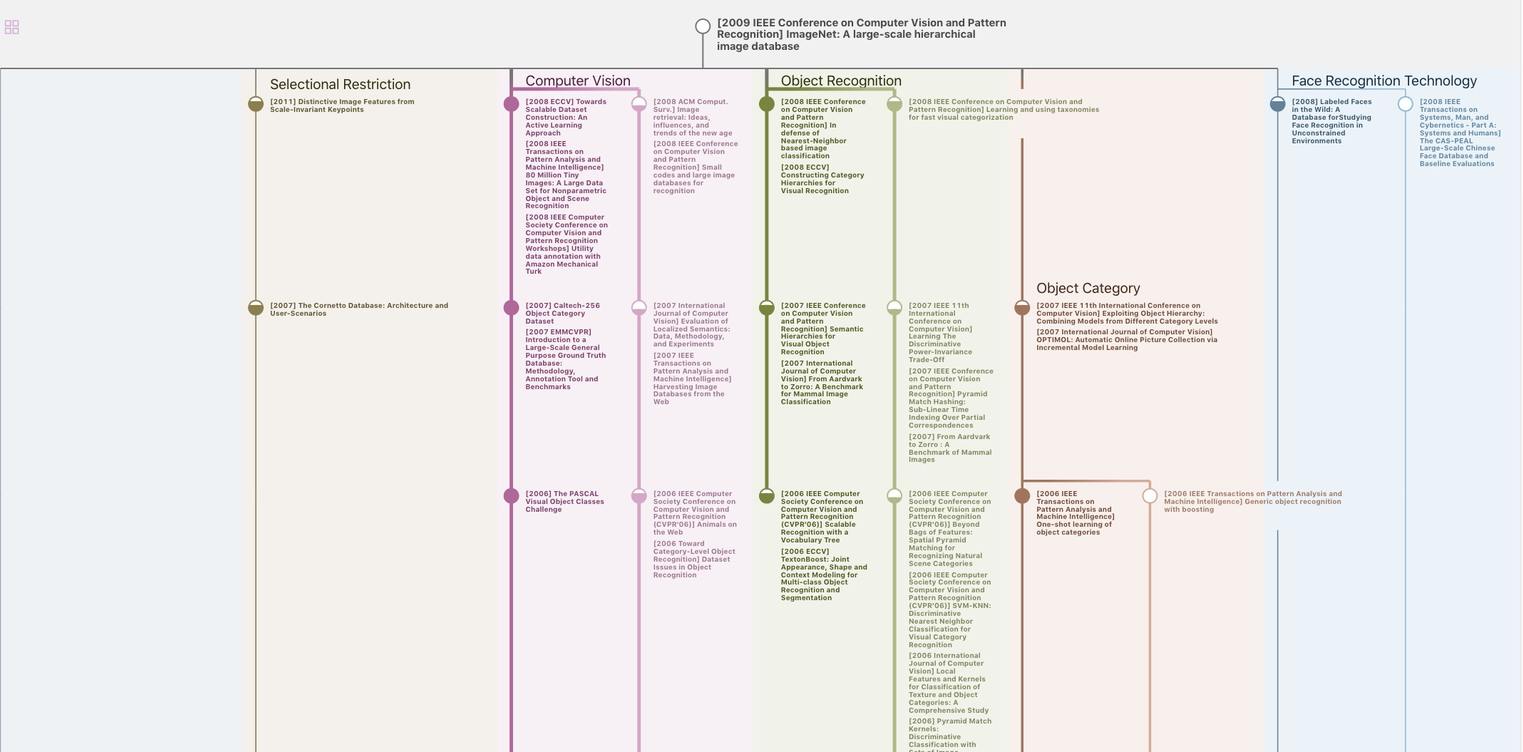
生成溯源树,研究论文发展脉络
Chat Paper
正在生成论文摘要