Design of Extreme Learning Machine with Smoothed ℓ 0 Regularization
Mobile Networks and Applications(2020)
摘要
In extreme learning machine (ELM), a large number of hidden nodes are required due to the randomly generated hidden layer. To improve network compactness, the ELM with smoothed ℓ 0 regularizer (ELM-SL0 for short) is studied in this paper. Firstly, the ℓ 0 regularization penalty term is introduced into the conventional error function, such that the unimportant output weights are gradually forced to zeros. Secondly, the batch gradient method and the smoothed ℓ 0 regularizer are combined for training and pruning ELM. Furthermore, both the weak convergence and strong convergence of ELM-SL0 are investigated. Compared with other existing ELMs, the proposed algorithm obtains better performance in terms of estimation accuracy and network sparsity.
更多查看译文
关键词
Extreme learning machine,Sparsity,ℓ 0 regularization,Network compactness
AI 理解论文
溯源树
样例
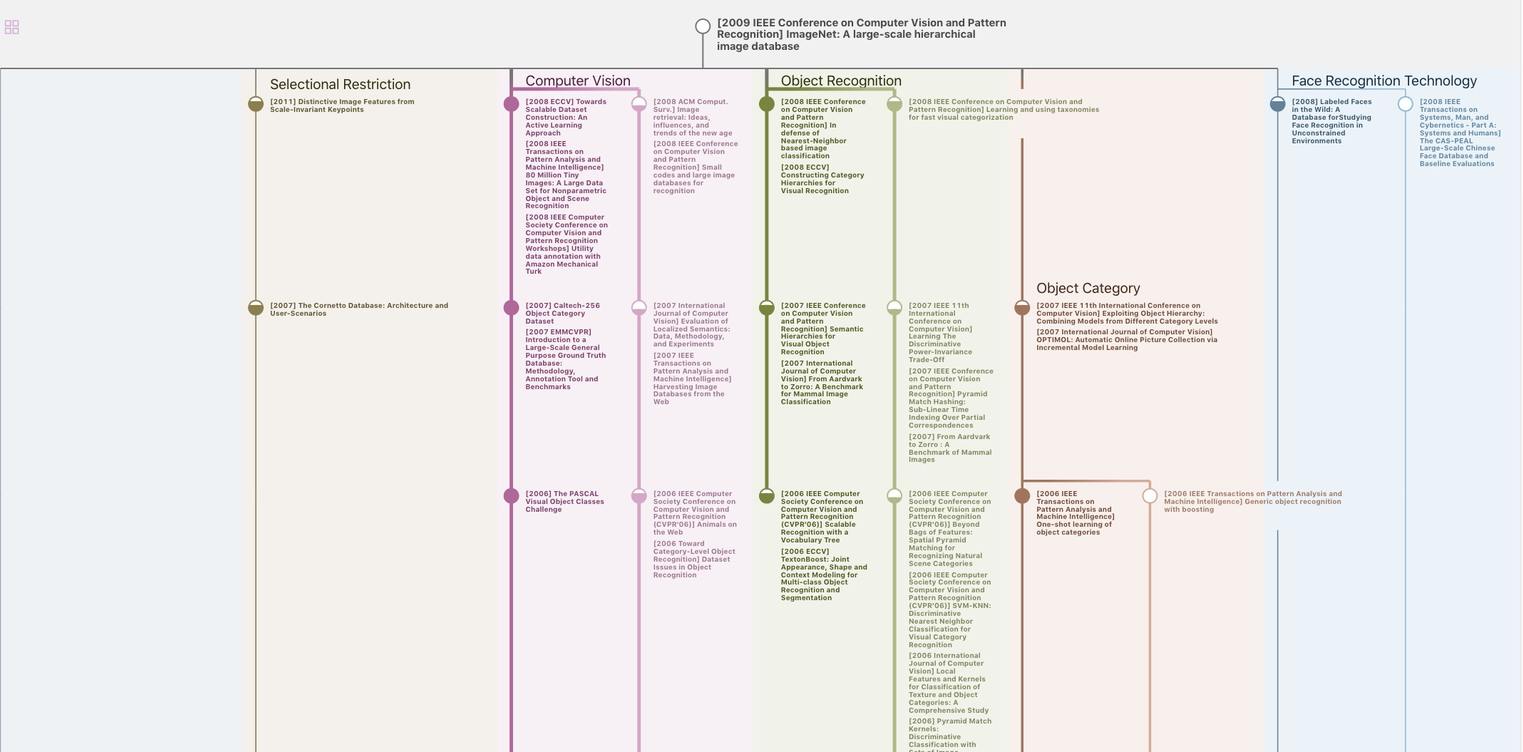
生成溯源树,研究论文发展脉络
Chat Paper
正在生成论文摘要