Defocus Blur detection via transformer encoder and edge guidance
Applied Intelligence(2022)
摘要
Defocus blur detection (DBD) aims to separate blurred and unblurred regions for a given image. Benefiting from the powerful extraction capabilities of convolutional neural networks (CNNs), deep learning based defocus blur detection has achieved a remarkable progress compared with traditional methods. However, due to the limited local receptive field of CNNs, it is difficult to achieve satisfactory results in the detection of the low-contrast focal regions. Besides, the output maps of the most of previous works have coarse object boundaries and background clutter. In this paper, we propose a hybrid CNN-Transformer architecture with an edge guidance aggregation module (EGAM) and a feature fusion module (FFM) for DBD. In our knowledge, this is the first study to utilize a transformer encoder for DBD to capture the global context information. Additionally, an edge extraction network (EENet) is adopted to obtain local edge information of in-focus objects. To effectively aggregate local edge information and global semantic features, three EGAMs are integrated into an edge guidance fusion network (EGFNet). Benefiting from the rich edge information, the fused features can generate more accurate boundaries. Finally, three FFMs are cascaded as a hierarchical feature aggregation network (HFANet) to hierarchically decode and refine the feature maps. Experimental results on three widely used DBD datasets demonstrate that the proposed model outperforms the state-of-the-art approaches.
更多查看译文
关键词
Defocus blur detection,Edge guidance aggregation,Transformer encoder,Low-contrast focal regions
AI 理解论文
溯源树
样例
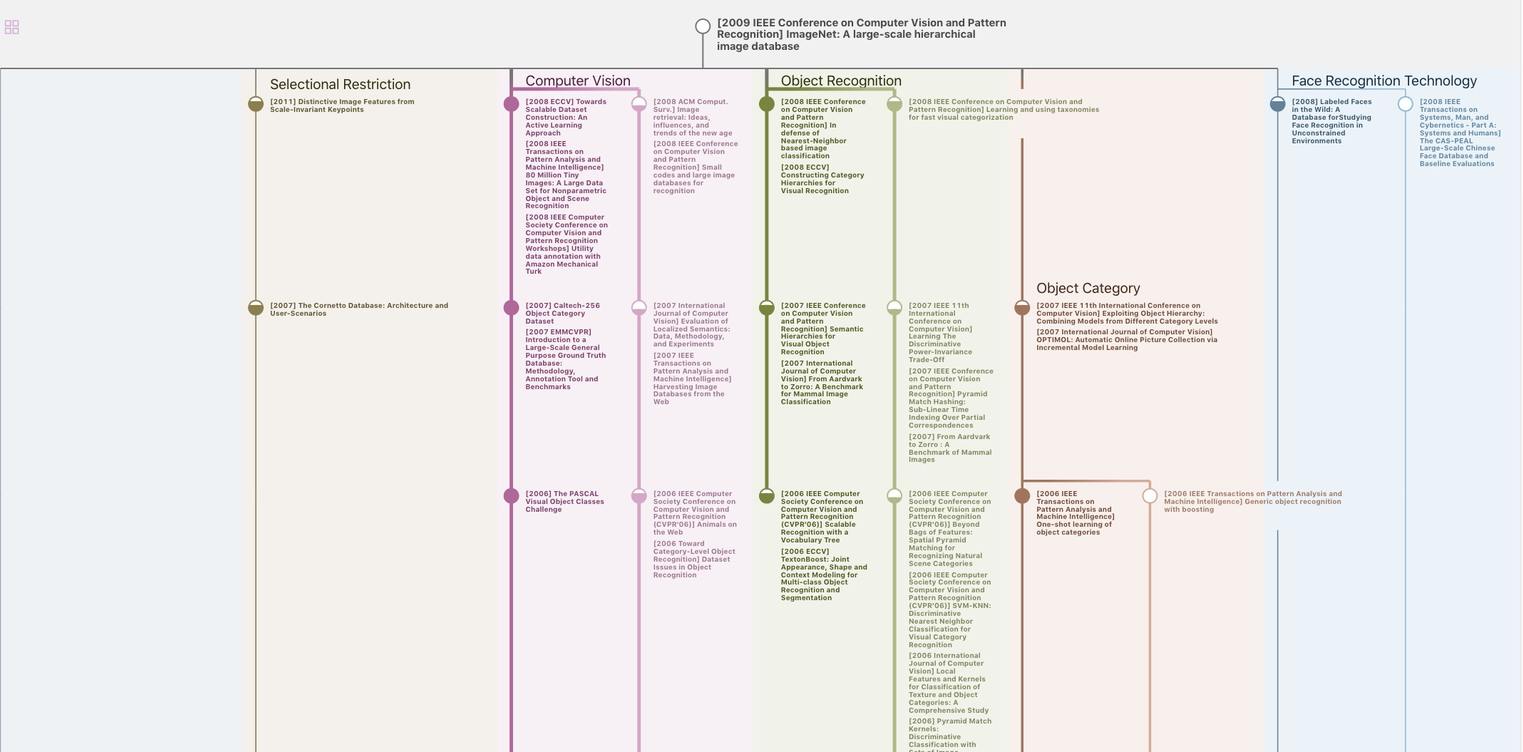
生成溯源树,研究论文发展脉络
Chat Paper
正在生成论文摘要