Applicability of Machine Learning Model to Simulate Atmospheric CO2 Variability
IEEE TRANSACTIONS ON GEOSCIENCE AND REMOTE SENSING(2022)
摘要
Carbon dioxide (CO2) is the most important greenhouse gas influencing the Earth's climate; therefore, accurate modeling of its variability has paramount significance. In this regard, we have performed the simulation of CO2 residue (i.e., detrended depersonalized CO2) based on input of meteorological parameters (temperature, humidity, pressure, and wind), El Nino index, sea surface temperature, and normalized difference vegetation index in a machine learning (ML) model. Long-term observations available from the World Data Centre for Greenhouse Gases (WDCGG) and the National Oceanic and Atmospheric Administration (NOAA) have been used for training and validation of ML model. The model successfully reproduced 72% of observed variability in CO2 residue with an error of 0.45 ppmv over Mauna Loa (19.54 degrees N; -155.58 degrees E). The cumulative temperature anomaly is found to play a key role in the simulation of CO2 residue over Mauna Loa. Evaluation reveals a reasonably good agreement between modeled and observed CO2 residue (R-2 = 0.20-0.55 and root-mean-square error (RMSE) = 20%-60%) over regional sites and for global mean CO2. However, the model shows a limitation in capturing spikes likely caused by strong local influences. Inclusion of additional input parameters, representing local anthropogenic influences, is recommended to further improve the model performance over regional sites. Our study demonstrates the potential of ML modeling for the simulations of CO2 variability to complement the computationally expensive climate models.
更多查看译文
关键词
Artificial intelligence (AI),climate change,global warming,greenhouse gases
AI 理解论文
溯源树
样例
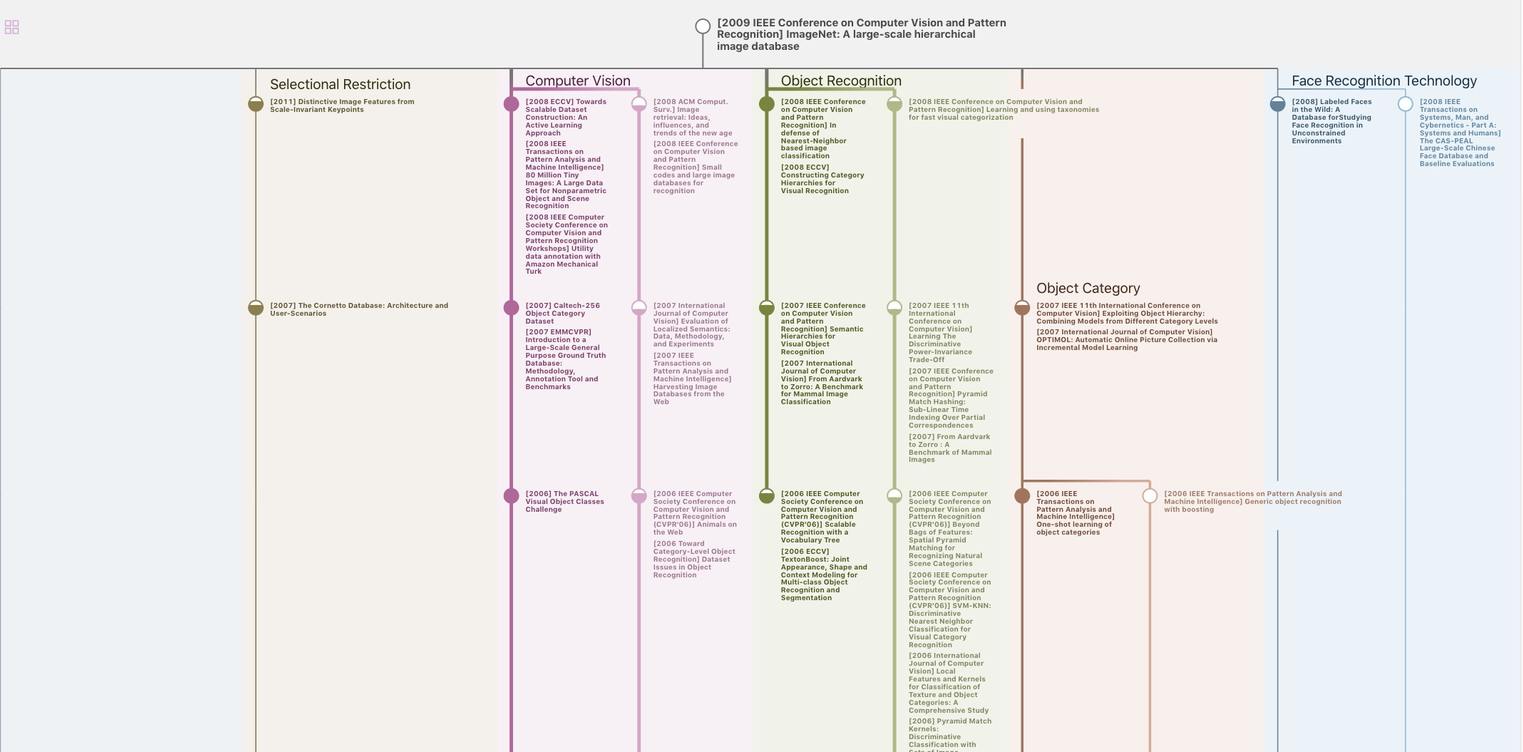
生成溯源树,研究论文发展脉络
Chat Paper
正在生成论文摘要