Face De-Occlusion With Deep Cascade Guidance Learning.
IEEE Transactions on Multimedia(2023)
摘要
Occlusion is a challenging yet commonly seen problem for facial perception. Existing works resort to deep learning models and perform model training on synthesized data due to the lack of paired real-world data. As a result,they usually perform unsatisfactorily on real-world occluded faces because of domain gaps. In this paper, we decompose the face de-occlusion task into three stages, i.e., occlusion detection, face parsing, and face reconstruction, to alleviate this issue. We first perform occlusion detection and use its results as guidance for the second stage to conduct occlusion-free face parsing. As such, face de-occlusion is first performed on the face paring space with less difficulty. We can train these two stages on both synthesized and real-world images, hence can obtain accurate results for the latter. In the last stage, we use the domain-agnostic occlusion detection map and the face parsing map as the guidance to conduct face reconstruction, thus can reduce the impact of appearance information and improve the model performance on real-world data. Aiming at improving the model capacity of inferring occluded facial appearance, we also propose two types of reference modules to use relevant facial parts to enhance the reconstruction of occluded regions. Consequently, our proposed model achieves promising face de-occlusion results on real-world images.
更多查看译文
关键词
Face de-occlusion, face inpainting, face parsing, GAN
AI 理解论文
溯源树
样例
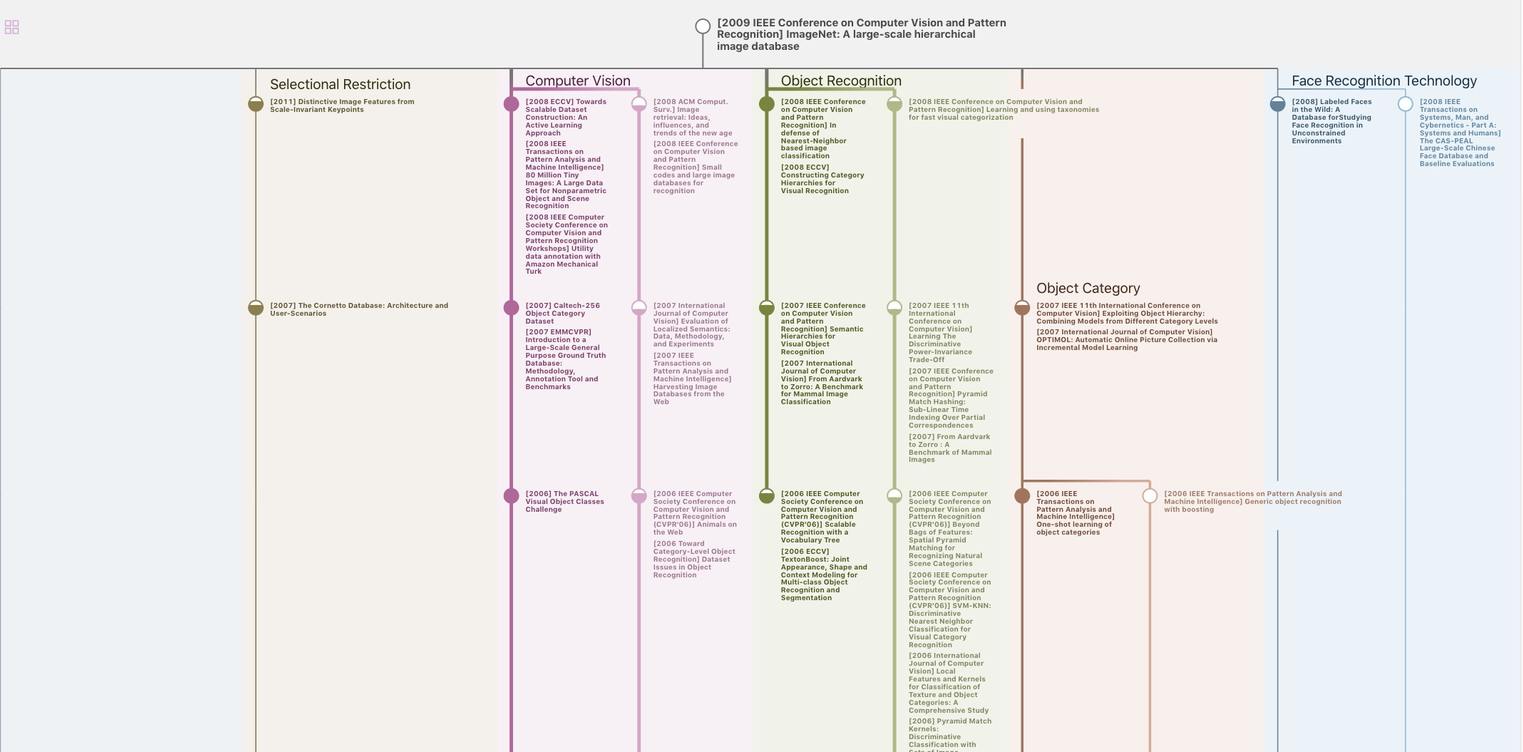
生成溯源树,研究论文发展脉络
Chat Paper
正在生成论文摘要