Data augmentation for speech separation
SSRN Electronic Journal(2023)
摘要
Deep learning models have advanced the state of the art of monaural speech separation. However, the performance of a separation model considerably decreases when tested on unseen speakers and noisy conditions. Separation models trained with data augmentation generalize better to unseen conditions. In this paper, we conduct a comprehensive survey of data augmentation techniques and apply them to improve the generalization of time-domain speech separation models. The augmentation techniques include seven source preserving approaches (Gaussian noise, Gain, Time masking, frequency masking, Short noise, Time stretch, and Pitch shift) and three non-source preserving approaches (Dynamix mixing, Mixup, and Cutmix). After hyperparameter search for each augmentation method, we test the generalization of the augmented model by cross-corpus testing on three datasets (LibriMix, TIMIT, and VCTK), and identify the best augmentation combination that enhances generalization. Experimental results indicate that a combination of several non source preserving strategies (CutMix, Mixup, and Dynamic mixing) resulted in the best generalization performance. Finally, the augmentation combinations also improved the performance of the speech separation model even when fewer training data are available.
更多查看译文
关键词
Data augmentation,Deep learning,Domain generalization,Speech separation
AI 理解论文
溯源树
样例
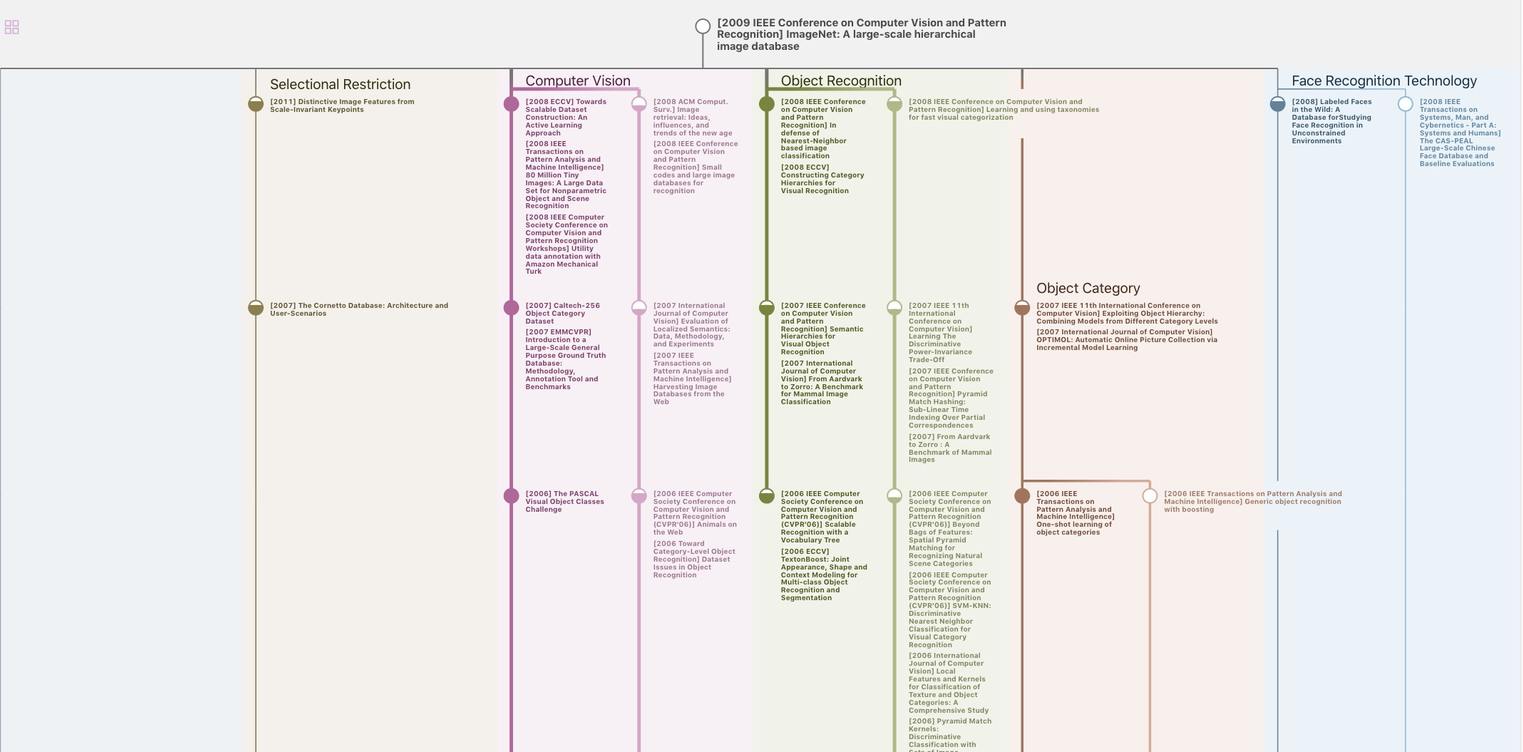
生成溯源树,研究论文发展脉络
Chat Paper
正在生成论文摘要