A Flexible Deartifacting Module for Compressed Sensing MRI
IEEE Journal of Selected Topics in Signal Processing(2022)
摘要
Compressed sensing (CS) has been a novel technique for fast reconstruction of magnetic resonance (MR) images from their partial
$k$
-space measurements. However, the quality of the reconstructed images could be severely affected by artifacts from various sources. In this paper, we propose a deartifacting module (DEMO) that can effectively remove the artifacts by eliminating sparse outliers in the
$k$
-space. Specifically, DEMO augments the measurements in the original loss function to approximate a new loss that is robust to outliers. Since DEMO is developed independently of any backbone algorithm to perform with, it can be flexibly incorporated into a broad range of CS-MRI methods, including both model-based methods and unrolling deep neural networks. Extensive experiments under various settings demonstrate the effectiveness and robustness of DEMO.
更多查看译文
关键词
Artifacts removal,compressed sensing (CS),high-dimensional sensing (HDS),magnetic resonance imaging (MRI),outliers,unrolling neural networks
AI 理解论文
溯源树
样例
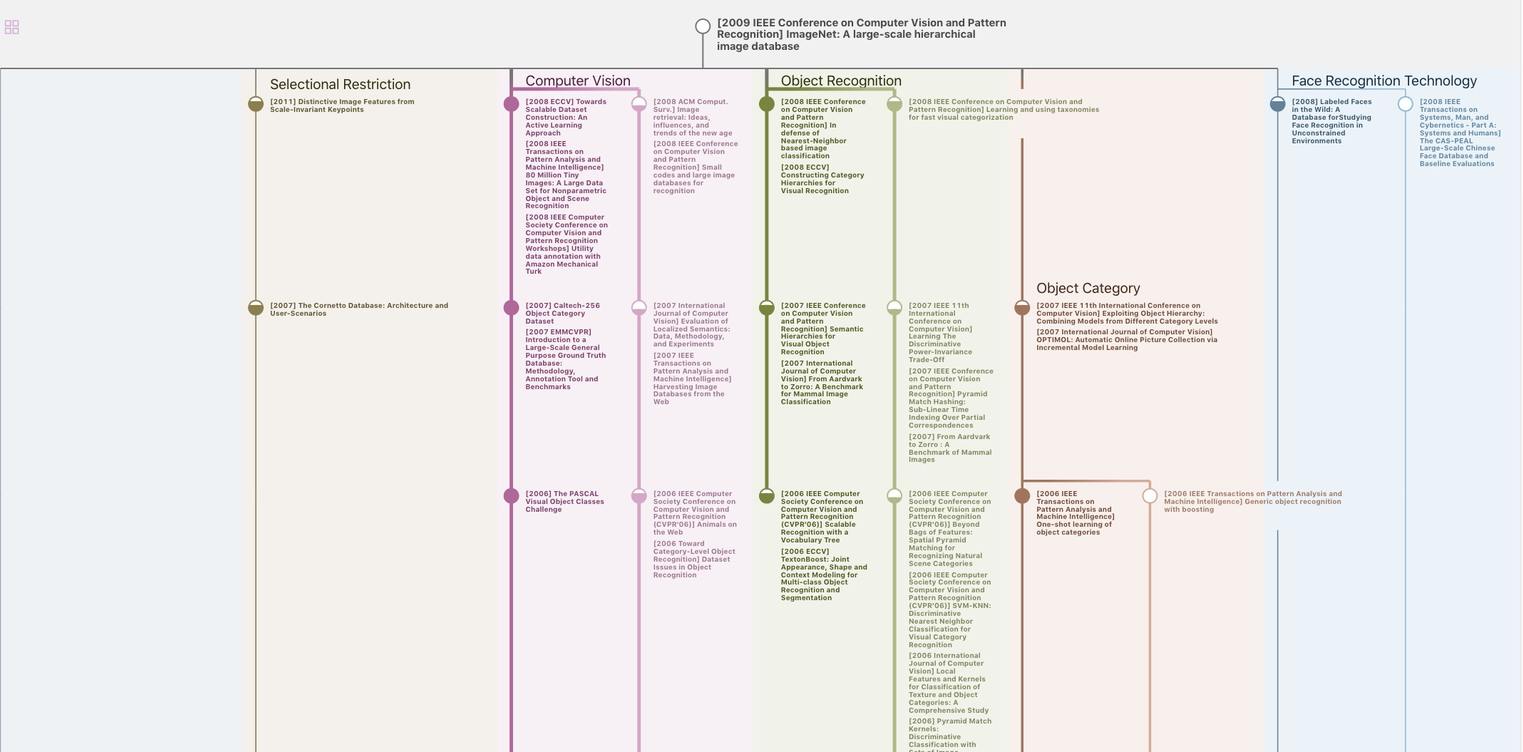
生成溯源树,研究论文发展脉络
Chat Paper
正在生成论文摘要