Type-2 Fuzzy Model-Based Movement Primitives for Imitation Learning
IEEE Transactions on Robotics(2022)
摘要
Imitation learning is an important direction in the area of robot skill learning. It provides a user-friendly and straightforward solution to transfer human demonstrations to robots. In this article, we integrate fuzzy theory into imitation learning to develop a novel method called type-2 fuzzy model-based movement primitives (T2FMP). In this method, a group of data-driven type-2 fuzzy models are used to describe the input–output relationships of demonstrations. Based on the fuzzy models, T2FMP can efficiently reproduce the trajectory without high computational costs or cumbersome parameter settings. Besides, it can well handle the variation of the demonstrations and is robust to noise. In addition, we develop extensions that endow T2FMP with trajectory modulation and superposition to achieve real-time trajectory adaptation to various scenarios. Going beyond existing imitation learning methods, we further extend T2FMP to regulate the trajectory to avoid collisions in the environment that is unstructured, nonconvex, and detected with noisy outliers. Several experiments are performed to validate the effectiveness of our method.
更多查看译文
关键词
Collision avoidance,imitation learning,movement primitives,point cloud,type-2 fuzzy model
AI 理解论文
溯源树
样例
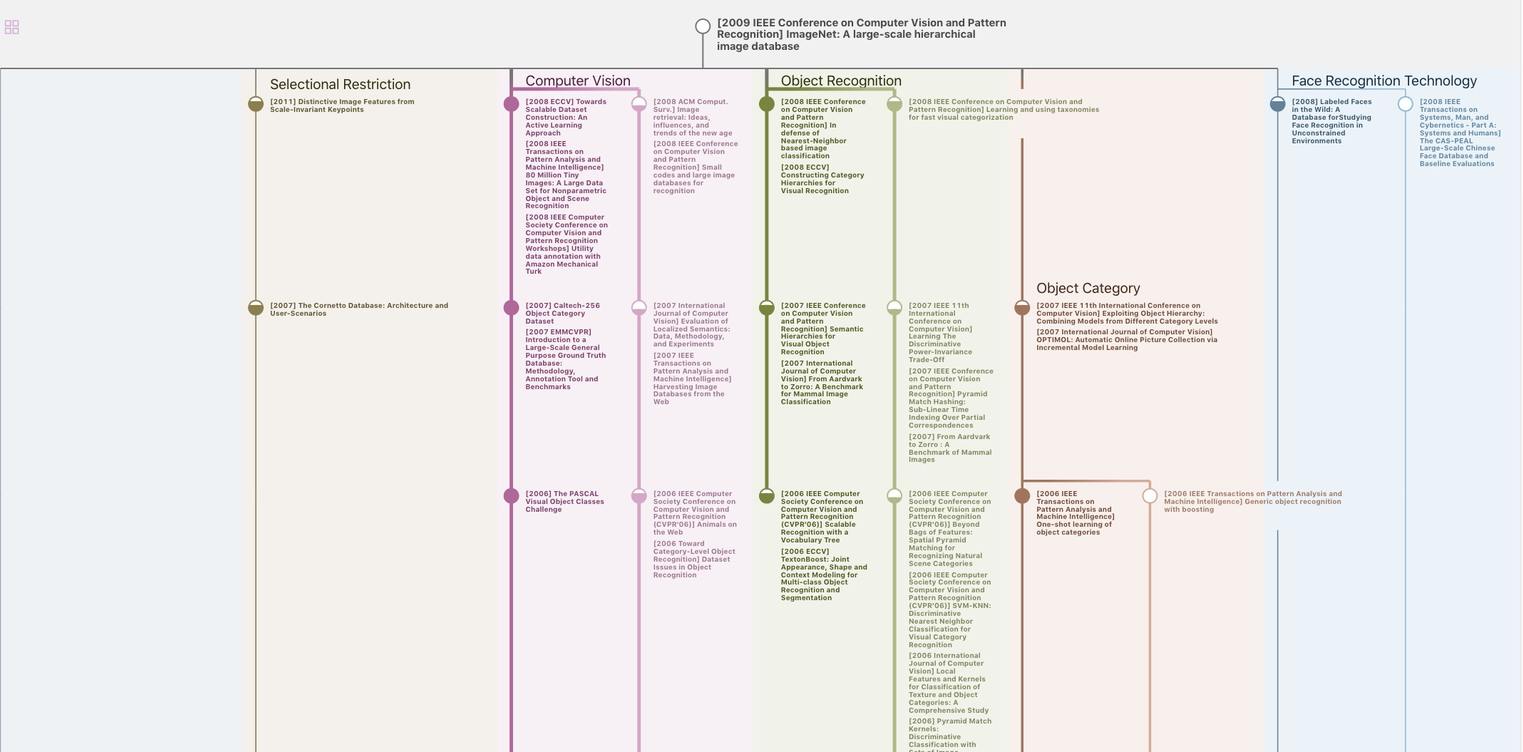
生成溯源树,研究论文发展脉络
Chat Paper
正在生成论文摘要