Scalar and Matrix Chernoff Bounds from ℓ∞-Independence
Proceedings of the 2022 Annual ACM-SIAM Symposium on Discrete Algorithms (SODA)(2022)
摘要
Previous chapter Next chapter Full AccessProceedings Proceedings of the 2022 Annual ACM-SIAM Symposium on Discrete Algorithms (SODA)Scalar and Matrix Chernoff Bounds from ℓ∞-IndependenceTali Kaufman, Rasmus Kyng, and Federico SoldáTali Kaufman, Rasmus Kyng, and Federico Soldápp.3732 - 3753Chapter DOI:https://doi.org/10.1137/1.9781611977073.147PDFBibTexSections ToolsAdd to favoritesExport CitationTrack CitationsEmail SectionsAboutAbstract We present new scalar and matrix Chernoff-style concentration bounds for a broad class of probability distributions over the binary hypercube {0, 1}n. Motivated by recent tools developed for the study of mixing times of Markov chains on discrete distributions, we say that a distribution is ℓ∞-independent when the infinity norm of its influence matrix is bounded by a constant. We show that any distribution which is ℓ∞-infinity independent satisfies a matrix Chernoff bound that matches the matrix Chernoff bound for independent random variables due to Tropp. Our matrix Chernoff bound is a broad generalization and strengthening of the matrix Chernoff bound of Kyng and Song (FOCS'18). Using our bound, we can conclude as a corollary that a union of O(log |V|) random spanning trees gives a spectral graph sparsifier of a graph with |V| vertices with high probability matching results for independent edge sampling, and matching lower bounds from Kyng and Song. Previous chapter Next chapter RelatedDetails Published:2022eISBN:978-1-61197-707-3 https://doi.org/10.1137/1.9781611977073Book Series Name:ProceedingsBook Code:PRDA22Book Pages:xvii + 3771
更多查看译文
关键词
matrix chernoff bounds
AI 理解论文
溯源树
样例
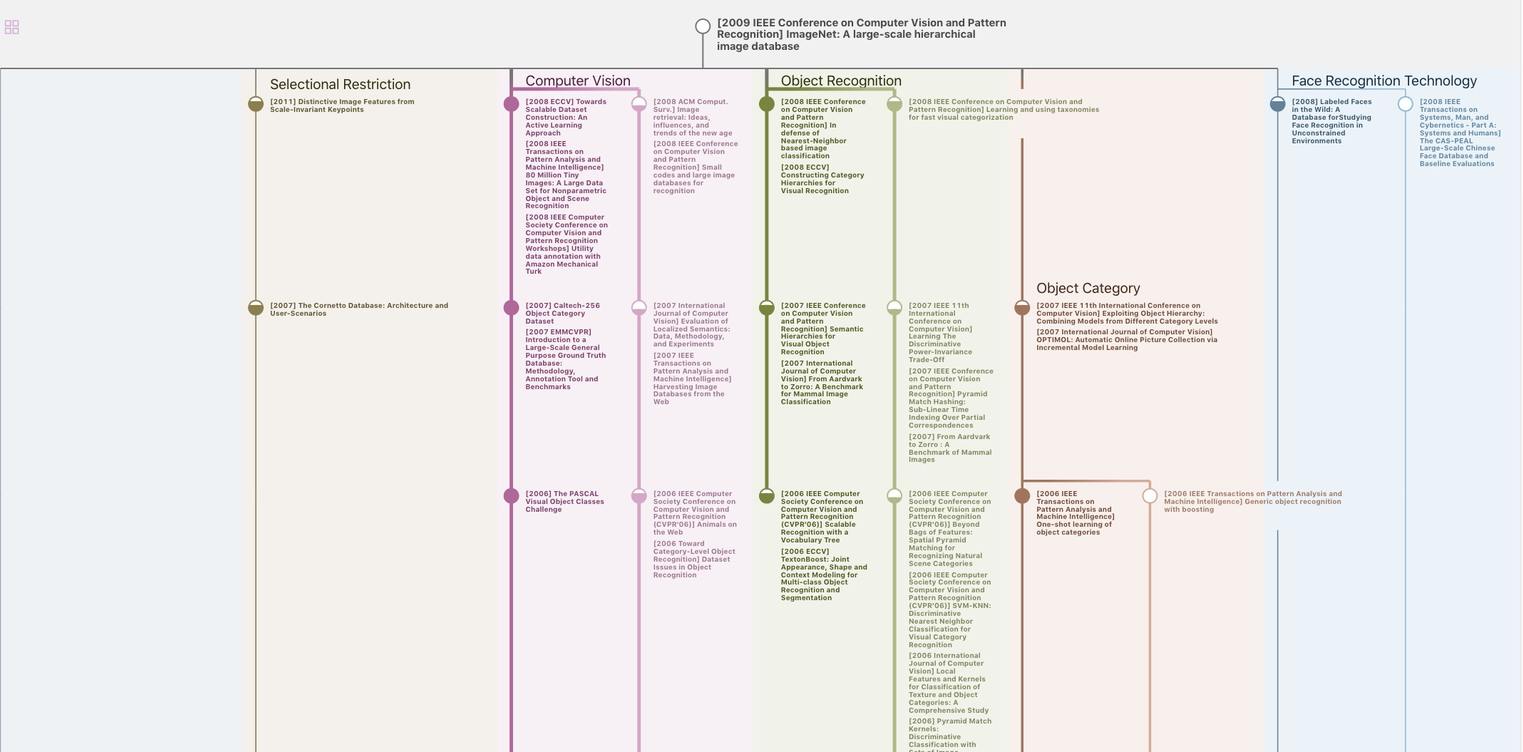
生成溯源树,研究论文发展脉络
Chat Paper
正在生成论文摘要