Deep transfer learning of additive manufacturing mechanisms across materials in metal-based laser powder bed fusion process
Journal of Materials Processing Technology(2022)
摘要
The defective regimes in metal-based Laser Powder Bed Fusion (LPBF) processes can be minimized by deploying in-situ monitoring strategies comprising Machine learning (ML) algorithms and sensing techniques. So far, algorithms trained for monitoring a particular material type cannot be re-used to monitor another material in Additive Manufacturing (AM). This is a topic rarely researched in AM. Inspired by the idea of transfer learning in ML, we demonstrate the knowledge learned by the two native Deep Learning (DL) networks, namely VGG and ResNets, on four LPBF process mechanisms such as balling, Lack of Fusion (LoF) pores, conduction mode, and keyhole pores in stainless steel (316L) can be transferred to bronze (CuSn8). In this work, the spectrograms computed using Wavelet Transforms (WT) on Acoustic Emissions (AE) during the LBPF process of stainless steel and bronze are used for training the two DL networks. Either network is first trained for classification by spectrograms representing four mechanisms during the processing of stainless steel. The trained model is then re-trained using transfer learning with spectrograms from bronze data for a similar classification task. The accuracy of the two networks during transfer learning shows that it is effectively possible to learn transferable features from one material to another with minimum network training time and dataset collection.
更多查看译文
关键词
Powder bed fusion,In-situ monitoring,Wavelet Transform,Convolutional neural network,Transfer learning
AI 理解论文
溯源树
样例
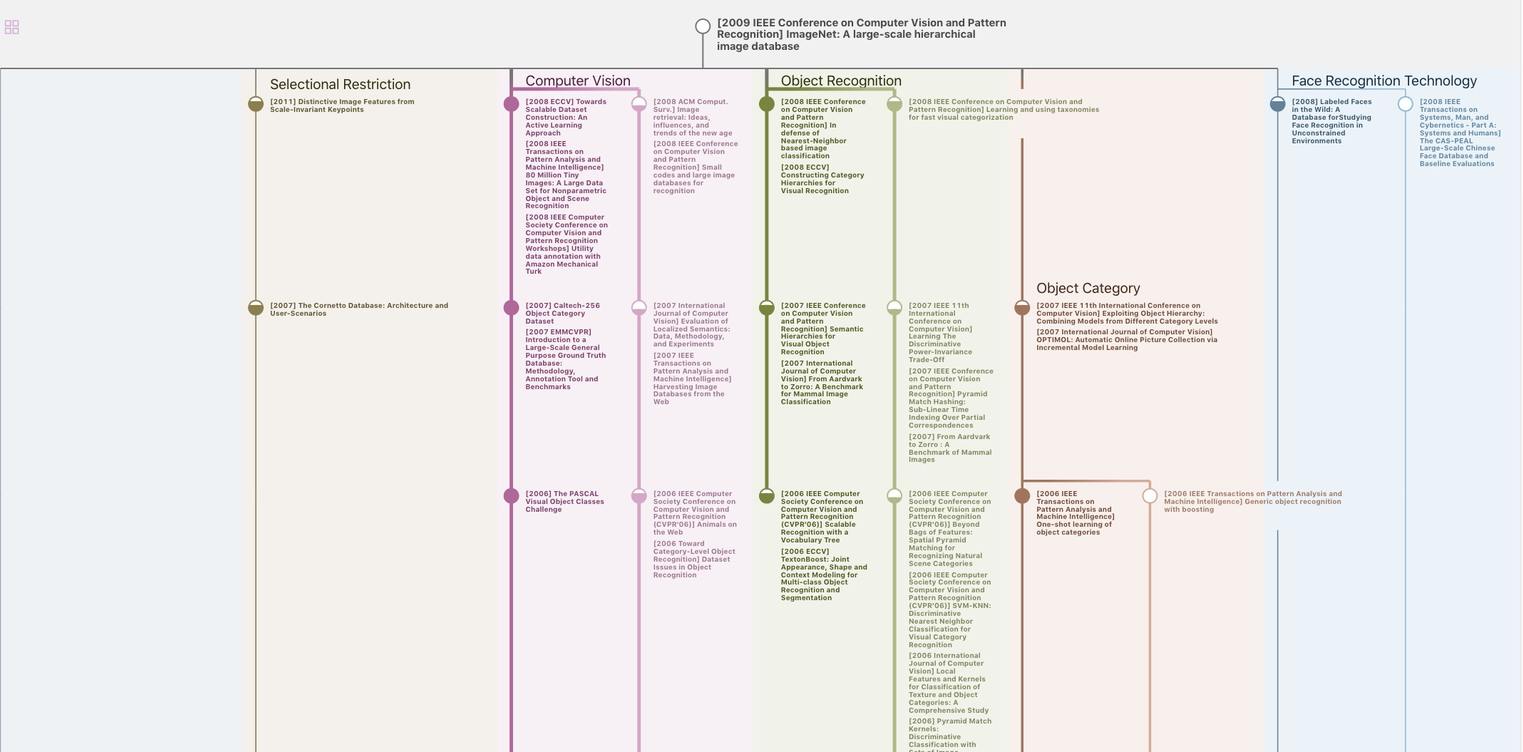
生成溯源树,研究论文发展脉络
Chat Paper
正在生成论文摘要