Air-Ground Multi-Source Image Matching Based on High-Precision Reference Image
REMOTE SENSING(2022)
摘要
Robustness of aerial-ground multi-source image matching is closely related to the quality of the ground reference image. To explore the influence of reference images on the performance of air-ground multi-source image matching, we focused on the impact of the control point projection accuracy and tie point accuracy on bundle adjustment results for generating digital orthophoto images by using the Structure from Motion algorithm and Monte Carlo analysis. Additionally, we developed a method to learn local deep features in natural environments based on fine-tuning the pre-trained ResNet50 model and used the method to match multi-scale, multi-seasonal, and multi-viewpoint air-ground multi-source images. The results show that the proposed method could yield a relatively even distribution of feature corresponding points under different conditions, seasons, viewpoints, illuminations. Compared with state-of-the-art hand-crafted computer vision and deep learning matching methods, the proposed method demonstrated more efficient and robust matching performance that could be applied to a variety of unmanned aerial vehicle self- and target-positioning applications in GPS-denied areas.
更多查看译文
关键词
bundle adjustment,Monte Carlo analysis,digital orthophoto image,ResNet50 model,image matching,GPS-denied
AI 理解论文
溯源树
样例
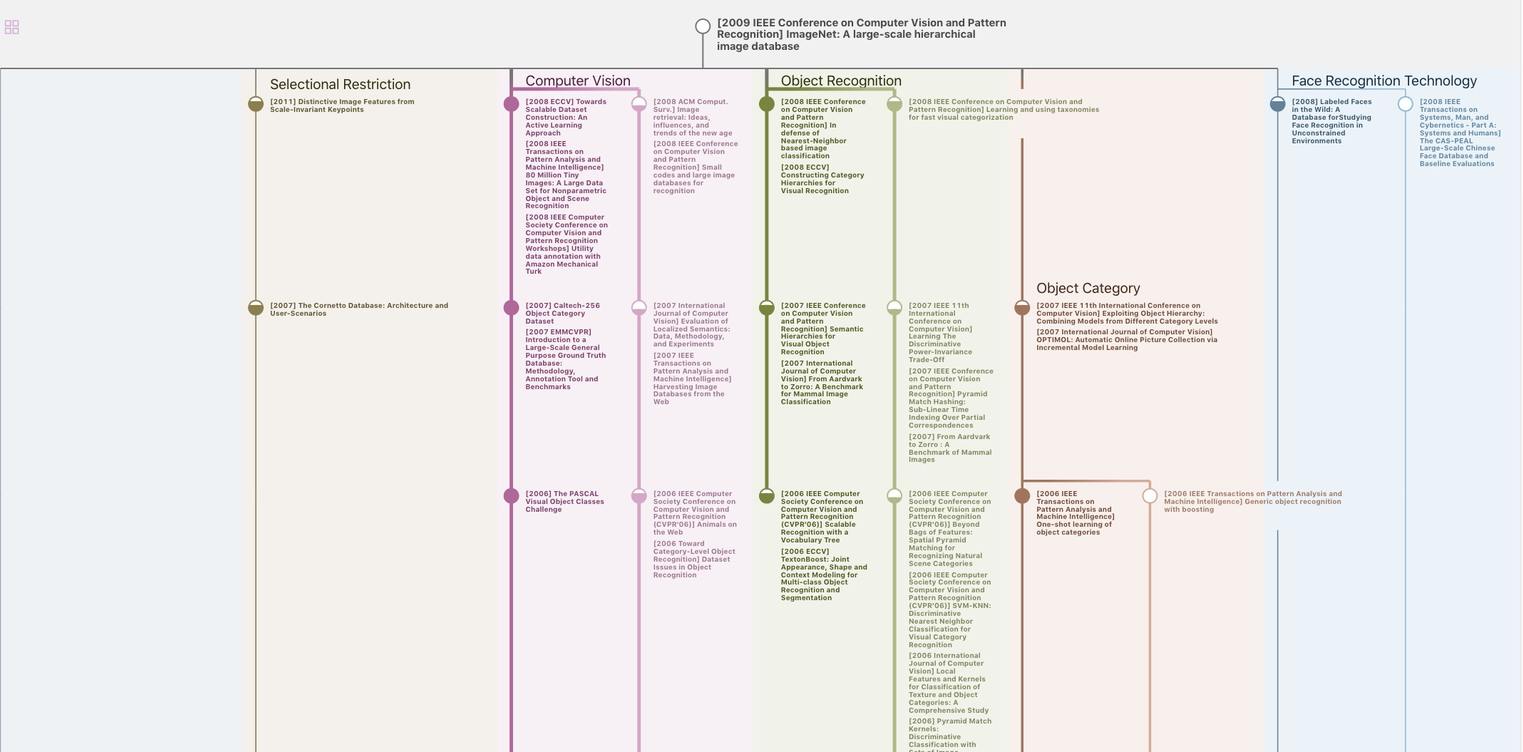
生成溯源树,研究论文发展脉络
Chat Paper
正在生成论文摘要