DEVELOPMENT OF A NOVEL ULCERATIVE COLITIS (UC) ENDOSCOPIC ACTIVITY PREDICTION MODEL USING MACHINE LEARNING (ML)
Journal of Crohn's and Colitis(2022)
摘要
Abstract Background Previous studies have described machine learning (ML) models to predict how human readers would score disease activity in UC using the endoscopic Mayo Score (eMS). So far, none employed deep human annotation that considers all the endoscopic features making up the eMS. Here we report the results of an ML model that is trained on eMS features using centrally read endoscopies. Methods 793 full-length videos were obtained from 249 patients with UC who participated in NCT02589665, a phase 2 trial with mirikizumab in patients with UC and associated with centrally read (single reader) eMS (CReMS) as the primary dataset. After cleaning for usable frames, the data were split into training, validation and testing subsets. The ML workflow consisted of annotation, segmentation, and classification (e.g., erosions, ulcers, erythema, vascular pattern, and bleeding). Human image classification and segmentation with bounding boxes and was subjected to quality control adjudicated by one of three IBD specialists, generating more than 60,000 eMS-relevant annotation labels. The model was evaluated on a test set of 147 videos using the CReMS, and a consensus set of 94 test videos, where CReMS and annotator reported eMS (AReMS) were in agreement without adjudication. The primary objective of the model was a categorical prediction of endoscopically inactive disease (eMS 0 & 1) compared with active disease (eMS 2 & 3). The secondary objectives of the model were to predict endoscopic healing (eMS 0) and to predict severe disease (eMS 3). Results The model performances are in Table 1. On the full test set of 147 videos, the model predicted inactive disease compared with active disease with an accuracy of 84%, positive predictive value (PPV) of 80%, and negative predictive value (NPV) of 85%. In the subset of 94 videos with CreMS and AReMS consensus, the model predicted inactive disease compared with active disease with an accuracy of 89%, PPV 87%, and NPV of 90%. In this same subset, the model predicted endoscopic healing and severe disease with an accuracy of 95% and 85%, PPVs of 86% and 82% and NPVs of 95% and 87%, respectively. For the secondary objectives in the full set of 147 videos, the model predicted endoscopic healing and severe disease with an accuracy of 90% and 80%, PPVs of 44% and 86%, and NPVs of 95% and 86%, respectively. Conclusion We have developed a ML predictive model of the eMS in UC using centrally read videos and demonstrate excellent distinction between active and inactive disease, and clear discrimination between other levels of endoscopic activity. We propose that this unique ML approach to endoscopic assessment be considered as a substitute to human central reading in future clinical trials.
更多查看译文
关键词
endoscopic activity prediction model,ulcerative colitis,novel ulcerative colitis,machine learning,prediction model
AI 理解论文
溯源树
样例
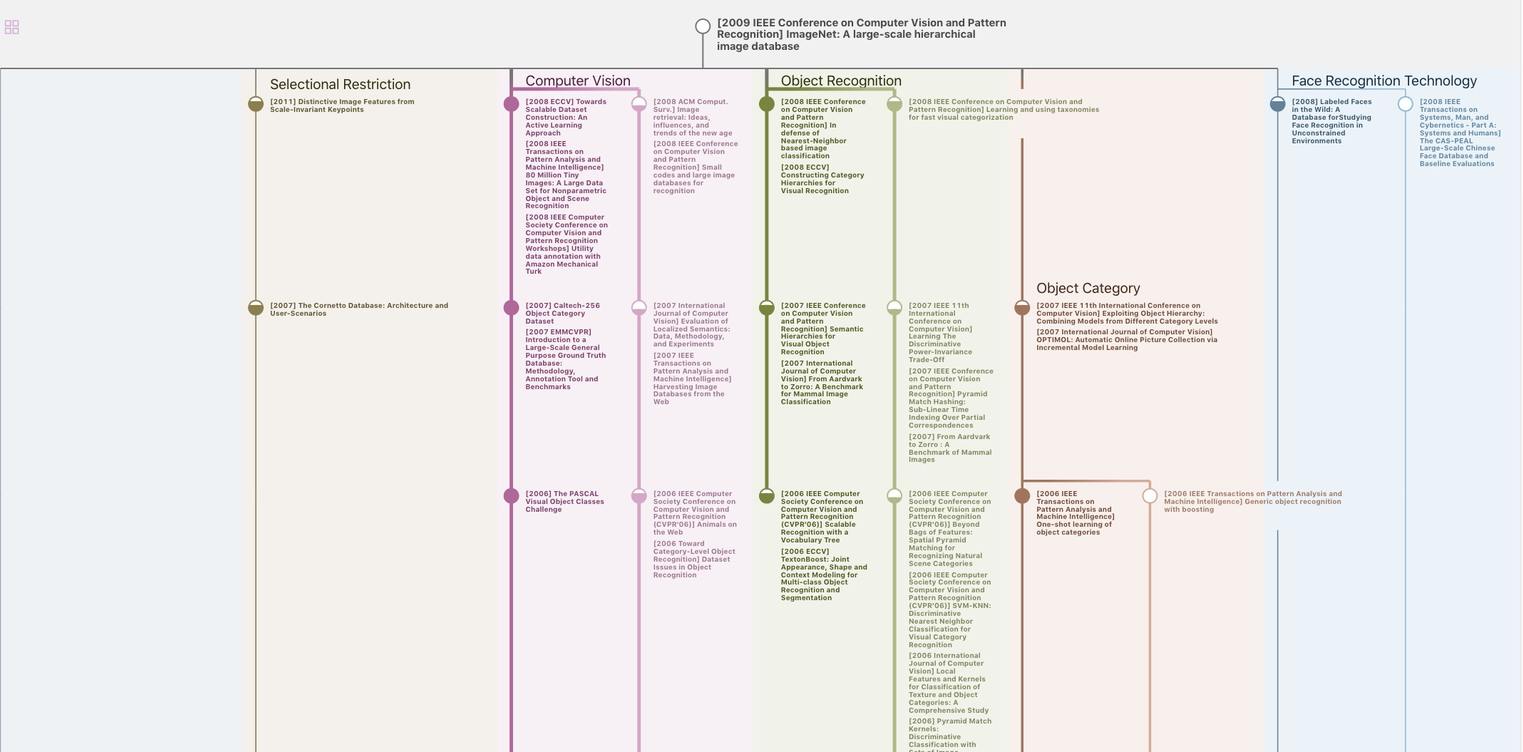
生成溯源树,研究论文发展脉络
Chat Paper
正在生成论文摘要