CNN-RNN and Data Augmentation Using Deep Convolutional Generative Adversarial Network for Environmental Sound Classification
IEEE SIGNAL PROCESSING LETTERS(2022)
摘要
Deep neural networks in deep learning have been widely demonstrated to have higher accuracy and distinct advantages over traditional machine learning methods in extracting data features. While convolutional neural networks (CNNs) have shown great success in feature extraction and audio classification, it is important to note that real-time audios are dependent on previous scenes. Also, the main drawback of deep learning algorithms is that they need a huge number of datasets to indicate their efficient performance. In this paper, a recurrent neural network (RNN) combined with CNN is proposed to address this problem. Moreover, a Deep Convolutional Generative Adversarial Network (DCGAN) is used for high-quality data augmentation. This data augmentation technique is applied to the UrbanSound8K dataset to improve the environmental sound classification. Batch normalization, transfer learning, and three feature representations map are used to improve the model accuracy. The results show that the generated images by DCGAN have similar features to the original training images and has the capability to generate spectrograms and improve the classification accuracy. Experimental results on UrbanSound8K datasets demonstrate that the proposed CNN-RNN architecture achieves better performance than the state-of-the-art classification models.
更多查看译文
关键词
Feature extraction,Convolution,Data models,Deep learning,Convolutional neural networks,Training,Generators,Data augmentation,deep convolutional genera-tive adversarial networks,environmental sound classification,convolutional recurrent neural network (CRNN)
AI 理解论文
溯源树
样例
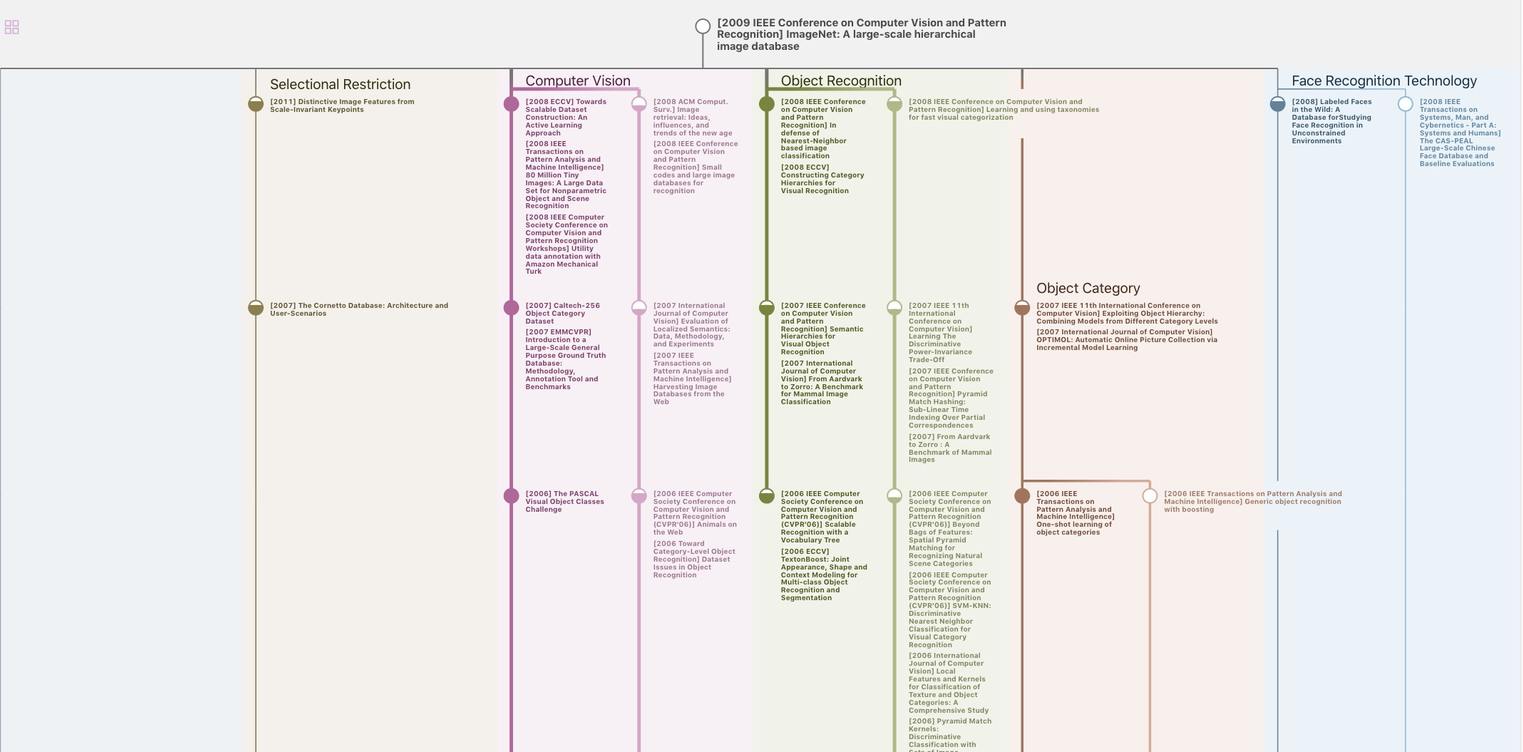
生成溯源树,研究论文发展脉络
Chat Paper
正在生成论文摘要