Deep learning model to assist multiphysics conjugate problems
PHYSICS OF FLUIDS(2022)
摘要
The availability of accurate and efficient numerical simulation tools has become of utmost importance for the design and optimization phases of existing industrial processes. The latter requires the computation of multiple physical fields governed by coupled systems of partial differential equations and tends to require large computational resources. Recently, the coupling of machine learning techniques with numerical simulation tools has allowed lifting part of this computational burden, by replacing parts of the resolution process with trained neural networks, whose execution cost is far less than their traditional counterparts. In this work, an auto-encoder convolutional neural network is suggested to reduce the resolution cost of the forced cooling of a hot workpiece in a confined space by modeling the scalar transport equation coupled to the Navier-Stokes equations. Although the proposed model was trained on a relatively limited amount of data, it was able to generalize accurately for different cooling setups with different inlet locations, thus leading to a reliable deep learning-assisted numerical solver.
更多查看译文
关键词
learning
AI 理解论文
溯源树
样例
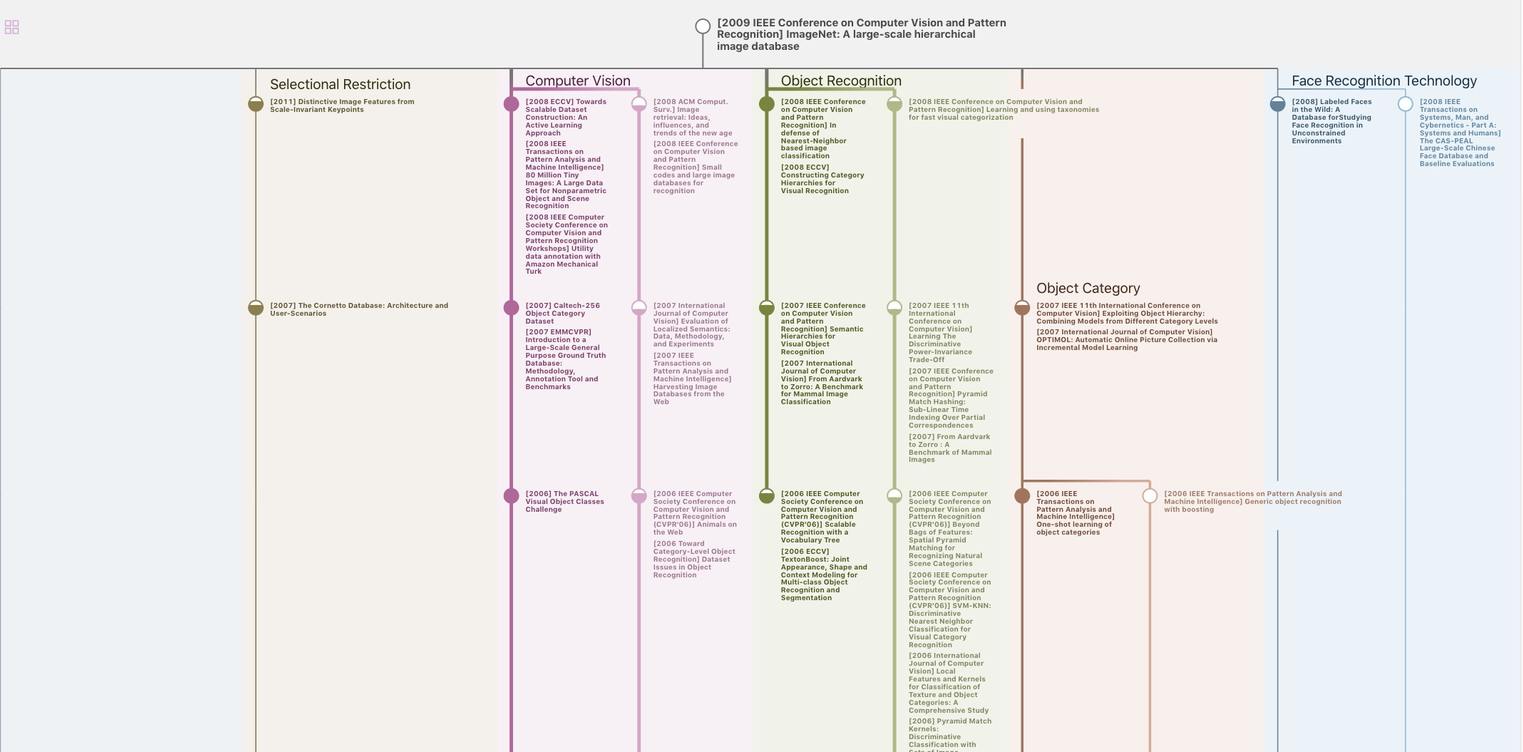
生成溯源树,研究论文发展脉络
Chat Paper
正在生成论文摘要