MSL-MNN: image deraining based on multi-scale lightweight memristive neural network
Neural Computing and Applications(2022)
摘要
Images and videos captured in rainy conditions show visual degradation, resulting in poor performance for outdoor computer vision systems. Traditional deraining methods fail to work in moderate and torrential rain removal, while the data-driven learning-based methods are hindered by removing rain streaks in an efficient manner, and lack consideration applied to edge devices with limited resources. So, this paper proposes a software-hardware co-designed image deraining system named MSL-MNN based on the multi-scale lightweight memristive neural network, which combines deep learning and the nanoscale device memristor. Specifically, a novel feature extraction block ReFiSE based on the lightweight residual mode and squeeze-and-excitation operation is designed. Benefiting from this, MSL-MNN is endowed with a lightweight network framework. Then, the multi-scale skip connection design is introduced to encourage the decoder to use low-level semantic information. Besides, a hardware implementation scheme of MSL-MNN is presented by leveraging the memristor crossbar array, which organically combines the deep learning algorithm with the neuro-inspired computing chip to speed the real-time processing of image data. Finally, a series of experimental results prove the effectiveness and superiority of MSL-MNN in rain removal and the recovery of image details. This study is expected to provide an end-to-end algorithm design and hardware acceleration solution for intelligent image processing besides image deraining.
更多查看译文
关键词
Image deraining, Lightweight, Deep learning, Memristor, edge device
AI 理解论文
溯源树
样例
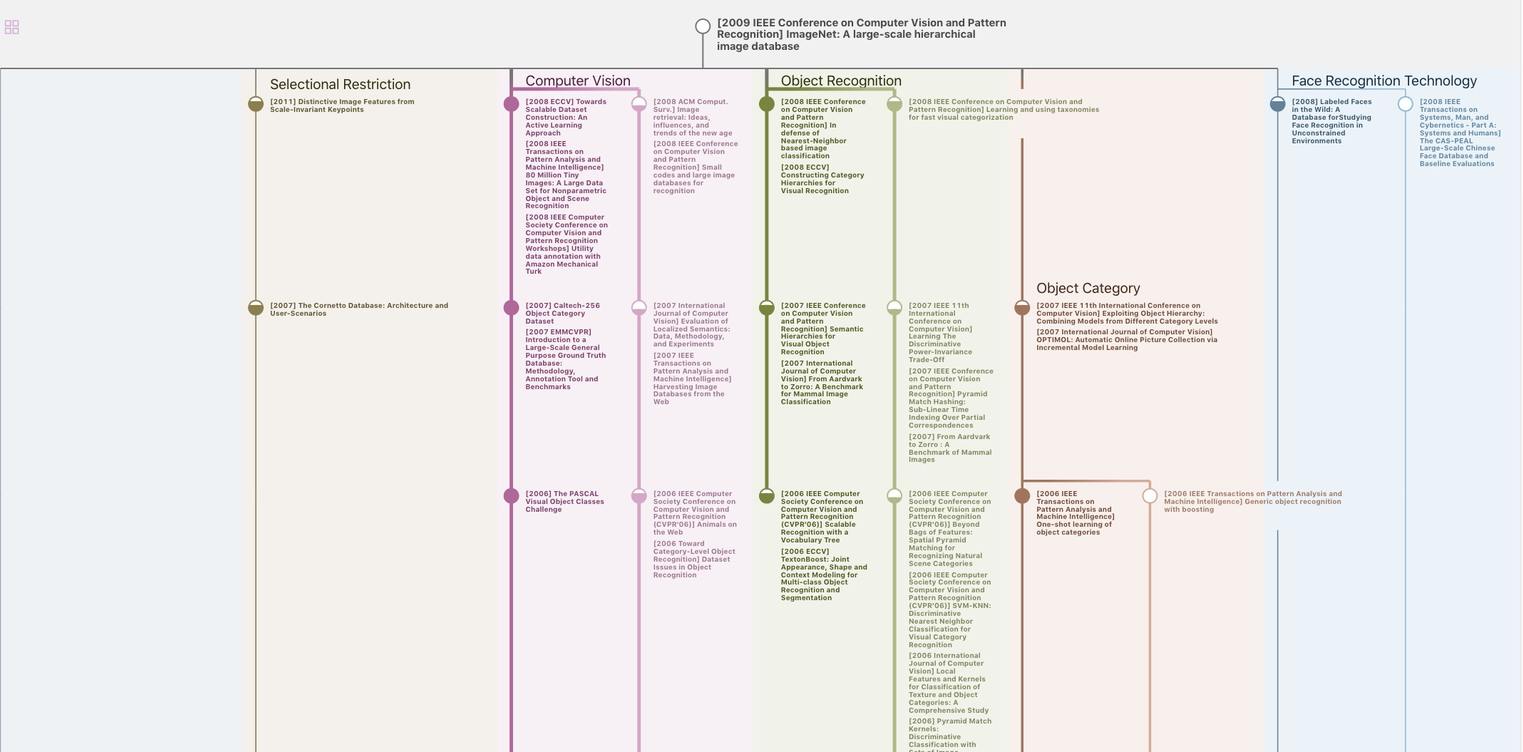
生成溯源树,研究论文发展脉络
Chat Paper
正在生成论文摘要