Variance reduction for root-finding problems
Mathematical Programming(2022)
摘要
Minimizing finite sums of smooth and strongly convex functions is an important task in machine learning. Recent work has developed stochastic gradient methods that optimize these sums with less computation than methods that do not exploit the finite sum structure. This speedup results from using efficiently constructed stochastic gradient estimators, which have variance that diminishes as the algorithm progresses. In this work, we ask whether the benefits of variance reduction extend to fixed point and root-finding problems involving sums of nonlinear operators. Our main result shows that variance reduction offers a similar speedup when applied to a broad class of root-finding problems. We illustrate the result on three tasks involving sums of n nonlinear operators: averaged fixed point, monotone inclusions, and nonsmooth common minimizer problems. In certain “poorly conditioned regimes,” the proposed method offers an n -fold speedup over standard methods.
更多查看译文
关键词
Stochastic algorithm, Variance reduction, Root-finding algorithm, Operator splitting, Monotone inclusions, Saddle-point problems
AI 理解论文
溯源树
样例
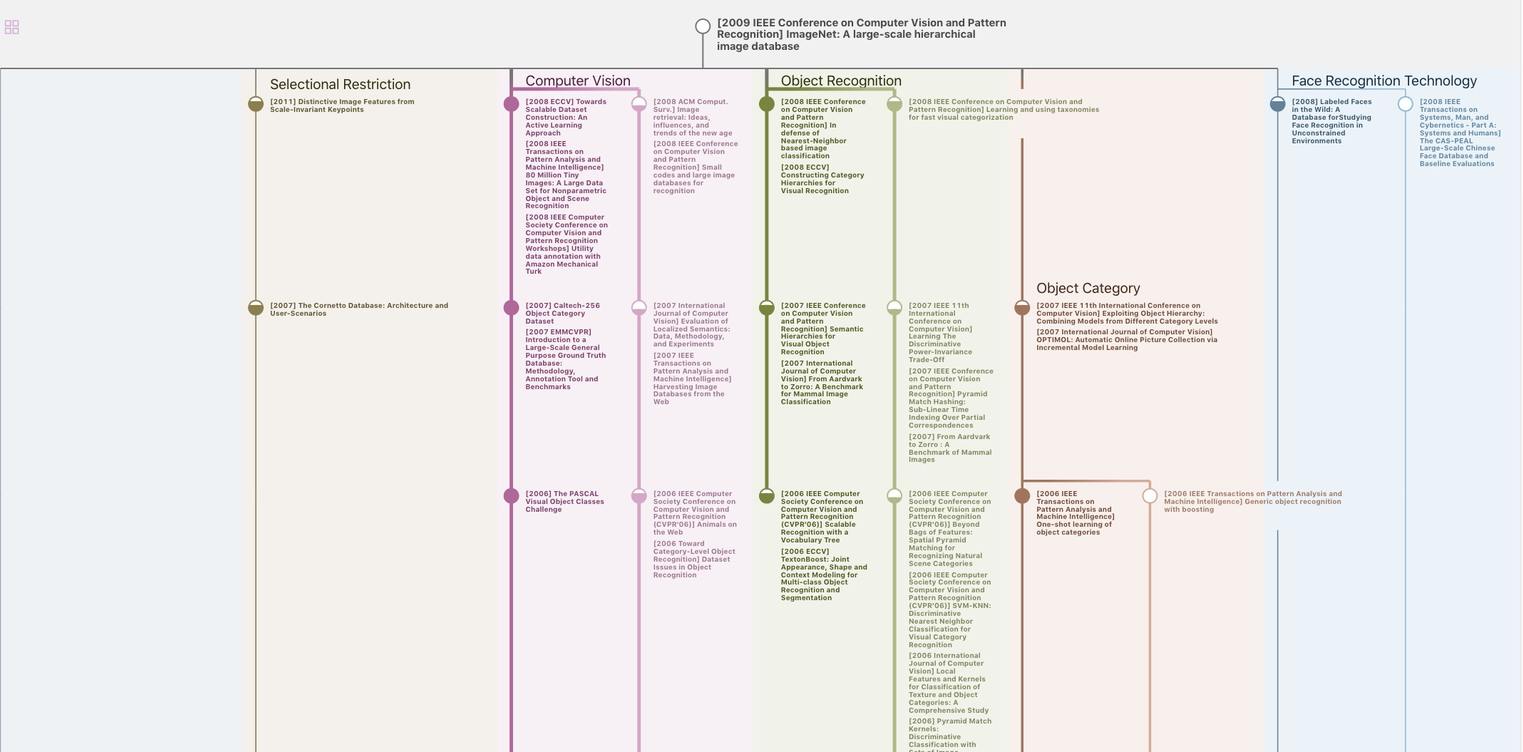
生成溯源树,研究论文发展脉络
Chat Paper
正在生成论文摘要