Adaptive Hardness Indicator Softmax for Deep Face Recognition
INTERNATIONAL JOURNAL OF PATTERN RECOGNITION AND ARTIFICIAL INTELLIGENCE(2022)
摘要
Due to their simplicity and efficiency, the margin-based Softmax losses are proposed to enhance feature discrimination in face recognition. Recently, the strategy of hard sample mining is incorporated to the margin-based Softmax losses for focusing the misclassified samples and achieves superior performance. However, the current mining-based Softmax losses indicate the sample difficultness only from the perspective of the negative cosine similarity, which is local and not robust. To obtain more discriminative deep face features, a novel adaptive hardness indicator Softmax (AHI-Softmax) loss is proposed in this paper to fully exploit the hardness information of samples. Our AHI-Softmax firstly defines a global sample hardness indicator function that integrates three difficultness factors to robustly indicate the level of "hardness" in numerical form. Then, a training stage indicator is incorporated to avoid the convergence issue. Finally, a novel sample-related modulation coefficient of the negative cosine similarity which combines the global and local hardness indicator will be defined to further enhance the differentiation of constraints imposed on samples. The experimental results on general face datasets, including LFW, AgeDB-30, CFP-FP, CALFW, CPLFW, MegaFace, IJB-B and IJB-C, show that our method can obtain more discriminative features and achieve superior verification and recognition results.
更多查看译文
关键词
Deep face recognition,margin-based loss functions,hard samples,adaptive mining-based strategies
AI 理解论文
溯源树
样例
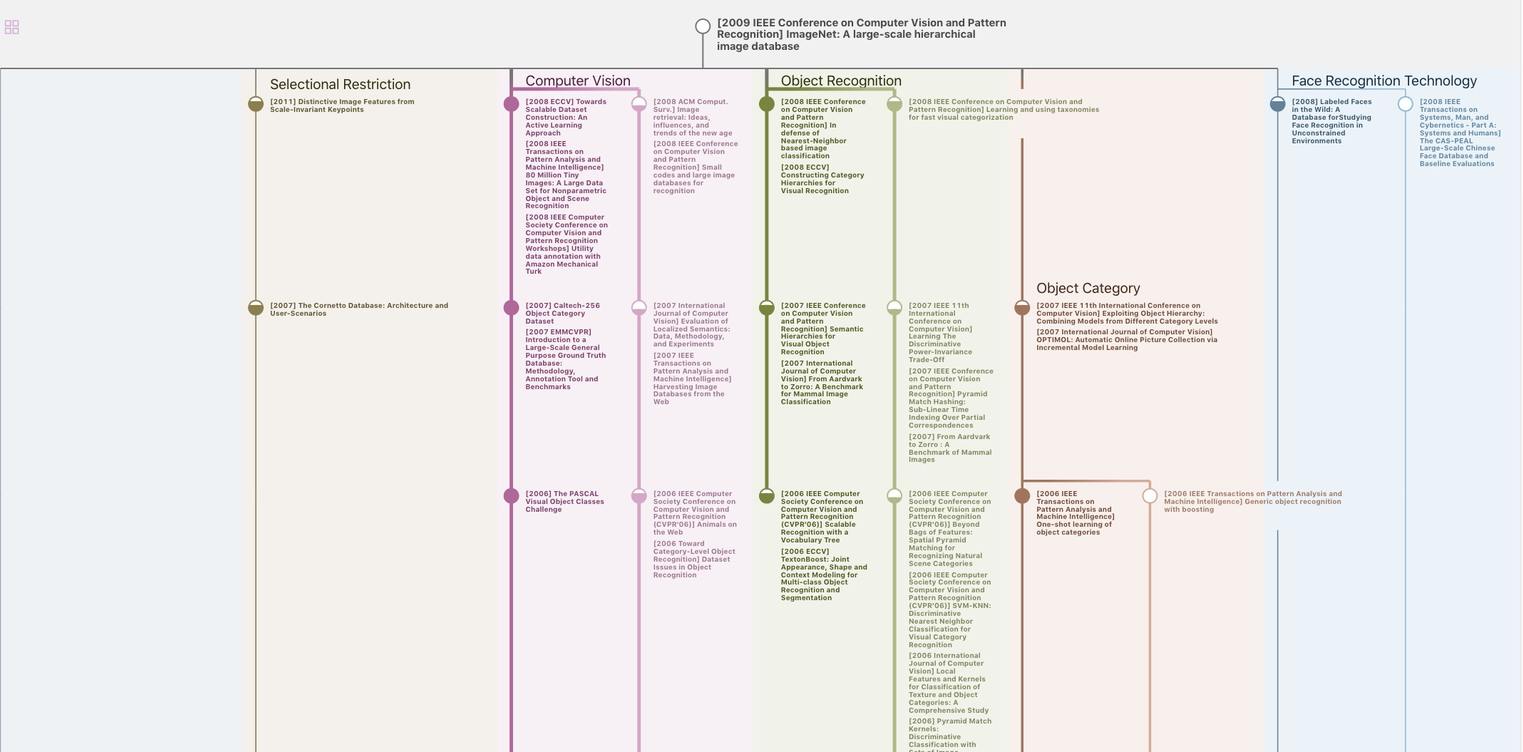
生成溯源树,研究论文发展脉络
Chat Paper
正在生成论文摘要