Extending the Detection Range for Low-Channel Roadside LiDAR by Static Background Construction
IEEE TRANSACTIONS ON GEOSCIENCE AND REMOTE SENSING(2022)
摘要
Further detection will increase traffic safety by perceiving unexpected traffic incidents earlier, allowing more time to make decisions and implement these decisions. Therefore, this article attempts to extend the detection range by using a low-channel roadside light detection and range sensor (LiDAR) sensor due to its low price and widespread employment in the future. The proposed method contains two major parts: static background construction and traffic objects detection. For static background construction, successive point cloud frames data were used to cover the most LiDAR scanning horizontal-vertical angle and obtain background information ultimately. Furthermore, the constructed static background was optimized to reduce the missing of the far range points and the occurrence of noise points. For vehicle and road user detection, the density-based spatial clustering of applications with noise (DBSCAN) algorithm was used to identify the near-range traffic objects. For far-range traffic objects detection, the trajectories of objects were extracted based on their moving direction and distance, and a fast Fourier transform (FFT) algorithm was used to filter the noise point and identify the vehicle and road user points. The experimental results show that the static background construction with the proposed method is more effective and uses fewer point cloud data. The average maximum detection range can be up to 100, 100, and 85 m for vehicles, cyclists, and pedestrians, respectively. The average precision (AP) and recall are 95.71% and 90.62%, respectively, which are better than the previous studies.
更多查看译文
关键词
Laser radar,Sensors,Roads,Point cloud compression,Feature extraction,Filtering,Visualization,Extending detection range,far-range detection,roadside light detection and range sensor (LiDAR) data,static background construction,traffic perception
AI 理解论文
溯源树
样例
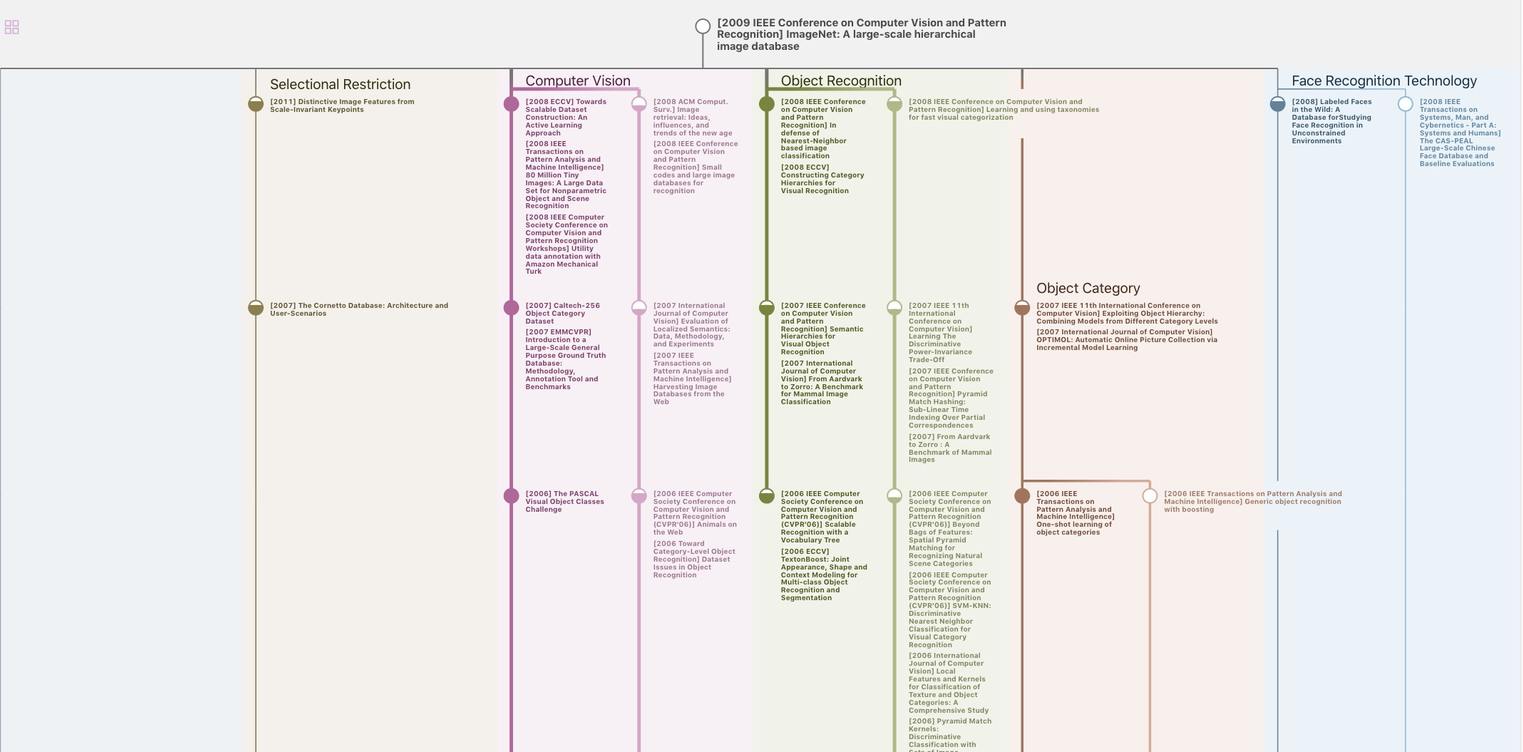
生成溯源树,研究论文发展脉络
Chat Paper
正在生成论文摘要