Coverage Prediction of Novel Coronavirus Vaccine Based on Deep Learning Time Series Analysis
2021 International Conference on Computer Information Science and Artificial Intelligence (CISAI)(2021)
摘要
Since the beginning of 2020, COVID-19 has swept the world, bringing many inconveniences and even threats to human life. Through medical scientists' constant study, the vaccine was finally developed earlier this year. According to mathematical and medical modelling, if novel coronavirus transmission is counted as three (i.e., one patient can infect three), 70% of the vaccinations will be required for protection to be substantially achieved. To tackle the issue of vaccine coverage prediction, this paper proposed three-time series analysis models, which can be utilized to analyze and predict the COVID-19 Vaccine coverage worldwide with the application of machine learning. For a long time, statistical methods have mostly solved time series prediction problems (AR, AM, ARMA, ARIMA). Mathematicians try to constantly refine these techniques to constrain stationary and non-stationary time series, but the results are often not very good. In this paper, we propose a method based on deep learning, using CNN-LSTM, VAE-LSTM, DeepAR, and other models to analyze and predict the data of vaccine coverage rate. The experimental results demonstrated that the RMSE of LSTM, CNN-LSTM, VAE-LSTM and DeepAR are 9.295522e+07, 1.028151e+07, 1.857031e+06 and 1.961001e+07 separately.
更多查看译文
关键词
COVID-19 vaccine,CNN- LSTM,VAE-LSTM,Deep AR
AI 理解论文
溯源树
样例
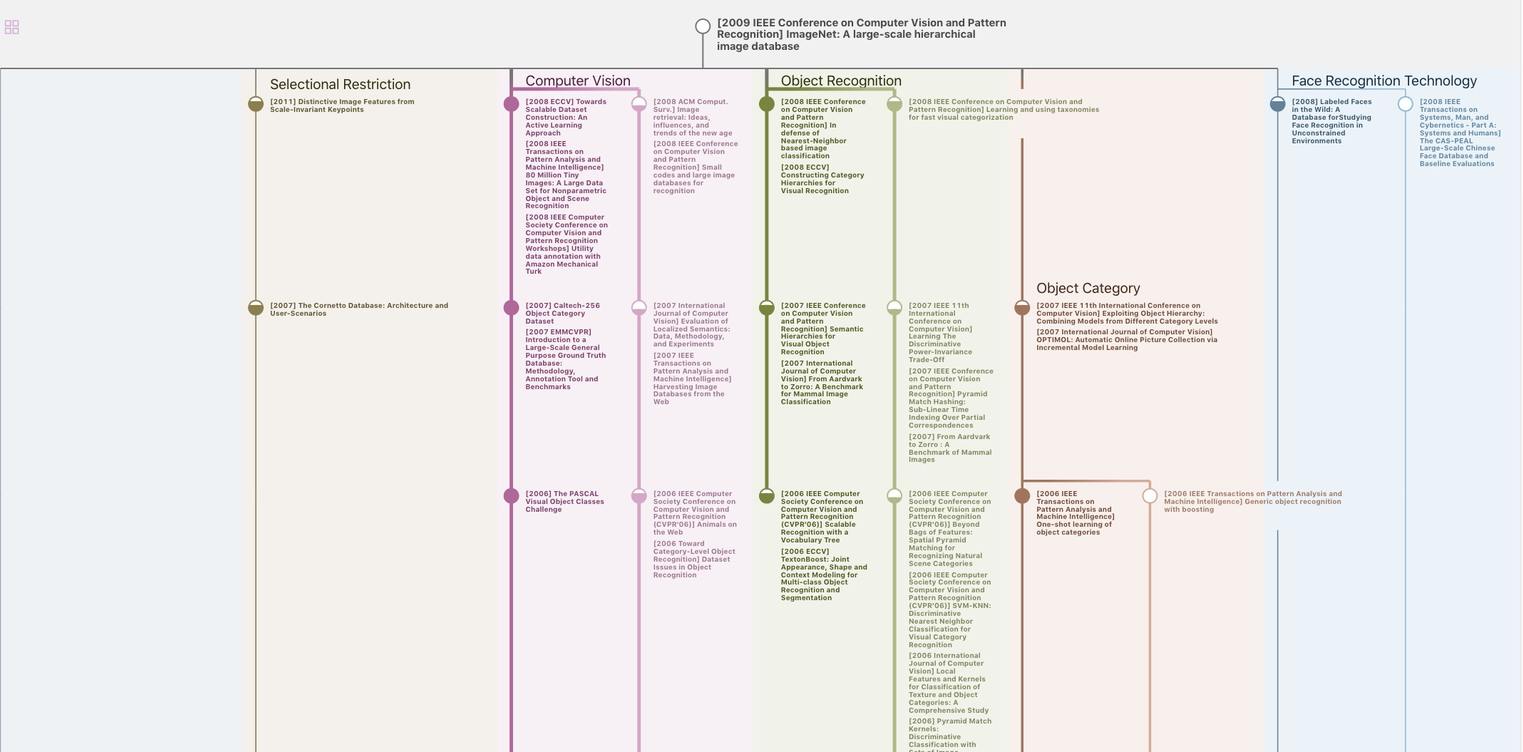
生成溯源树,研究论文发展脉络
Chat Paper
正在生成论文摘要