Predicting Elevated TSH Levels in the Physical Examination Population With a Machine Learning Model
FRONTIERS IN ENDOCRINOLOGY(2022)
摘要
ObjectiveThe purpose of this study was to predict elevated TSH levels by developing an effective machine learning model based on large-scale physical examination results. MethodsSubjects who underwent general physical examinations from January 2015 to December 2019 were enrolled in this study. A total of 21 clinical parameters were analyzed, including six demographic parameters (sex, age, etc.) and 15 laboratory parameters (thyroid peroxidase antibody (TPO-Ab), thyroglobulin antibody (TG-Ab), etc.). The risk factors for elevated TSH levels in the univariate and multivariate Logistic analyses were used to construct machine learning models. Four machine learning models were trained to predict the outcome of elevated TSH levels one year/two years after patient enrollment, including decision tree (DT), linear regression (LR), eXtreme Gradient boosting (XGBoost), and support vector machine (SVM). Feature importance was calculated in the machine learning models to show which parameter plays a vital role in predicting elevated TSH levels. ResultsA total of 12,735 individuals were enrolled in this study. Univariate and multivariate Logistic regression analyses showed that elevated TSH levels were significantly correlated with gender, FT3/FT4, total cholesterol (TC), TPO-Ab, Tg-Ab, creatinine (Cr), and triglycerides (TG). Among the four machine learning models, XGBoost performed best in the one-year task of predicting elevated TSH levels (AUC (0.87(+/- 0.03))). The most critical feature in this model was FT3/FT4, followed by TPO-Ab and other clinical parameters. In the two-year task of predicting TSH levels, none of the four models performed well. ConclusionsIn this study, we trained an effective XGBoost model for predicting elevated TSH levels one year after patient enrollment. The measurement of FT3 and FT4 could provide an early warning of elevated TSH levels to prevent relative thyroid diseases.
更多查看译文
关键词
elevated TSH level,machine learning model,antithyroid peroxidase-antibody (TPO-Ab),logistic analysis,free triiodothyronine (FT3) to thyroxine (FT4)
AI 理解论文
溯源树
样例
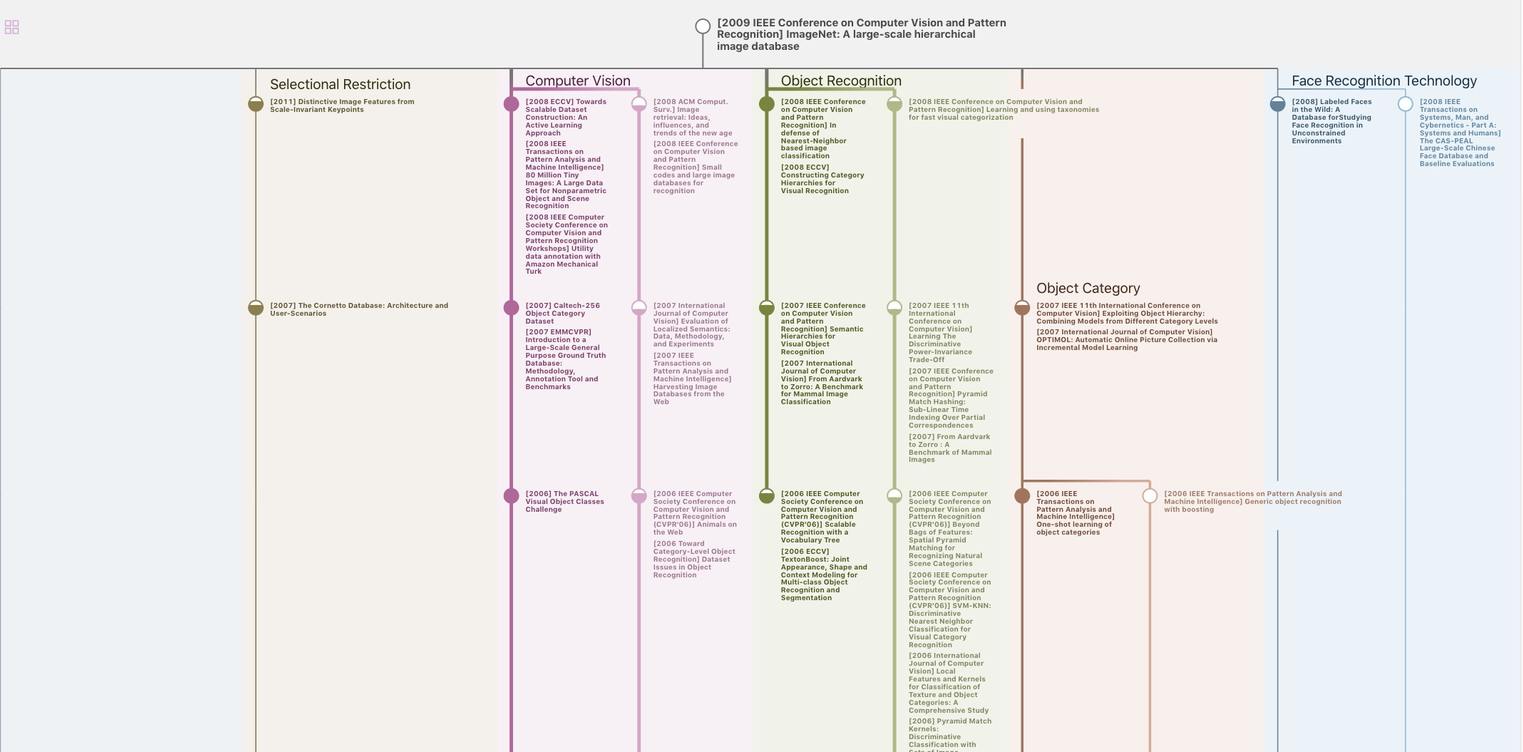
生成溯源树,研究论文发展脉络
Chat Paper
正在生成论文摘要