Truthful User Recruitment for Cooperative Crowdsensing Task: A Combinatorial Multi-Armed Bandit Approach
IEEE Transactions on Mobile Computing(2023)
摘要
Mobile Crowdsensing (MCS) is a promising paradigm that recruits users to cooperatively perform a sensing task. When recruiting users, existing works mainly focus on selecting a group of users with the best objective ability, e.g., the user's probability or frequency of covering the task locations. However, we argue that the task completion effect depends not only on the user's objective ability, but also on their subjective collaboration likelihood with each other. Furthermore, even though we can find a well-behaved group of users in the single-round scenario, while in the multi-round scenario without enough prior knowledge, we still face the problem of recruiting previously well-behaved user groups (exploitation) or recruiting uncertain user groups (exploration). Additionally, we consider that each user has a different cost, and the platform recruits users under a cost budget; thus, the problem becomes more challenging: users may report fake costs to gain more profits. To address these problems, assuming that the user's information is known, we first convert the single-round user recruitment problem into the min-cut problem and propose a graph theory based algorithm to find the approximate solution. Then, in the multi-round scenario where the user's information is estimated from the previous rounds, to balance the trade-off between exploration and exploitation, we propose the multi-round User Recruitment strategy under the budget constraint based on the combinatorial Multi-armed Bandit model (URMB), which is proven to achieve a tight regret bound. Next, we propose a graph-based payment strategy to achieve truthfulness and individual rationality of users. Finally, extensive experiments on three real-world datasets show that URMB always outperforms the state-of-th-art strategies.
更多查看译文
关键词
Task analysis,Recruitment,Costs,Collaboration,Sensors,Approximation algorithms,Target tracking,Mobile crowdsensing,collaboration likelihood,combinatorial multi-armed bandit,min-cut,truthful
AI 理解论文
溯源树
样例
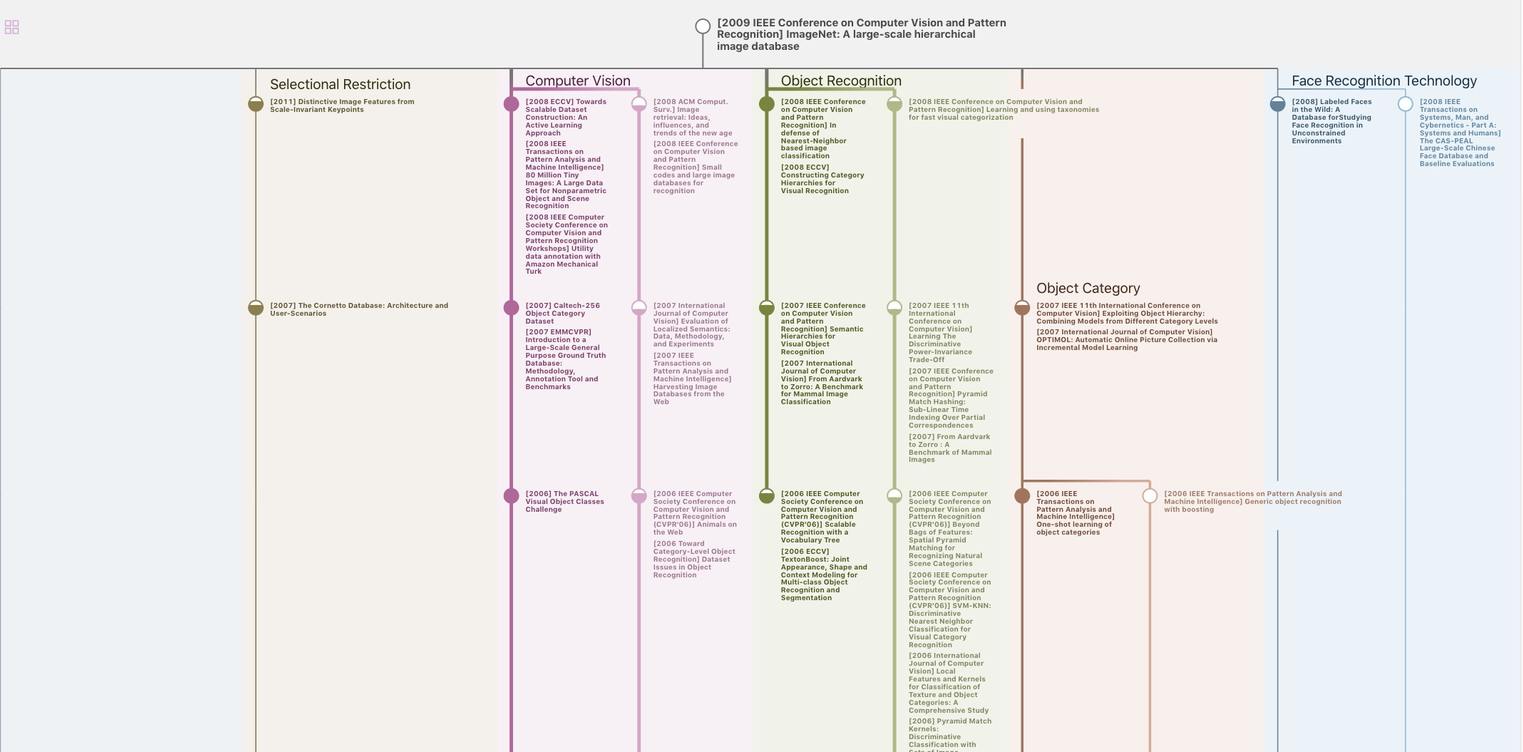
生成溯源树,研究论文发展脉络
Chat Paper
正在生成论文摘要