Generative Adversarial Networks‐Based Synthetic Microstructures for Data‐Driven Materials Design
Advanced Theory and Simulations(2022)
摘要
To understand the material paradigm, data-driven material design necessitates both microstructural input and output in the form of visual images. Therefore, generative adversarial networks (GAN)-based deep convolutional GAN, cycle-consistent GAN, and super-resolution GAN techniques are used to generate, translate, and improve the quality of microstructural images in this study. The reconstructed virtual microstructural images are realistic and indistinguishable from the real ones. Furthermore, using GAN techniques to reconstruct microstructural image suggests promising ways to design desired microstructures using parameterized descriptors and image augmentation, which are expected to advance data-driven materials research.
更多查看译文
关键词
cycle generative adversarial networks,data-driven material design,deep convolutional generative adversarial networks,microstructural-image reconstruction,super-resolution generative adversarial networks
AI 理解论文
溯源树
样例
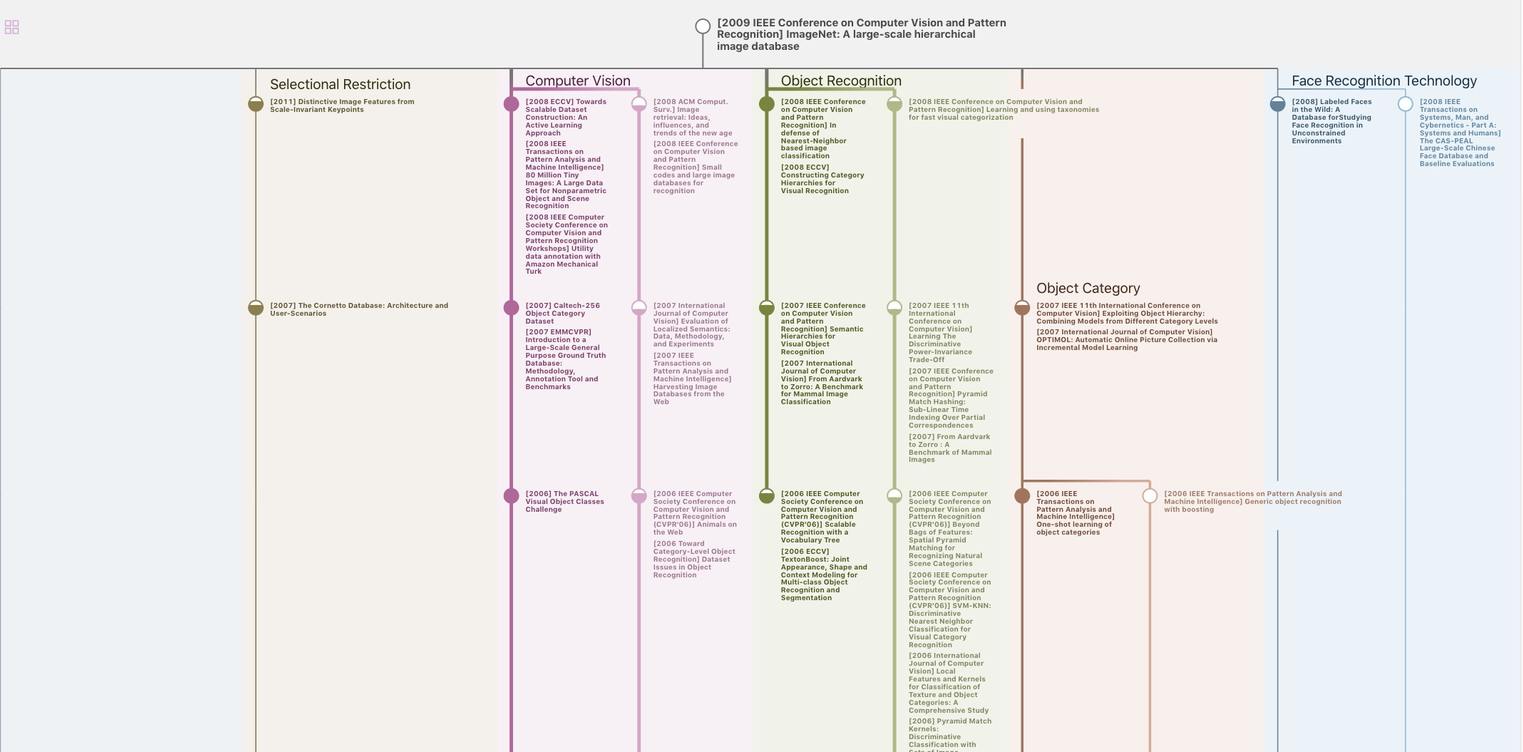
生成溯源树,研究论文发展脉络
Chat Paper
正在生成论文摘要