Fastcelf++: A Novel and Fast Heuristic for Influence Maximization in Complex Networks
SSRN Electronic Journal(2022)
摘要
Social networks reflect the relationships and interactions between individuals and have played a significant role in the spread of information, in which the communication of ideas and sharing of opinions happen all the time. There are various examples of how social networks can affect the behavior of individuals, like viral marketing, the spread of memes, and the propagation of fake news. This dynamic of information diffusion has motivated the research of several approaches to identify the prominent influencers in a network. The Influence Maximization Problem consists of identifying a subset S, called a seed set, of at most k elements to achieve the maximum (expected) propagation through a diffusion model, with S as the initial influencers on a network. It demonstrated that the influence maximization problem is an NP-hard optimization problem. Therefore, it is unfeasible to identify the subset S that ensures the most extensive diffusion due to its complexity. The most typical approach to this problem is using approximate algorithms, highlighting the Cost-Effective Lazy Forward (CELF), about 700 times faster than the greedy strategy proposed by Kemp et al., and CELF++, which presents a runtime gain of between 35 to 55% over CELF. This work modifies the two above-mentioned state-of-the-art algorithms, CELF and CELF++, replacing Monte Carlo simulations with functions to calculate the diffusion estimations (metamodels) for selecting a set of seeds. The adoption of well-known methods in the literature with metamodels can identify orders of magnitude faster, more influential individuals and, in some cases, even outperform the results of these methods in terms of propagation.
更多查看译文
关键词
influence maximization,complex networks
AI 理解论文
溯源树
样例
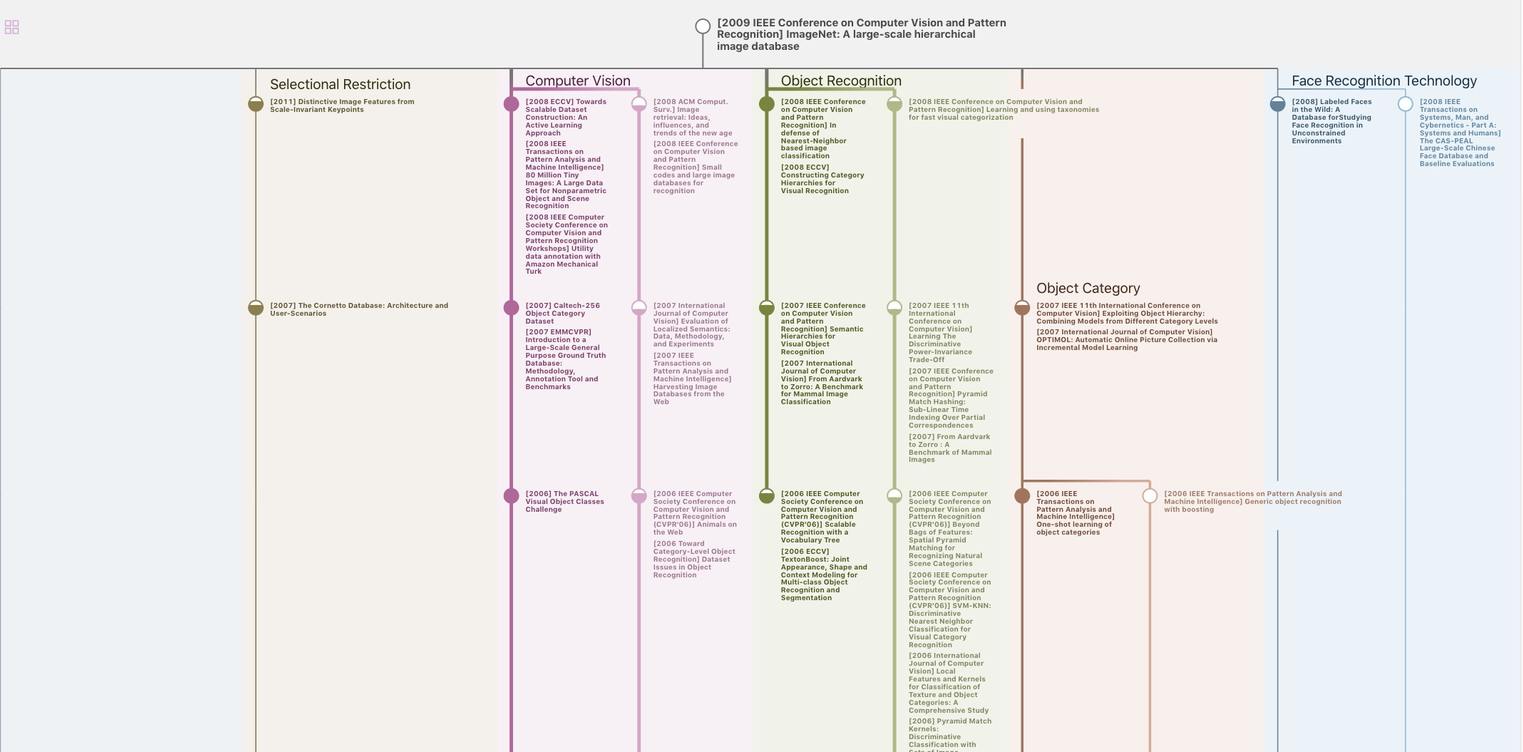
生成溯源树,研究论文发展脉络
Chat Paper
正在生成论文摘要