Cascaded Anomaly Detection with Coarse Sampling in Distributed Systems
BIG-DATA-ANALYTICS IN ASTRONOMY, SCIENCE, AND ENGINEERING, BDA 2021(2022)
摘要
In this contribution, analysis of usefulness of selected parameters of a distributed information system, for early detection of anomalies in its operation, is considered. Use of statistical analysis, or machine learning (ML), can result in high computational complexity and requirement to transfer large amount of data from the monitored system's elements. This enforces monitoring of only major components (e.g., access link, key machine components, filtering of selected traffic parameters). To overcome this limitation, a model in which an arbitrary number of elements could be monitored, using microservices, is proposed. For this purpose, it is necessary to determine the sampling threshold value and the influence of sampling coarseness on the quality of anomaly detection. To validate the proposed approach, the ST4000DM000 (Disk failure) and CICIDS2017 (DDoS) datasets were used, to study effects of limiting the number of parameters and the sampling rate reduction on the detection performance of selected classic ML algorithms. Moreover, an example of microservice architecture for coarse network anomaly detection for a network node is presented.
更多查看译文
关键词
Anomaly detection,Anomaly prediction,Complex distributed system,Computer network management
AI 理解论文
溯源树
样例
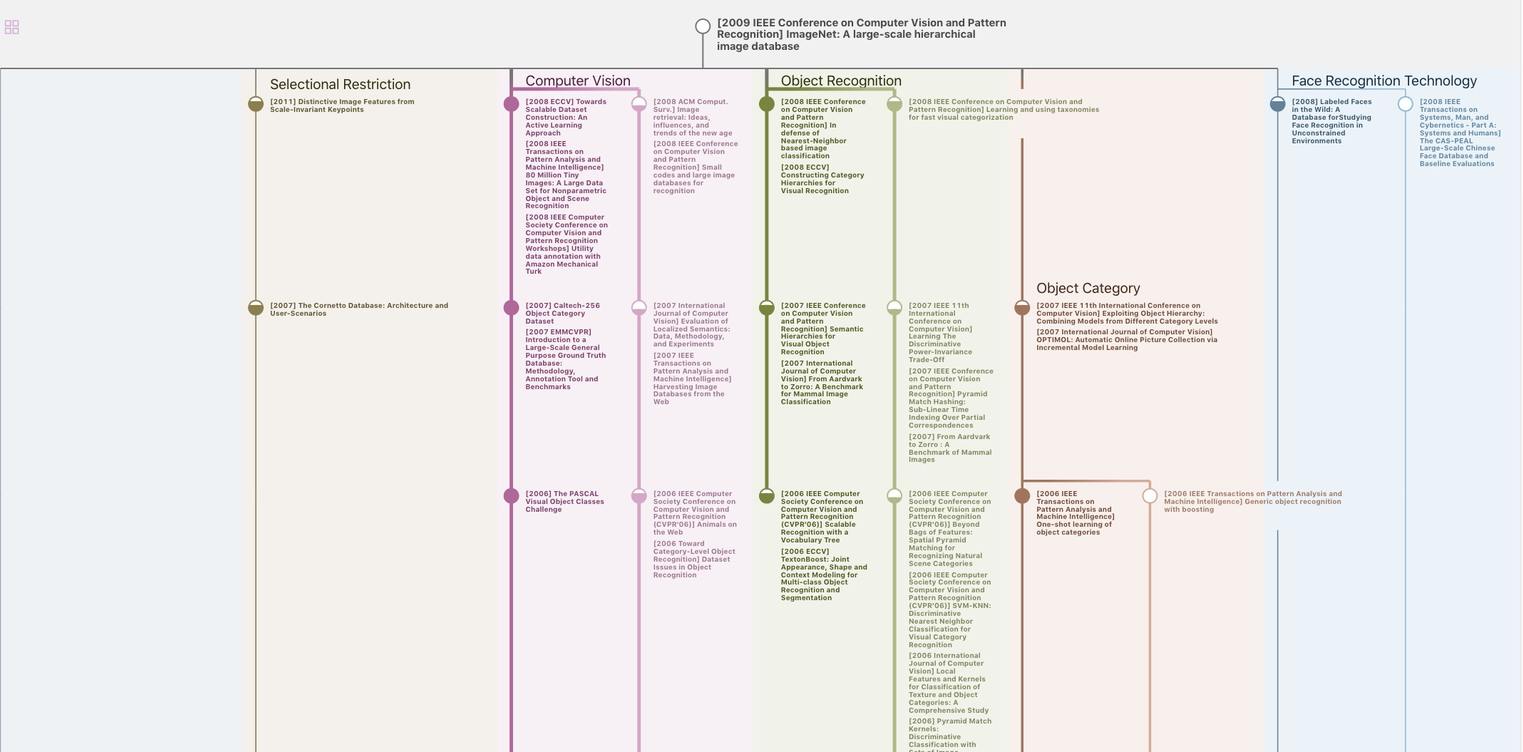
生成溯源树,研究论文发展脉络
Chat Paper
正在生成论文摘要