Explainable deep neural network for echocardiography view classification
European Heart Journal - Cardiovascular Imaging(2022)
摘要
Abstract Funding Acknowledgements Type of funding sources: Public grant(s) – National budget only. Main funding source(s): Ministry of Science and Technology, Taiwan Background Deep neural network may assist echocardiography interpretation in several tasks; however, the lack of expandability hindered its broader application since physicians couldn’t realize the rationale of interpretation clearly and comfortably. Method Retrospectively, we manually annotated 26,465 transthoracic echocardiography images into 29 classes for model development in 4:1 ratio as training and validation datasets. We added an autoencoder component into our model, adapted from capsule net, for view-classifying to deconvolute the feature maps of last layer as decoder for human interpretation. The performance of view classification was measured in accuracy and confusion matrix, and the interpretability of model were assessed by cardiologist. Result After appropriate model training, the accuracy of our model achieved averaged 98.2% for echocardiography view classification, ranged from lowest 80.0% in suprasternal view to 100% of several more common view in validation dataset. The successful deconvolution of feature map to reconstruct images showed essential independent components of echocardiography view and could be interpretated by cardiologist and clinical physicians. Conclusion With the use of autoencoder in model for echocardiography view classification showed maintainable good performance in accuracy and facilitated clinical interpretation to enhance its reliability. Abstract Figure. Model architecture and performance Abstract Figure. Example of explaniable deconvolution
更多查看译文
关键词
explainable deep neural network,echocardiography,deep neural network,classification,view
AI 理解论文
溯源树
样例
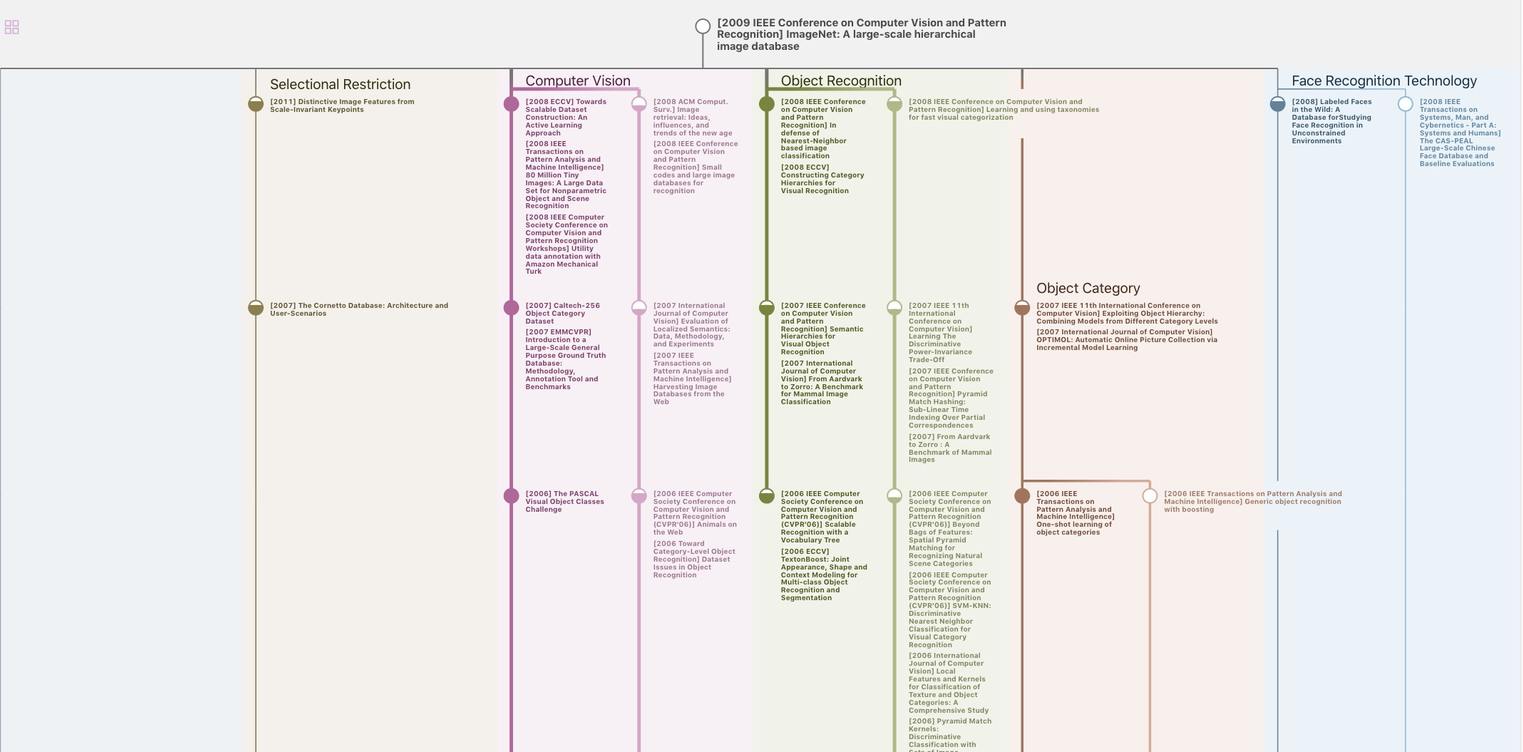
生成溯源树,研究论文发展脉络
Chat Paper
正在生成论文摘要