MMSTN: A Multi‐Modal Spatial‐Temporal Network for Tropical Cyclone Short‐Term Prediction
Geophysical Research Letters(2022)
摘要
Forecasting the trajectory and intensity of tropical cyclones (TCs) is important in disaster mitigation as TC usually causes huge damages. However, it remains a substantial challenge due to the limited understanding of TC complexity. Still, TCs have been observed and recorded for several decades, and so can be predicted if viewed as a spatial-temporal prediction problem with a huge amount of existing data. We propose a novel TC trajectory and intensity short-term prediction method: Multi-Modal Spatial-temporal Networks (MMSTN). It not only predicts the TC's central pressure, winds, and the location of its center, but also forecasts the TC's varied possible tendencies. Experiments were conducted on the China Meteorological Administration Tropical Cyclone Best Track Dataset. Experimental results show that the proposed MMSTN outperformed state-of-the-art methods as well as the official prediction method of the China Central Meteorological Observatory, in intensity prediction and 6 hr trajectory prediction. Plain Language Summary Tropical cyclones (TCs) are weather disasters that can cause huge economic losses and human casualties. It is thus necessary to establish a TC prediction system to help humans to defend against them. In this paper, we combine artificial intelligence with meteorological multi-modal data, including trajectory and intensity data, to propose a deep learning method for TC trajectory and intensity prediction. This model can take a short-term TC prediction and forecast its central pressure, winds, and the location of its center. The experimental results indicate that the short-term prediction performance of our method is better than the method of the China Central Meteorological Observatory in most indexes.
更多查看译文
AI 理解论文
溯源树
样例
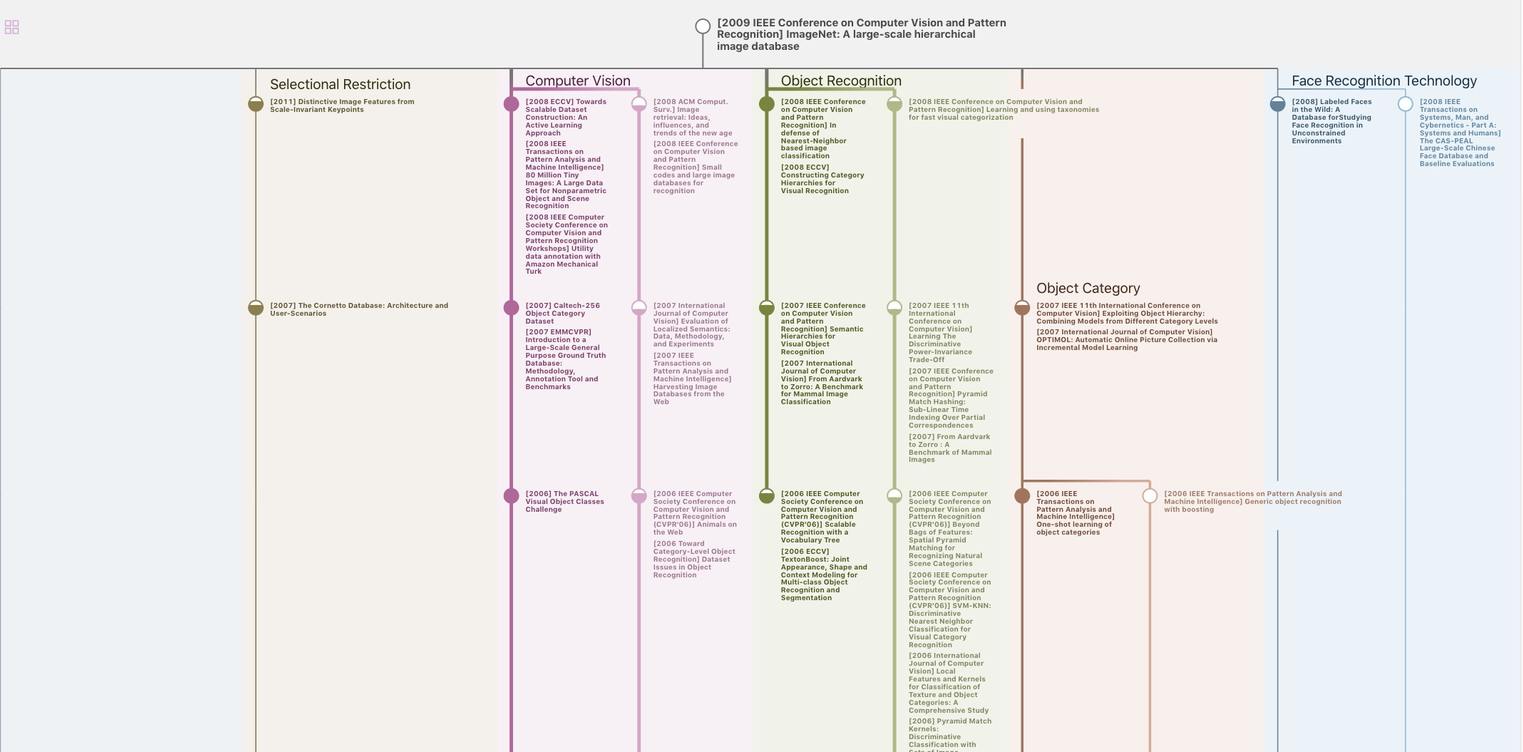
生成溯源树,研究论文发展脉络
Chat Paper
正在生成论文摘要