Mirror invariant convolutional neural networks for image classification
IET IMAGE PROCESSING(2022)
摘要
Deep convolutional neural networks (DCNNs) have been developed rapidly and they perform well on both classification and object detection tasks. However, its strong performance makes people ignore to study the invariance of the DCNNs, such as mirror invariance. In fact, the ability of DCNNs in handling mirror-symmetrical images remains limited. In this paper, a mirror transformation convolutional layer is proposed, which transforms several feature maps to produce mirror-symmetrical feature maps based on the traditional convolutional layer. By combining with the mirror transformation convolutional layer, the DCNNs will have mirror invariance and the performance of neural networks can be improved on classification tasks. A dataset for driver's and passenger's seatbelt detection has been collected, which is used to verify the effectiveness of the proposed convolutional layer. In the experiments, one of the state-of-the-art DCNNs, GoogLeNet, is collaborated with the mirror transformation convolutional layer to form a mirror invariant networks (MINets). The experimental results show that the MINets can achieve better classification performance than the original GoogLeNet. MINets can also reduce the risk of over-fitting caused by applying data augmentations to the dataset.
更多查看译文
AI 理解论文
溯源树
样例
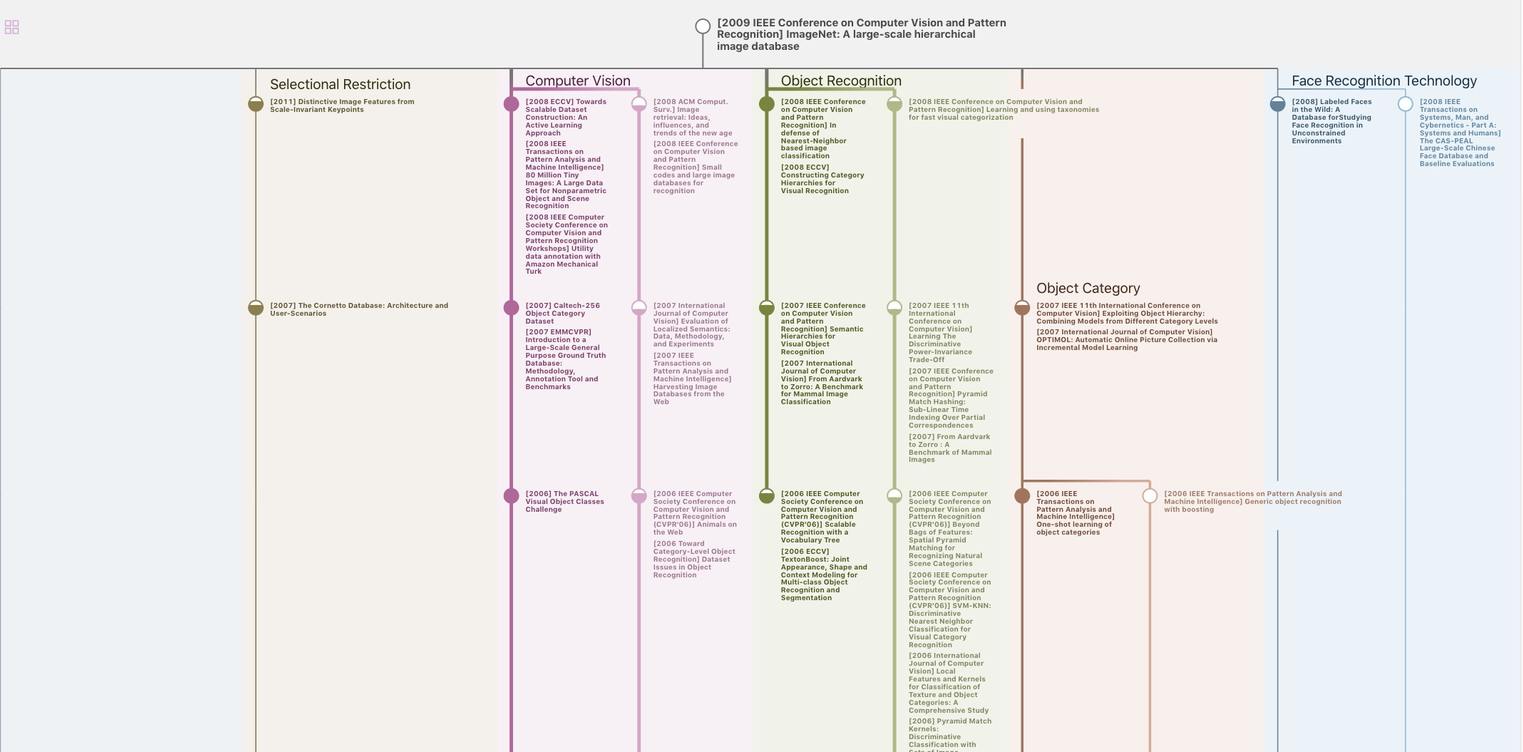
生成溯源树,研究论文发展脉络
Chat Paper
正在生成论文摘要