Predicting Ischemic Stroke In Emergency Departments: Development And Validation Of Machine Learning Models
Stroke(2022)
摘要
Background: Stroke misdiagnosis is estimated to occur in 9% of all stroke patients and is associated with poor outcomes. We hypothesized that machine learning (ML) could be used to detect ischemic stroke at the point of care in emergency departments (ED). Methods: Clinical data from 13 hospitals of a large health system in Pennsylvania, United States, from September 2003 to May 2019 were used for model development. Data from June 2019 to December 2020 were prospectively collected and divided into pre- and post-COVID cohorts for validation. We simulated three clinical settings by enrollment with different inclusion/exclusion criteria and created two ML pipelines: ML applied to pre-event clinical data, and natural language processing (NLP) with ML applied to clinical notes during triaging. Misdiagnosed stroke in ED was a case study to show the discriminative power. Results: We included a total of 49,155 patient encounters (8,900 consecutive ischemic stroke patients and 40,255 controls). The best model, based on the patient’s pre-event information, was XGBoost (AUROC=0.91). Using NLP+ML pipeline, we collected 2,070 notes during the triage process and 9,607 notes (non-stroke) for modeling. For independent validation, we identified 2,989 notes for stroke and 4,303 notes for non-stroke. We identified nine models that reached a balanced performance in terms of AUROC, sensitivity, and specificity. Model performance, in terms of AUROC, ranged from 0.876 to 0.985. Model sensitivity and specificity reached 0.993 and 0.975, respectively. There was no performance difference between models tested on pre- and post-COVID. Three out of four models correctly identified a separate case series of five misdiagnosed strokes. Conclusion: Available clinical information can be used to reduce stroke misdiagnosis in real-time. In addition, this study serves as a foundation for prospective trials to establish how pre-event clinical data and triage information can be used to improve care.
更多查看译文
AI 理解论文
溯源树
样例
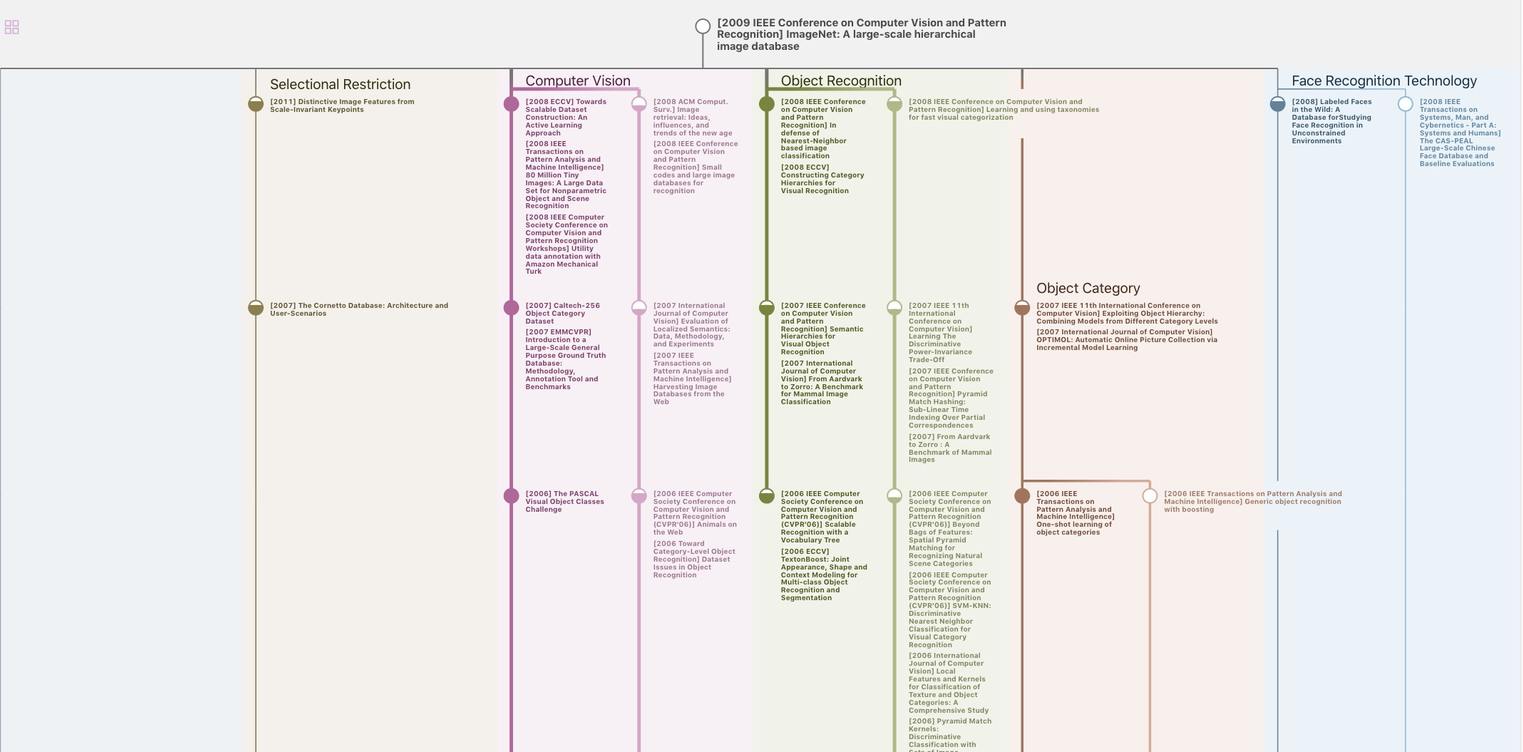
生成溯源树,研究论文发展脉络
Chat Paper
正在生成论文摘要