A Novel Deep Learning Bi-GRU-I Model for Real-Time Human Activity Recognition Using Inertial Sensors
IEEE SENSORS JOURNAL(2022)
摘要
Wearable sensor based Human Activity Recognition (HAR) has been widely used these years. This paper proposed a novel deep learning model for HAR using inertial sensors. First, a wearable device platform was developed with 6 inertial sensor units to collect triaxial acceleration signals during human movements, and the dataset of Command Actions of Traffic Police (CATP) was acquired. Then, a deep learning model named Bidirectional-Gated Recurrent Unit-Inception (Bi-GRU-I) was designed to improve the accuracy and reduce the amount of parameters. It is consisting of 2 Bi-GRU layers, 3 Inception layers, 1 Global Average Pooling (GAP) layer and 1 softmax layer. Finally, the comparing experiments with other methods were taken on 3 datasets: the self-collected CATP dataset, widely used Wireless Sensor Data Mining (WISDM) and University of California, Irvine (UCI-HAR) dataset. And the proposed method shows better performance and robustness. Moreover, the sensor configuration optimization was analyzed, and it shows that this method can also apply to the task using less sensor units.
更多查看译文
关键词
Sensors,Feature extraction,Deep learning,Inertial sensors,Data mining,Convolutional neural networks,Accelerometers,Human activity recognition (HAR),inertial sensor,deep learning,Bi-GRU,inception architecture
AI 理解论文
溯源树
样例
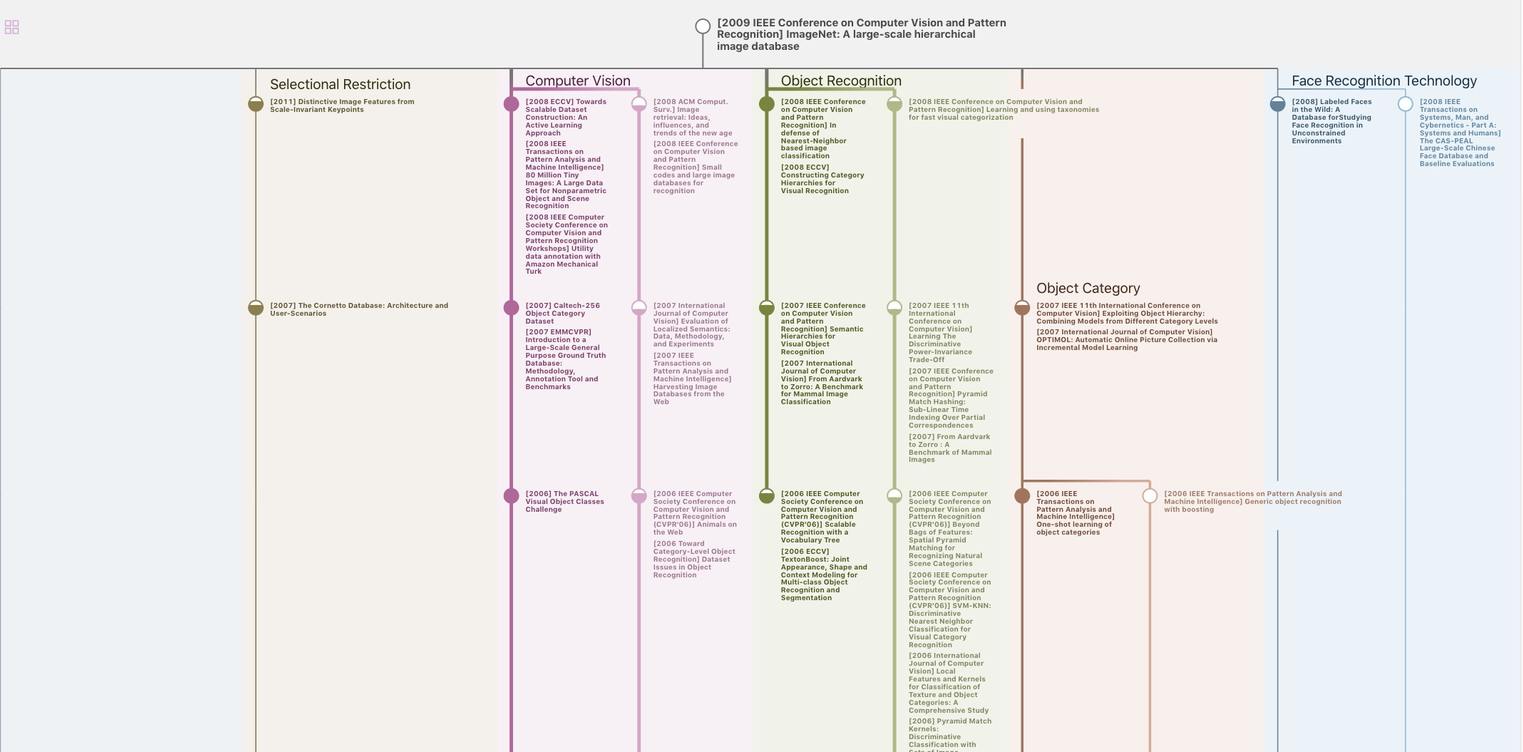
生成溯源树,研究论文发展脉络
Chat Paper
正在生成论文摘要