MOOC performance prediction and personal performance improvement via Bayesian network
Education and Information Technologies(2022)
摘要
In order to analyze the non-linear and uncertain relationships among the student-related features, curriculum-related features as well as the environment-related features, and then quantify the corresponding impacts on students’ final MOOC performance in a valid way, we first construct a Students’ performance Prediction Bayesian Network (SPBN) via the hill-climbing and maximum likelihood estimation (MLE) method. With SPBN, we can predict the students’ MOOC performance and then quantify the uncertain dependencies of all the relevant features. Furthermore, with the prediction results of SPBN, we further apply the genetic algorithm (GA) to offer the personal performance improvement suggestions for those who are about to fail the courses according to different students’ background and their current engagement behaviors, so as to avoid the MOOC exam failures in advance. The experiments conducted on the Open University Learning Analytics Dataset (OULAD) have shown that the SPBN can predict students’ performance in MOOC accurately, and the GA-based method can offer the reasonable performance improvement suggestions effectively.
更多查看译文
关键词
MOOC, Performance prediction, Performance improvement, Bayesian network, Genetic algorithm
AI 理解论文
溯源树
样例
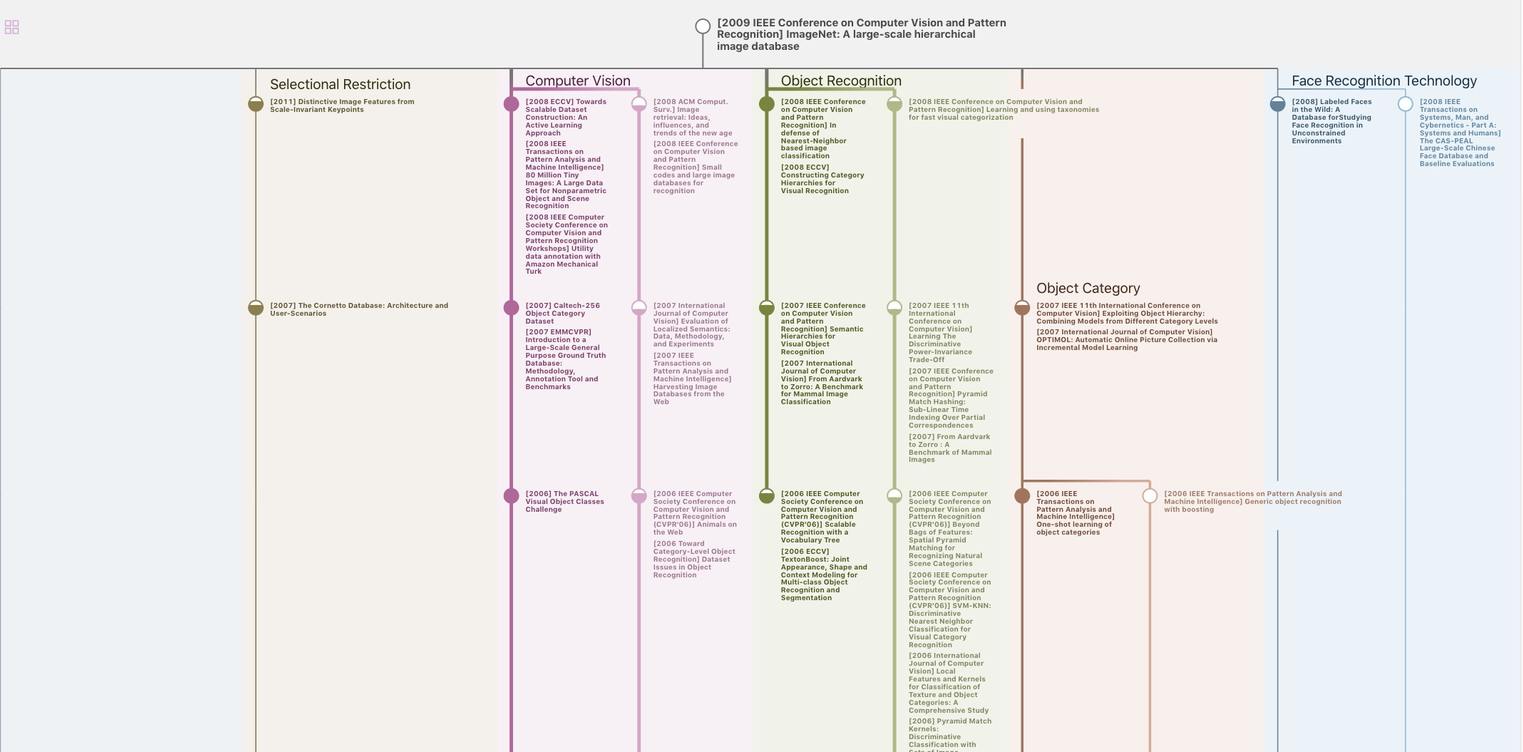
生成溯源树,研究论文发展脉络
Chat Paper
正在生成论文摘要