Coupled Noise Reduction in Distributed Acoustic Sensing Seismic Data Based on Convolutional Neural Network
IEEE GEOSCIENCE AND REMOTE SENSING LETTERS(2022)
摘要
Distributed acoustic sensing (DAS) is widely recognized as a new technology to replace conventional geophones for the acquisition of seismic data. However, the collected data often contain a lot of coupled noise due to cable slapping and ringing along the borehole casing, which brings great difficulties to the interpretation of seismic data. The existing conventional coupled noise reduction methods often need to estimate the parameters of each coupled noise (such as amplitude, noise period, attenuation coefficient, etc.), which takes a lot of time and cannot meet the requirements for large-data-volume DAS seismic data processing. In addition, some deep learning-based denoising methods lack detailed analysis on coupled noise and have problems in the construction of training sets, resulting in insufficient generalization ability of the denoising model. To solve these problems, we propose a coupled noise reduction method based on the convolutional neural network (CNN). The proposed method does not need to estimate the parameters of coupled noise, and the denoising process is more convenient and efficient. In addition, through the analysis of DAS seismic data, we also construct a training set for coupled noise reduction using real data and synthetic data. The denoising results of both synthetic data and field data show that the proposed method can effectively reduce the coupled noise in DAS seismic data, and the effective signal has almost no energy loss. After processing, the signal affected by coupled noise becomes clear and continuous, providing high-quality data support for subsequent interpretation.
更多查看译文
关键词
Noise reduction,Training,Attenuation,Convolutional neural networks,Data processing,Mathematical models,Geoscience and remote sensing,Convolutional neural network (CNN),coupled noise,denoise,distributed acoustic sensing (DAS),training set
AI 理解论文
溯源树
样例
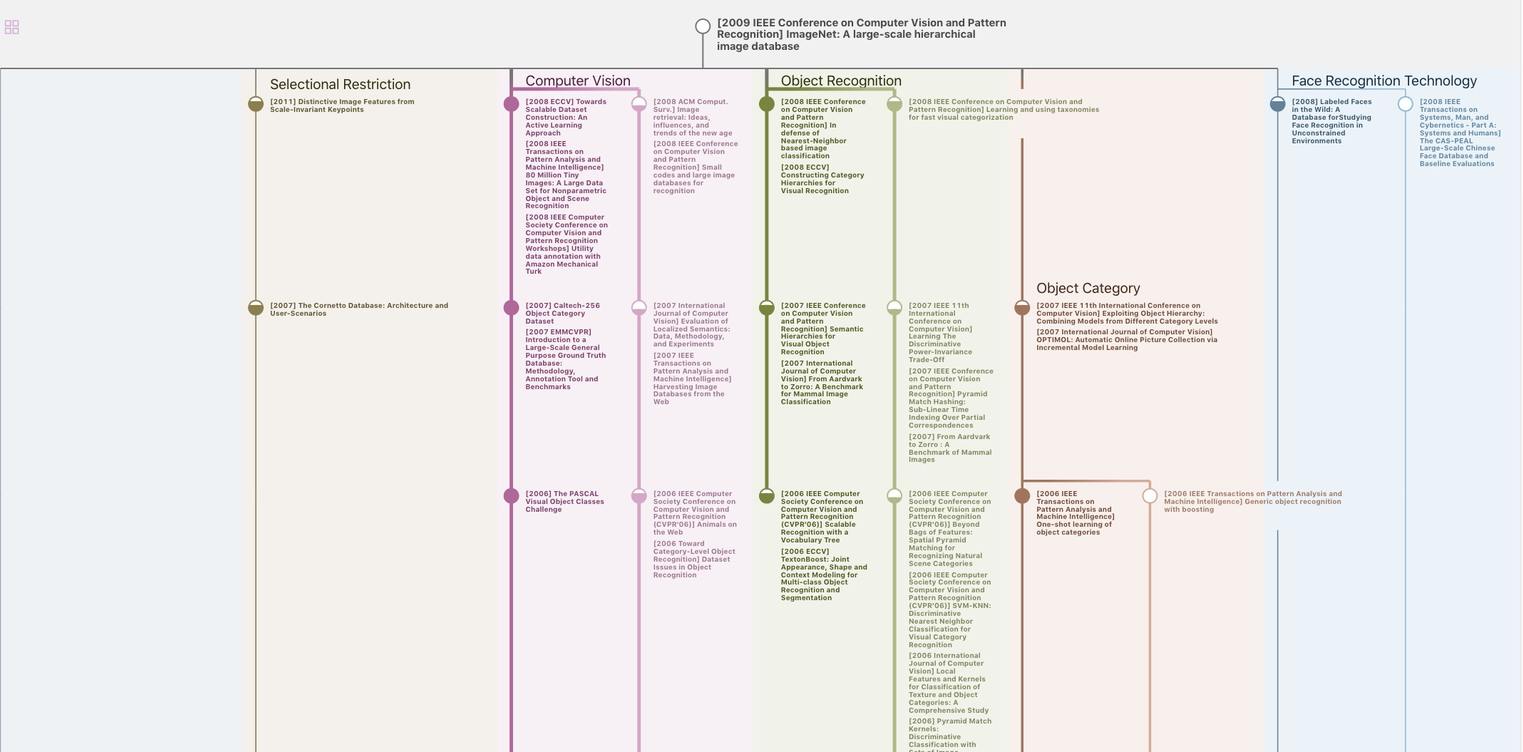
生成溯源树,研究论文发展脉络
Chat Paper
正在生成论文摘要