Rethinking Stability for Attribution-based Explanations
arxiv(2022)
摘要
As attribution-based explanation methods are increasingly used to establish model trustworthiness in high-stakes situations, it is critical to ensure that these explanations are stable, e.g., robust to infinitesimal perturbations to an input. However, previous works have shown that state-of-the-art explanation methods generate unstable explanations. Here, we introduce metrics to quantify the stability of an explanation and show that several popular explanation methods are unstable. In particular, we propose new Relative Stability metrics that measure the change in output explanation with respect to change in input, model representation, or output of the underlying predictor. Finally, our experimental evaluation with three real-world datasets demonstrates interesting insights for seven explanation methods and different stability metrics.
更多查看译文
关键词
explanations,stability,attribution-based
AI 理解论文
溯源树
样例
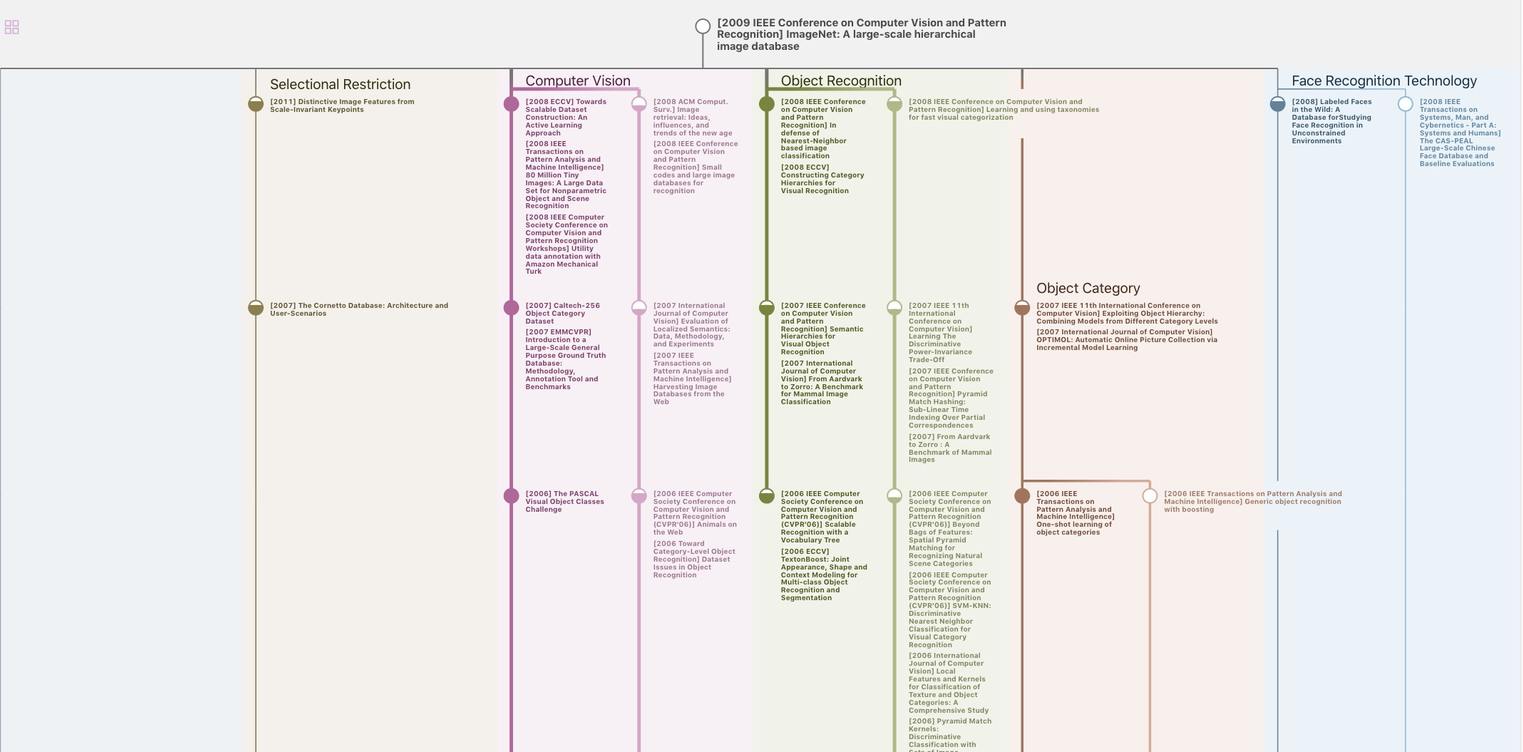
生成溯源树,研究论文发展脉络
Chat Paper
正在生成论文摘要