Continual Prompt Tuning for Dialog State Tracking
PROCEEDINGS OF THE 60TH ANNUAL MEETING OF THE ASSOCIATION FOR COMPUTATIONAL LINGUISTICS (ACL 2022), VOL 1: (LONG PAPERS)(2022)
摘要
A desirable dialog system should be able to continually learn new skills without forgetting old ones, and thereby adapt to new domains or tasks in its life cycle. However, continually training a model often leads to a well-known catastrophic forgetting issue. In this paper, we present Continual Prompt Tuning, a parameter-efficient framework that not only avoids forgetting but also enables knowledge transfer between tasks. To avoid forgetting, we only learn and store a few prompt tokens' embeddings for each task while freezing the backbone pre-trained model. To achieve bi-directional knowledge transfer among tasks, we propose several techniques (continual prompt initialization, query fusion, and memory replay) to transfer knowledge from preceding tasks and a memory-guided technique to transfer knowledge from subsequent tasks. Extensive experiments demonstrate the effectiveness and efficiency of our proposed method on continual learning for dialog state tracking, compared with state-of-the-art baselines.
更多查看译文
关键词
dialog state tracking,continual prompt tuning
AI 理解论文
溯源树
样例
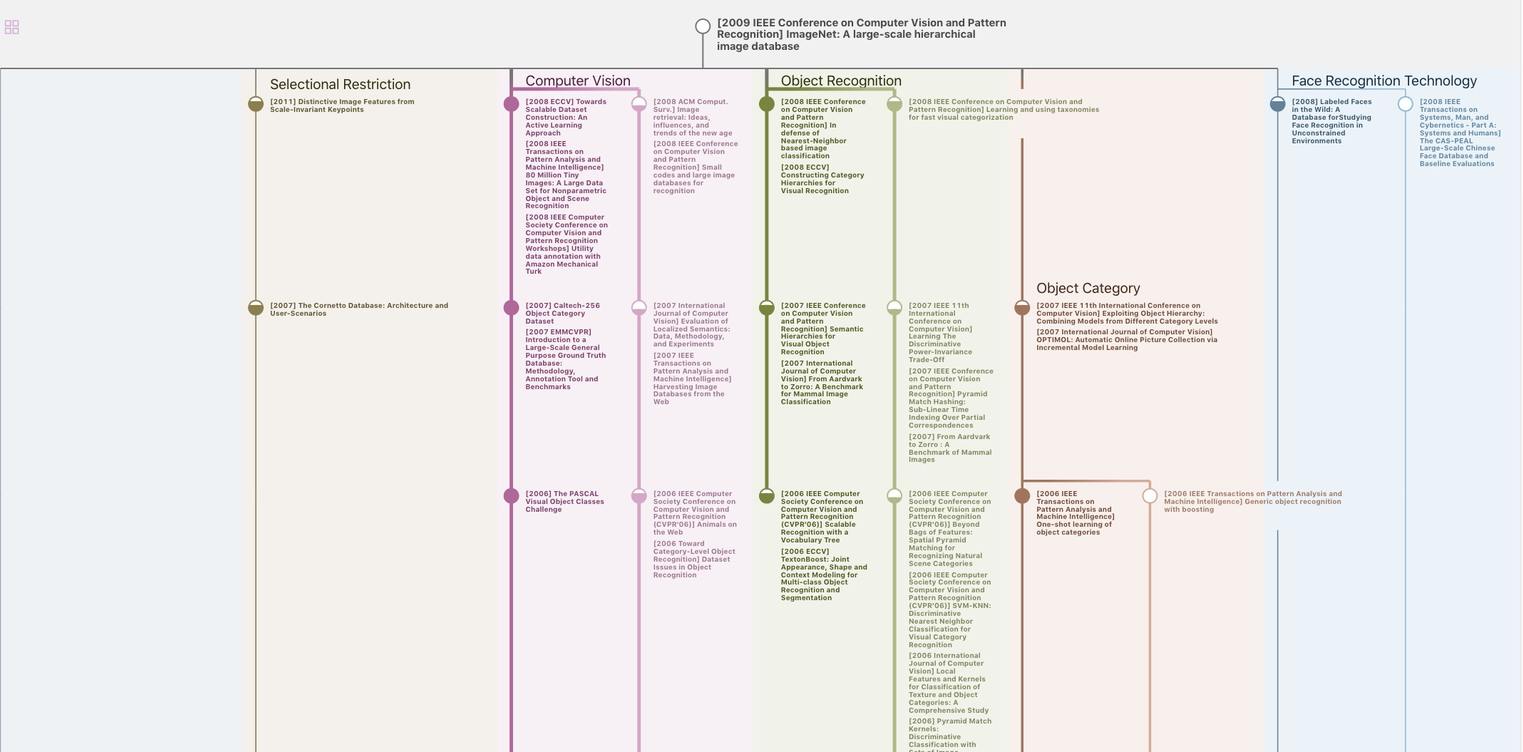
生成溯源树,研究论文发展脉络
Chat Paper
正在生成论文摘要