Homogeneity and Sub-homogeneity Pursuit: Iterative Complement Clustering PCA
arxiv(2022)
摘要
Principal component analysis (PCA), the most popular dimension-reduction technique, has been used to analyze high-dimensional data in many areas. It discovers the homogeneity within the data and creates a reduced feature space to capture as much information as possible from the original data. However, in the presence of a group structure of the data, PCA often fails to identify the group-specific pattern, which is known as sub-homogeneity in this study. Group-specific information that is missed can result in an unsatisfactory representation of the data from a particular group. It is important to capture both homogeneity and sub-homogeneity in high-dimensional data analysis, but this poses a great challenge. In this study, we propose a novel iterative complement-clustering principal component analysis (CPCA) to iteratively estimate the homogeneity and sub-homogeneity. A principal component regression based clustering method is also introduced to provide reliable information about clusters. Theoretically, this study shows that our proposed clustering approach can correctly identify the cluster membership under certain conditions. The simulation study and real analysis of the stock return data confirm the superior performance of our proposed methods.
更多查看译文
关键词
iterative complement clustering
AI 理解论文
溯源树
样例
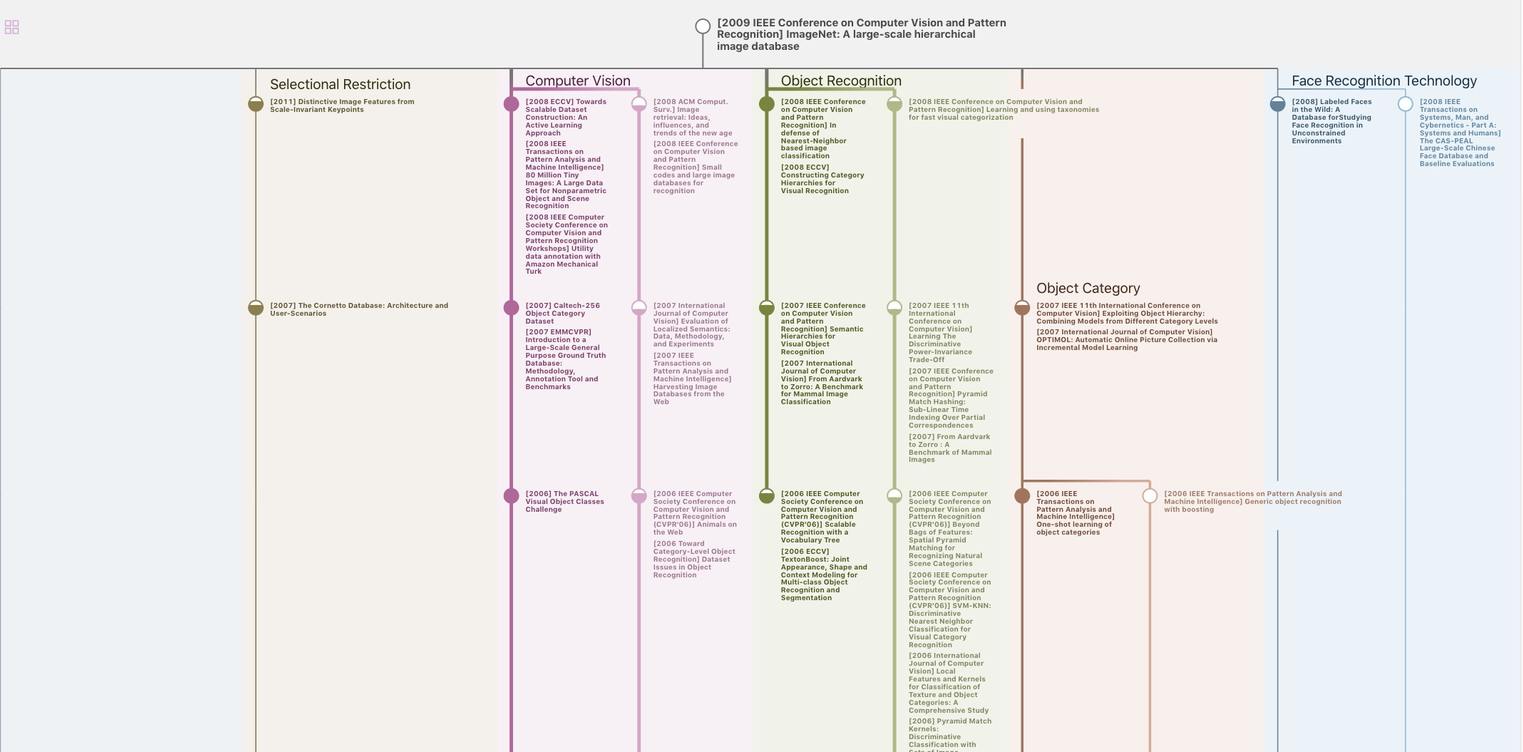
生成溯源树,研究论文发展脉络
Chat Paper
正在生成论文摘要