FiNER: Financial Numeric Entity Recognition for XBRL Tagging
PROCEEDINGS OF THE 60TH ANNUAL MEETING OF THE ASSOCIATION FOR COMPUTATIONAL LINGUISTICS (ACL 2022), VOL 1: (LONG PAPERS)(2022)
摘要
Publicly traded companies are required to submit periodic reports with eXtensive Business Reporting Language (XBRL) word-level tags. Manually tagging the reports is tedious and costly. We, therefore, introduce XBRL tagging as a new entity extraction task for the financial domain and release FINER-139, a dataset of 1.1M sentences with gold XBRL tags. Unlike typical entity extraction datasets, FINER-139 uses a much larger label set of 139 entity types. Most annotated tokens are numeric, with the correct tag per token depending mostly on context, rather than the token itself. We show that subword fragmentation of numeric expressions harms BERT's performance, allowing word-level BILSTMS to perform better. To improve BERT's performance, we propose two simple and effective solutions that replace numeric expressions with pseudo-tokens reflecting original token shapes and numeric magnitudes. We also experiment with FIN-BERT, an existing BERT model for the financial domain, and release our own BERT (SEC-BERT), pre-trained on financial filings, which performs best. Through data and error analysis, we finally identify possible limitations to inspire future work on XBRL tagging.
更多查看译文
关键词
financial numeric entity recognition
AI 理解论文
溯源树
样例
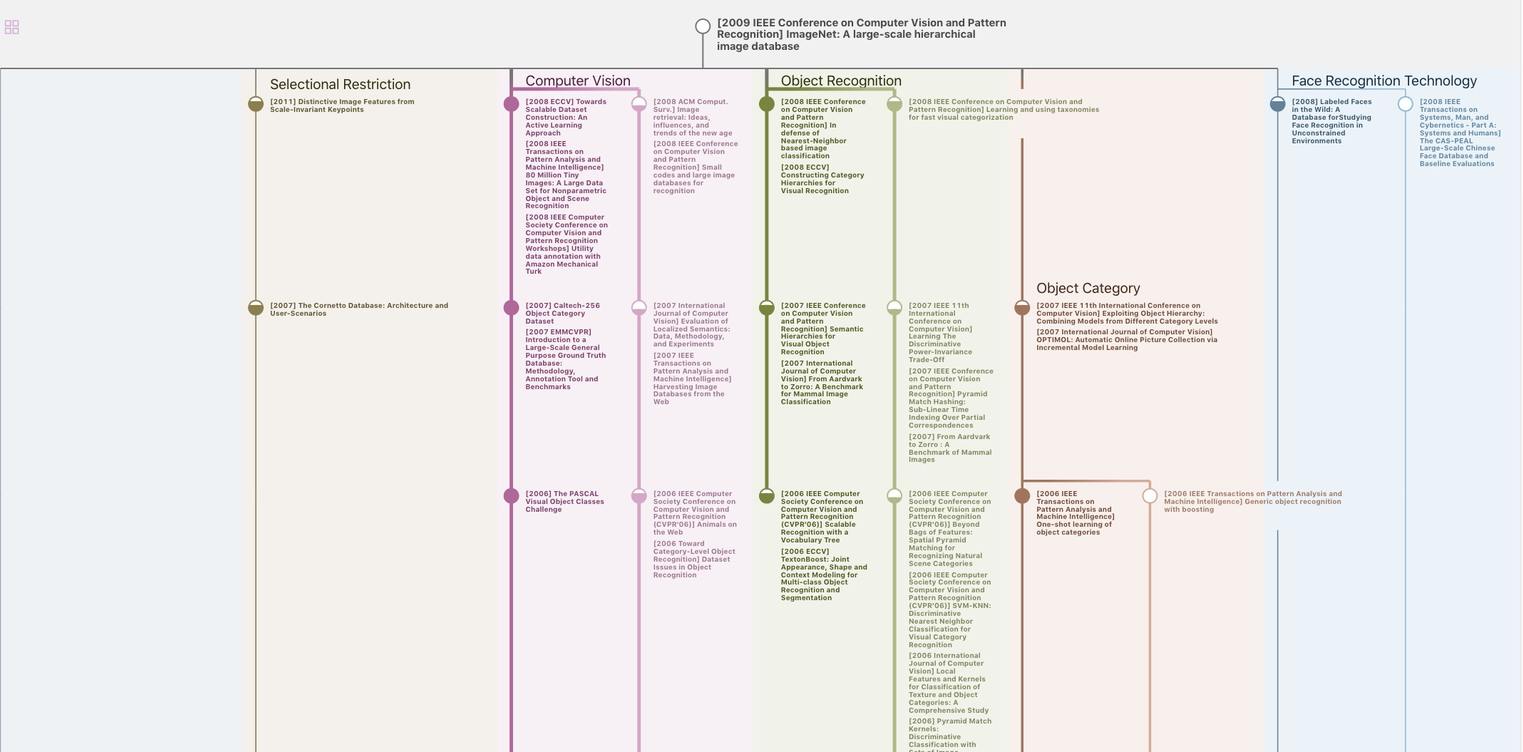
生成溯源树,研究论文发展脉络
Chat Paper
正在生成论文摘要