Sampling Bias Correction for Supervised Machine Learning: A Bayesian Inference Approach with Practical Applications
arxiv(2022)
摘要
Given a supervised machine learning problem where the training set has been subject to a known sampling bias, how can a model be trained to fit the original dataset? We achieve this through the Bayesian inference framework by altering the posterior distribution to account for the sampling function. We then apply this solution to binary logistic regression, and discuss scenarios where a dataset might be subject to intentional sample bias such as label imbalance. This technique is widely applicable for statistical inference on big data, from the medical sciences to image recognition to marketing. Familiarity with it will give the practitioner tools to improve their inference pipeline from data collection to model selection.
更多查看译文
关键词
supervised machine learning,bias,bayesian
AI 理解论文
溯源树
样例
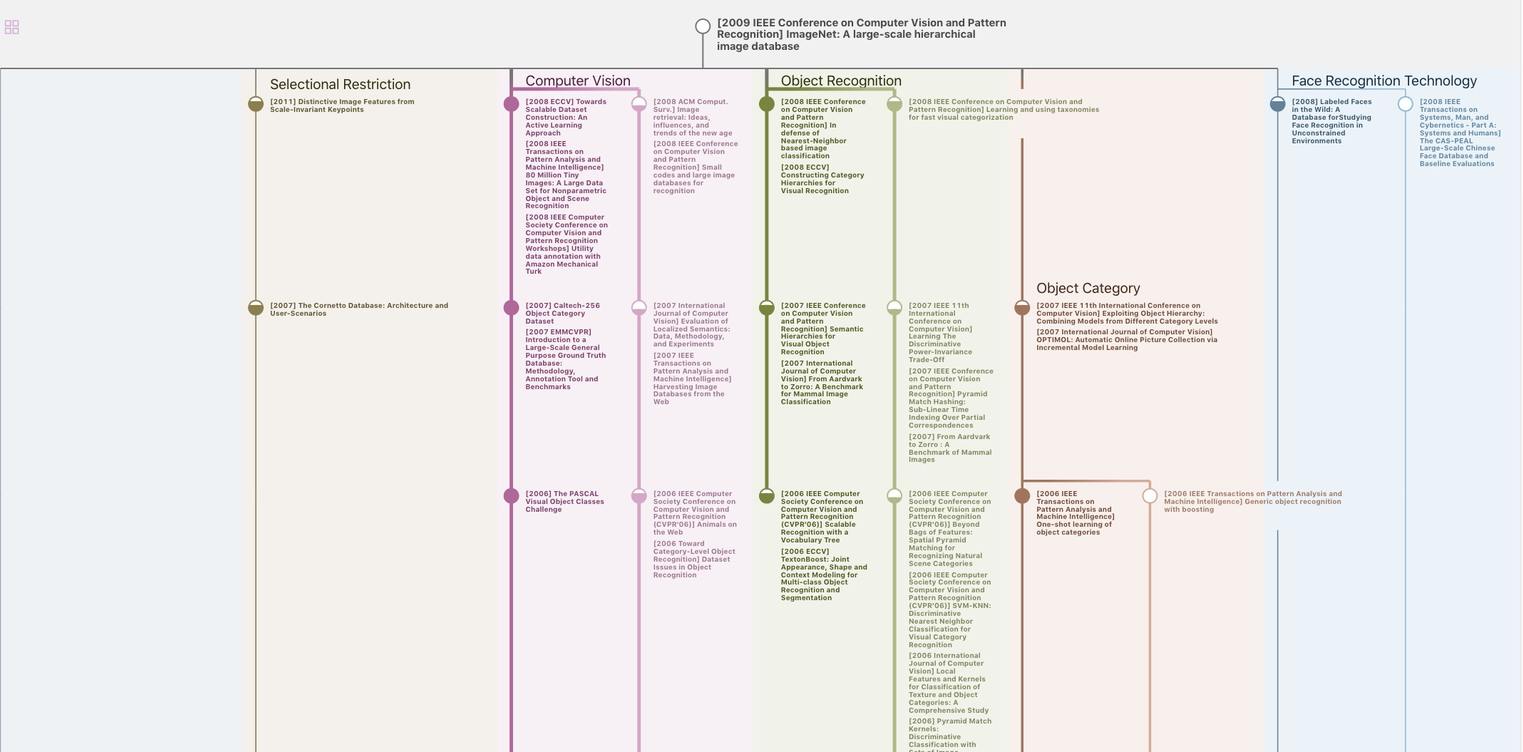
生成溯源树,研究论文发展脉络
Chat Paper
正在生成论文摘要