Nondestructive discrimination of seedless from seeded watermelon seeds by using multivariate and deep learning image analysis
COMPUTERS AND ELECTRONICS IN AGRICULTURE(2022)
摘要
Watermelon cultivators often encounter various challenges of the varietal mixing of triploid, diploid, and tetraploid seeds, thus hindering the watermelon industry due to the uncertainty in the ploidy seed nomenclature. These circumstances indirectly impose negative effects on the income of farmers and the development of companies specializing in watermelon seeds. Therefore, high seed purity is a necessity for all seed breeders and firms, as the performance of a given seed variety can be standardized. In this study, we employed machine vision techniques to classify triploid watermelon seeds from diploid and tetraploid seeds. The major objective of the research was to illustrate the potential of the discrimination of triploid watermelon seeds with multivariate machine learning classification, and, thereafter, deep learning techniques. Watermelon ploidy seed images were acquired by RGB camera, and discrimination models were constructed with multivariate machine learning methods using one-class classification with the DD-SIMCA and SVM quadratic methods. One-class classification with the DD-SIMCA and the SVM-quadratic models yielded triploid discrimination accuracies of 69.5% and 84.3%, respectively. To further improve the ploidy-class discrimination accuracy, deeplabv3 + and Resnet18 deep learning models produced accuracy of 95.5%. The deep learning model results demonstrated a higher discrimination accuracy, and, thus, these results show the potential for automation and application to online systems for real-time ploidy seed discrimination and sorting.
更多查看译文
关键词
Watermelon,Seed purity,Triploid seeds,Image analysis,Deep learning
AI 理解论文
溯源树
样例
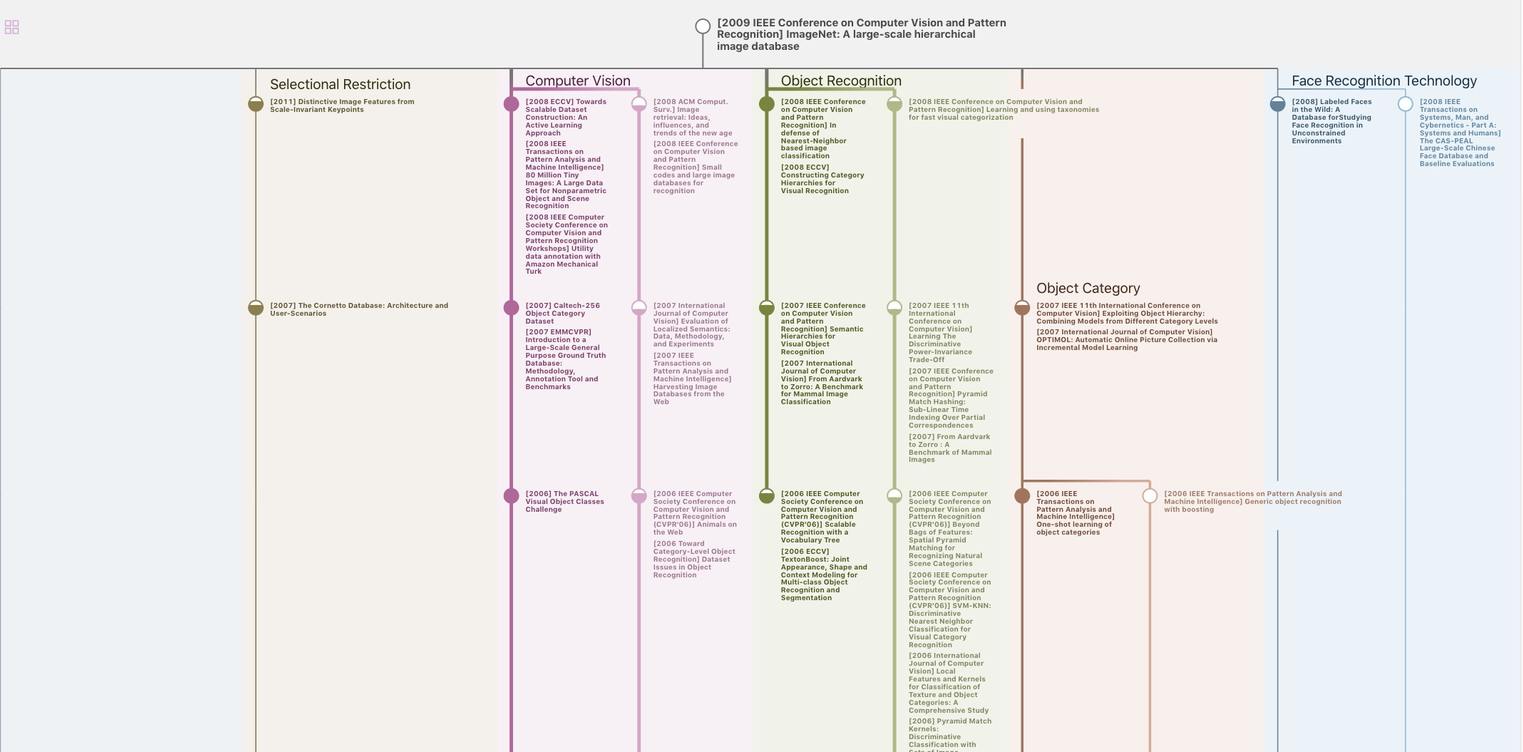
生成溯源树,研究论文发展脉络
Chat Paper
正在生成论文摘要