Co-optimization strategy of unmanned hybrid electric tracked vehicle combining eco-driving and simultaneous energy management
ENERGY(2022)
摘要
Combining eco-driving optimization and simultaneous proper energy management, this paper proposes an efficient co-optimization strategy of unmanned hybrid electric tracked vehicles (HETVs) based on a hierarchical control framework to achieve accurate path tracking and optimal energy management simultaneously. Constrained by a pre-known reference path, a deep Q-learning (DQL) algorithm with the AMSGrad optimizer is designed in the upper layer to optimize the velocity of both side tracks to find the best trade-off between energy economy and accurate path tracking. Based on the optimal velocity profile obtained from the upper layer, an explicit model predictive control method is designed in the lower layer to distribute the power between the engine generator and battery in real time to achieve approximate optimal fuel economy. Simulation results verify that the designed DQL method only requires 0.67 s on average for real-time velocity planning, which is markedly lower than the dynamic programming algorithm. In addition, the proposed method also exhibits higher rapidity and optimality for velocity planning than the traditional DQL algorithm. Compared with the model predictive control, dynamic programming and a process without velocity planning, the proposed co-optimization strategy achieves good fuel economy, accurate path tracking and high computational efficiency.(c) 2022 Published by Elsevier Ltd.
更多查看译文
关键词
Co-optimization strategy,Velocity planning,Energy management,HET V,optimizer,Explicit model predictive control,Deep Q-learning algorithm with AMSGrad
AI 理解论文
溯源树
样例
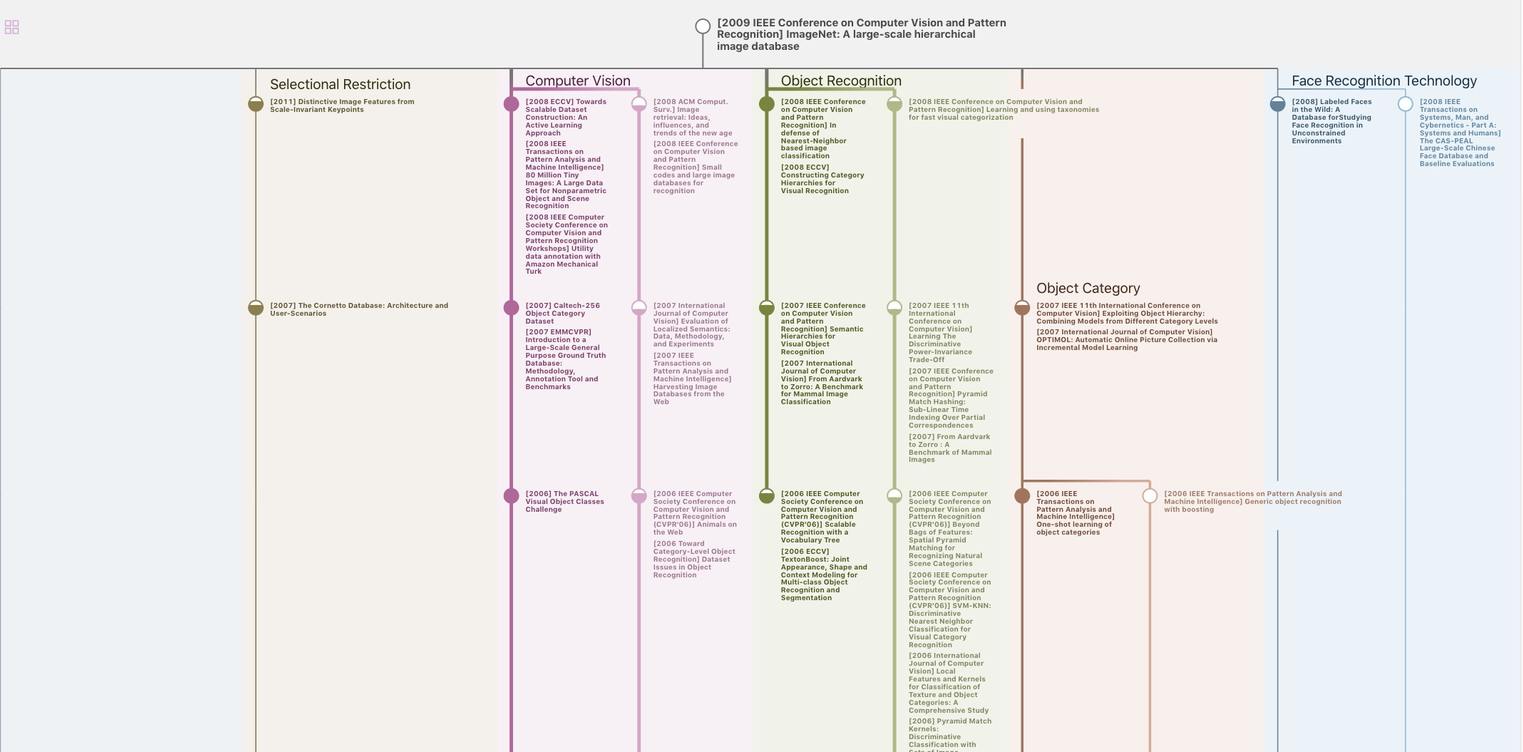
生成溯源树,研究论文发展脉络
Chat Paper
正在生成论文摘要