An empirical Bayes approach to incorporating demand intermittency and irregularity into inventory control
EUROPEAN JOURNAL OF OPERATIONAL RESEARCH(2022)
摘要
Spare parts inventory management is complex due to the combined impact of intermittent and vari-able demand patterns. It becomes even more challenging if the spare parts demand distribution is highly complex due to strong interdependent demand intermittency and extremely irregular demand. The re-search literature proposes many analytical methods for forecasting spare parts demand. But, due to their limited flexibility in modeling complex demand patterns, existing forecasting methods may not produce satisfactory results for a spare parts portfolio displaying extremely complex demand patterns. This study proposes a novel nonparametric Bayesian forecasting approach with its roots in the empirical Bayes paradigm. The method is subject to few performance constraints and is highly flexible in dealing with a rich diversity of demand patterns, including extreme demand complexity. We assess the relative per-formance of this new approach with several prominent methods in the literature using an automotive parts distributor's empirical demand data for 46,272 stock-keeping units. This dataset is representative of typical spare parts portfolios that are characterized by a wide variety and extremely complex demand patterns. The experimental findings show the new Bayesian approach achieves the best overall perfor-mance in terms of inventory efficiency and minimal backorders for meeting specified target service lev -els. This favorable performance reflects the approach's flexibility to accommodate disparate and complex demand patterns, including interdependence of demand intermittency, irregular demand distribution, and even nonstationary demand distribution to some extent, and provide robust solutions. Published by Elsevier B.V.
更多查看译文
关键词
Forecasting,Inventory management,Spare parts,Empirical Bayes
AI 理解论文
溯源树
样例
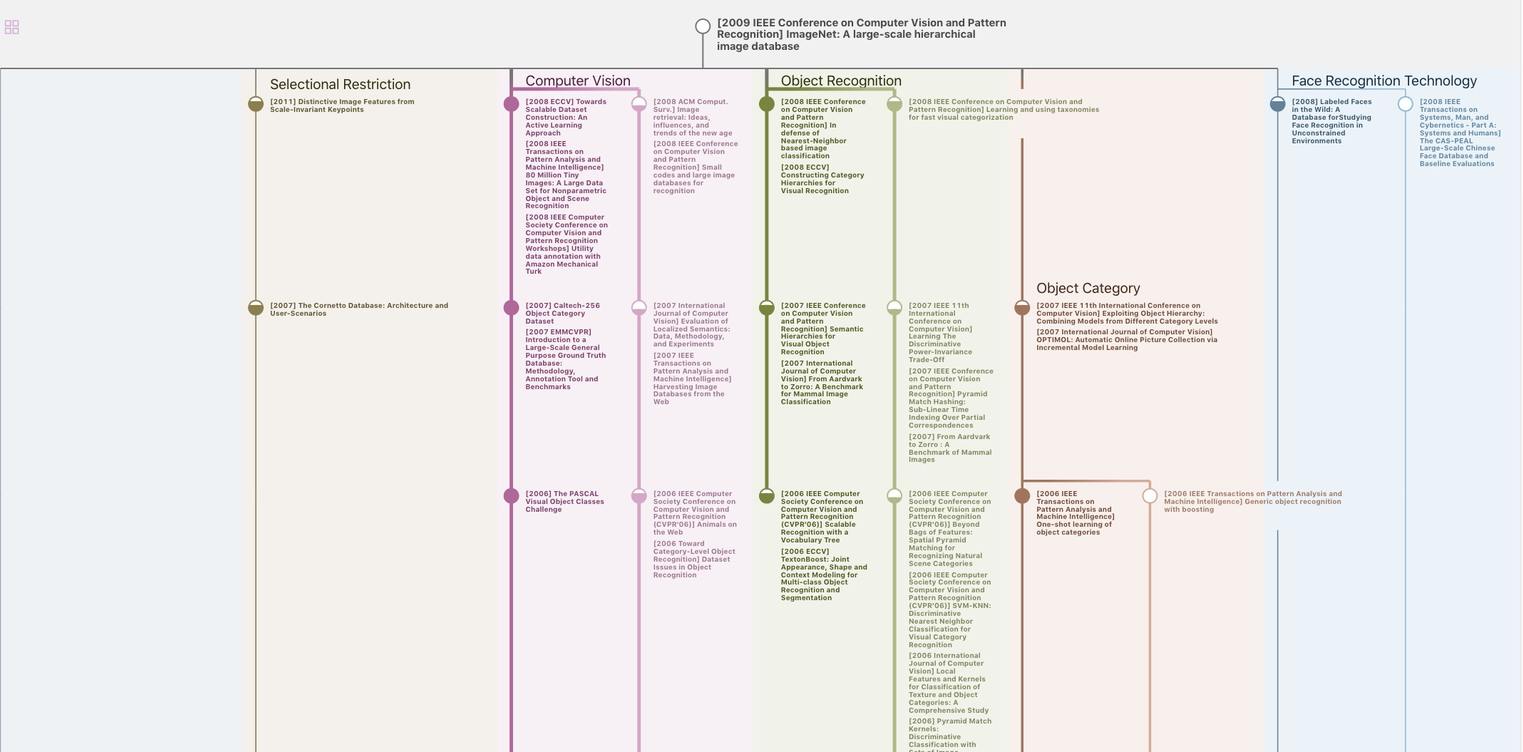
生成溯源树,研究论文发展脉络
Chat Paper
正在生成论文摘要