A generalised smoothing approach for continuous, planar, inverse kinematics problems
Journal of Biomechanics(2022)
摘要
Bayesian methods have recently been proposed to solve inverse kinematics problems for marker based motion capture. The objective is to find the posterior distribution, a probabilistic summary of our knowledge and corresponding uncertainty about the model parameters such as joint angles, segment angles, segment translations, and marker positions. To date, Bayesian inverse kinematics models have focused on a frame by frame solution, which if repeatedly applied gives estimates that are discontinuous in time. We propose to overcome this limitation for continuous, planar inverse kinematics problems via the use of finite basis representations to model latent kinematic quantities as smooth, continuous functions. Our generalised smoothing approach is able to accurately approximate the solution to planar inverse kinematics problems defined by simple systems of ordinary differential equations in addition to considerably more complex systems such as a planar analysis of human gait. Improvements in accuracy are considerable with a decrease in average RMSE of 0.025 rad observed when estimating ankle joint angle for a randomly selected running stride with the proposed generalised smoothing approach compared to previous time-independent approaches. In addition, the generalised smoothing approach is able to effectively estimate kinematic parameters in the presence of missing data along with derivatives of kinematic quantities without the need for prior filtering or gap-filling of data.
更多查看译文
关键词
Bayesian inference,Measurement error,Optical motion capture,Ordinary differential equations,Functional data analysis,Generalised additive models
AI 理解论文
溯源树
样例
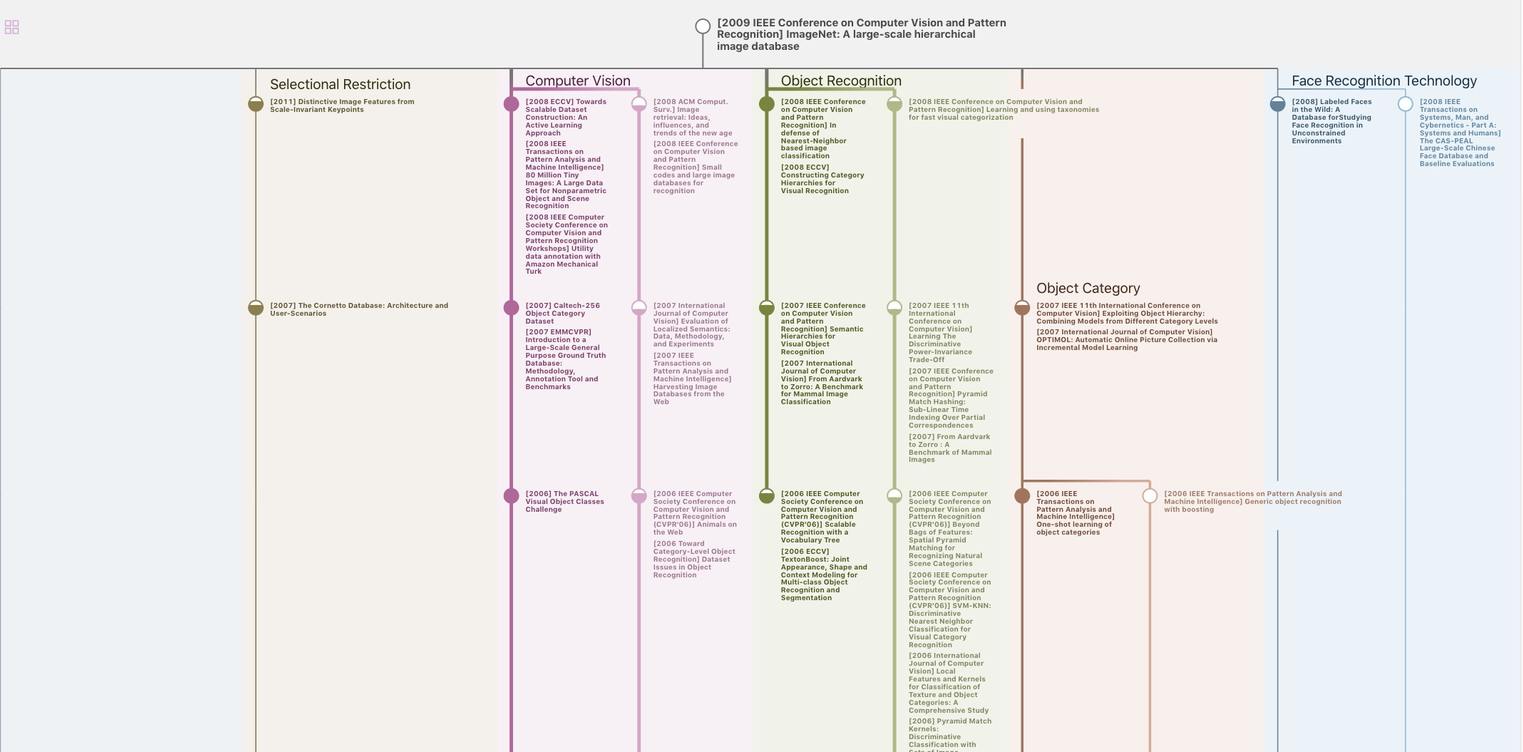
生成溯源树,研究论文发展脉络
Chat Paper
正在生成论文摘要