Salient Regions and Hierarchical Indexing for Crop Disease Images
INTERNATIONAL JOURNAL OF PATTERN RECOGNITION AND ARTIFICIAL INTELLIGENCE(2022)
摘要
With the development of modern agricultural facilities, crop diseases recognition, nutritional status and morphology achieved rapid growth. To avoid yield loss caused by the delay of disease detection, digital images that contain information with respect to crop growth, disease type and nutrition deficiency have been studied by some researchers. However, traditional image processing methods fail to extract typical disease features of crop images with ambiguous disease information. In this paper, a crop disease image recognition technique based on the salient region and hierarchical indexing was proposed. Improved Harris algorithm and maximum radius were used to calculate the widest salient region. In order to eliminate the effect of different salience distribution ranges between different features, a group of images in the cucumber disease image library were normalized. Experiment results indicate that the time complexity of each algorithm will go up as the size of the dataset increase. Especially when testing large datasets, nonhierarchical and nonclustering, hierarchical and nonclustering and hierarchical based on points all tend to raise the algorithm's time complexity. Plant Village dataset and AI Challenger 2018 dataset were utilized to compare the recognition performances among the models based on machine learning, neural network, deep learning and our methods. The experiment results show that the method proposed in this paper is capable of recognizing local similar images effectively rather than global similar images, therefore, it has better recognition performance than the model learning methods in the early detection stage of crop disease.
更多查看译文
关键词
Salient regions,dynamic weight,hierarchical indexing,clustering,similarity search
AI 理解论文
溯源树
样例
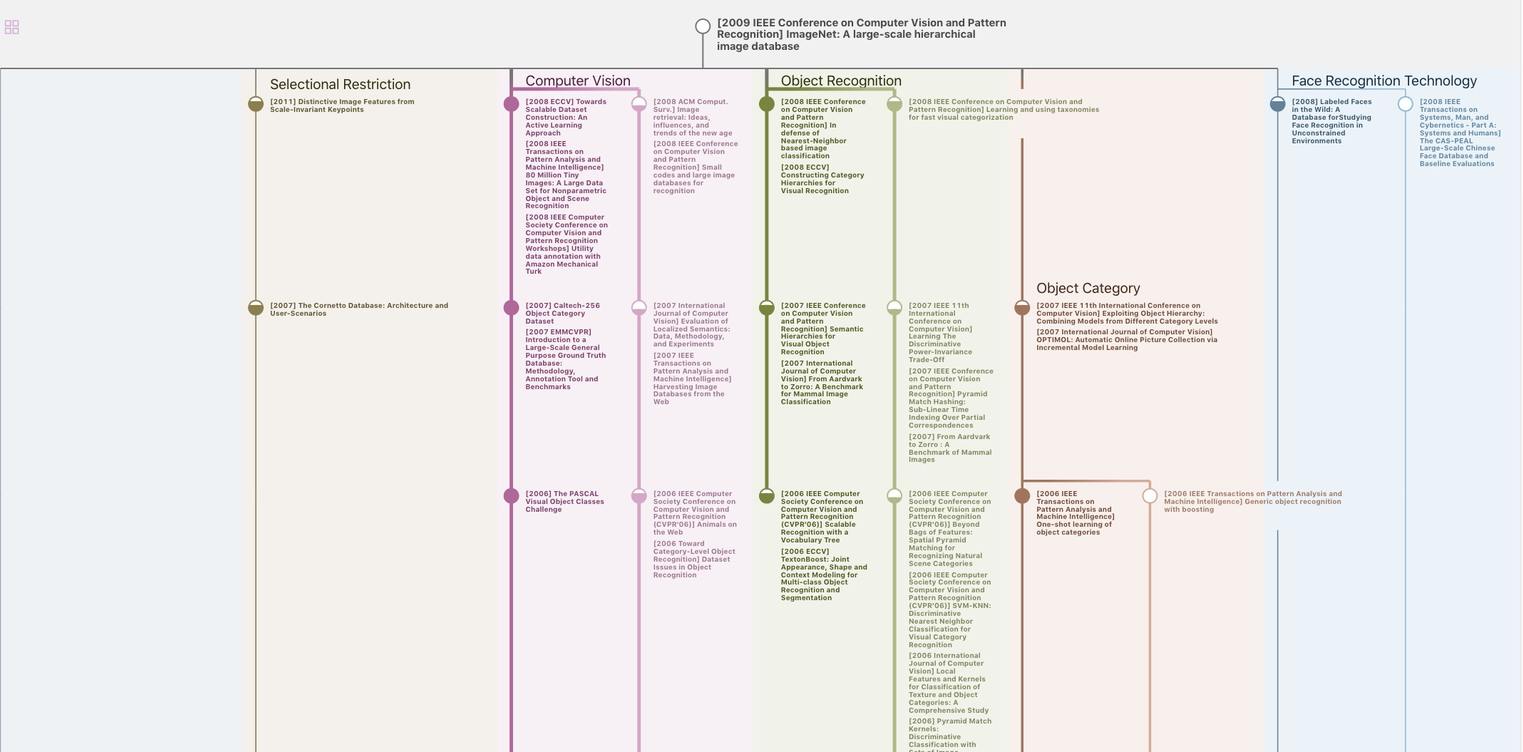
生成溯源树,研究论文发展脉络
Chat Paper
正在生成论文摘要