Unsupervised Machine Learning-based EV Load Profile Generation for Efficient Distribution System Operation
CIRED 2021 - The 26th International Conference and Exhibition on Electricity Distribution(2021)
摘要
With the popularization of EVs, learning the charging load patterns is becoming a key step for the stability of power grids. However, the randomly probable nature of EVs has brought new challenges to the scheduling, operation and planning of distribution system. Also, EV load patterns can be different from locations such as in areas where offices are located and in areas which makes it more complicated to generate EV load profiles. In this paper, we propose machine learning based EV profiling technique to better understand the information behind the random probability and irregularity of EV load. The proposed method is able to take into account the time-varying, dynamic character and the complex temporal correlation of EV loads. Through the proposed method, we develop reliable probabilistic models and generate a series of different EV load profiles. The simulation results show that EV load profiles generated by the proposed method can not only retain the temporal correlation and probability distribution nature, but also improve the accuracy of load forecast. As a result, considering a series of different EV load profiles generated by our proposed methods, the distribution system operation can take uncertainty into account when making decisions, such as safe operation of distribution network, optimal economic trade and cooperative scheduling strategy.
更多查看译文
关键词
ev load profile generation,distribution,learning-based
AI 理解论文
溯源树
样例
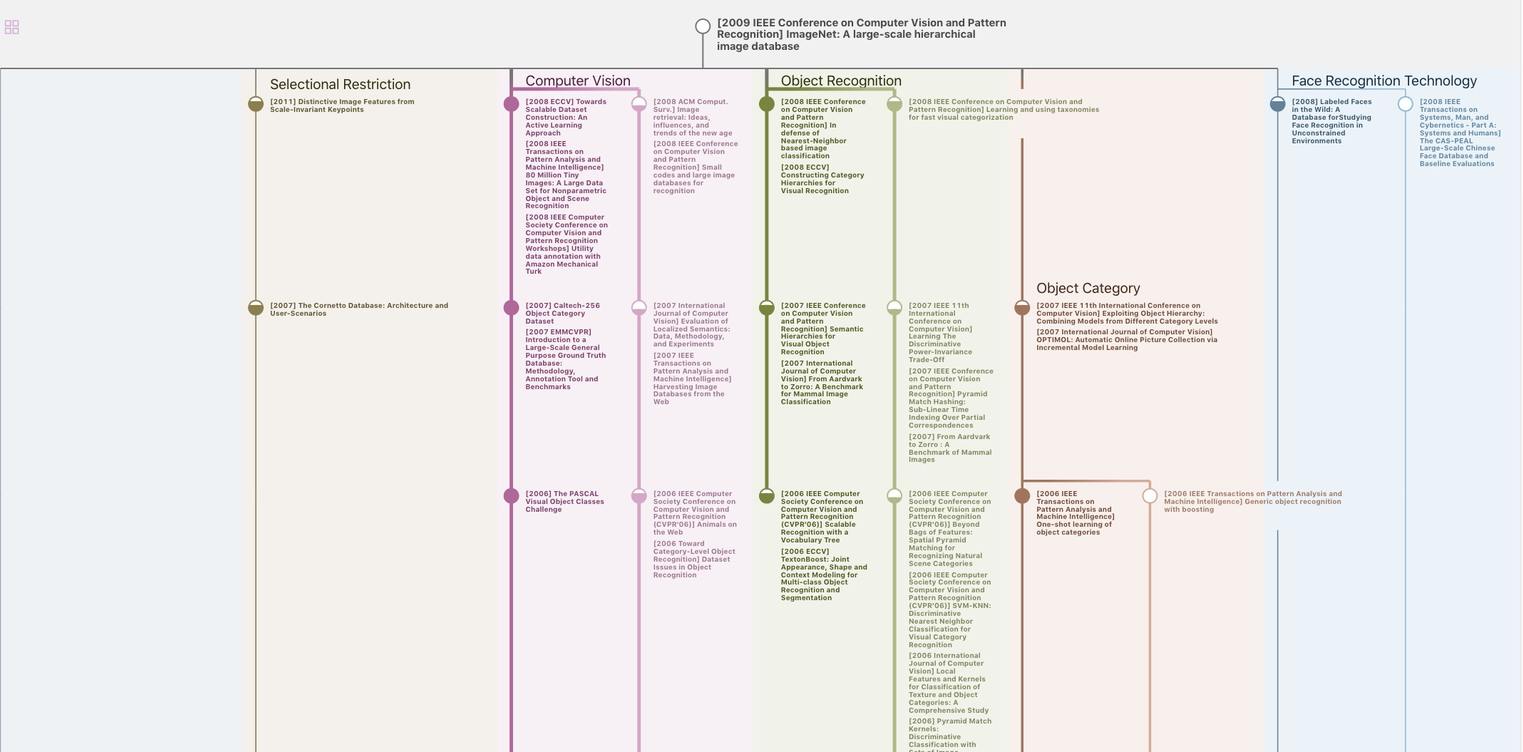
生成溯源树,研究论文发展脉络
Chat Paper
正在生成论文摘要