A differentially private indoor localization scheme with fusion of WiFi and bluetooth fingerprints in edge computing
Neural Computing and Applications(2022)
摘要
an enabling technology for edge computing scenarios, indoor localization has a broad prospect in a variety of location-based applications, such as tracking, navigating, and monitoring in indoor environments. In order to improve the location accuracy, numerous machine learning (ML)-based indoor localization schemes with fingerprint fusion have been proposed recently, which take advantage of the fusion of signal gathered from multiple wireless technologies (e.g., WiFi and BLE) and require a site survey to construct the fingerprint database. However, most solutions are based on cloud framework and thus pose a serious privacy leakage because users’ sensitive information (e.g., locations) is computed from the fingerprint database by the untrusted localization service provider. Furthermore, the site survey is time-consuming and labor-intensive. In this paper, we propose a differentially private fingerprint fusion semi-supervised extreme learning machine for indoor localization in the edge computing, called Adp-FSELM. The Adp-FSELM firstly employs a multi-level edge network-based privacy-preserving system framework to meet the requirements of ML-based fingerprint indoor localization for lightweight, low latency, and real-time response. Then, the Adp-FSELM extends the ε -differential privacy to the fingerprint fusion semi-supervised extreme learning machine for indoor localization in edge computing through a three-phase private process consisting of private labeled sample obfuscation, differentially private feature fusion, and differentially private model training. Theoretical and comprehensive experimental results in real indoor environments demonstrate that the Adp-FSELM provides a high ε -differential privacy guarantee for users’ location privacy while reducing human calibration effort and effectively resists Bayesian inference attacks. Compared with the existing semi-supervised learning-based localization methods, the mean absolute error of location accuracy of the Adp-FSELM is restricted to 2.22
更多查看译文
关键词
Location privacy,Edge computing,Differential privacy,Fusion semi-supervised extreme learning machine,Indoor localization
AI 理解论文
溯源树
样例
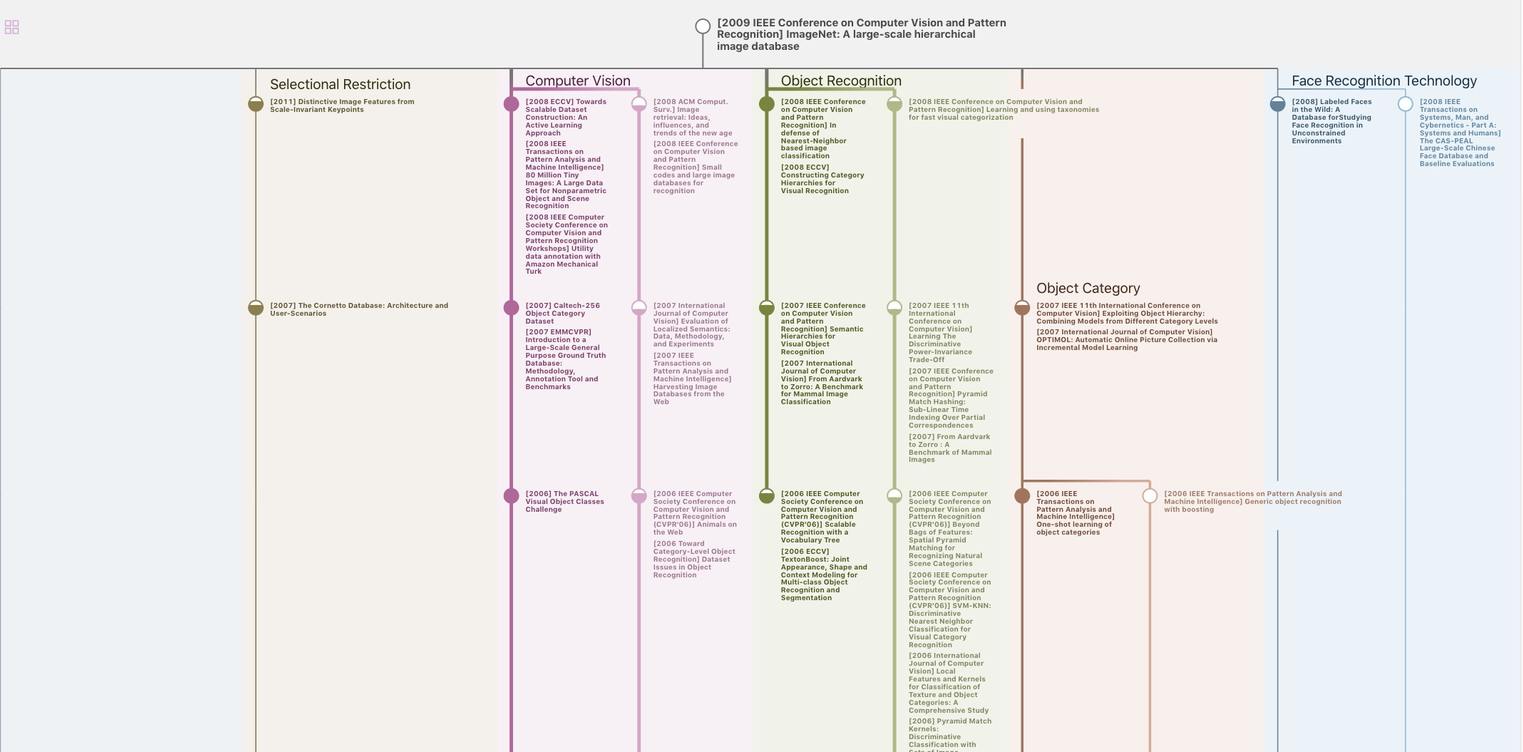
生成溯源树,研究论文发展脉络
Chat Paper
正在生成论文摘要