Experimental Study on the Effectiveness of Machine Learning Methods in Web Intrusion Detection
Advances in Information, Communication and CybersecurityLecture Notes in Networks and Systems(2022)
摘要
Web-based attacks have been more sophisticated and hard to detect in recent years, relying on just using traditional intrusion detection systems may not be enough. In this respect, intrusion detection and prevention systems using Machine learning methods have been important in recent literature. In this paper, we present an experimental study on the effectiveness of Machine learning methods in web intrusion detection. In which, We have investigated and compared four types of ML classifiers often used in the cybersecurity domain: KNN, Decision Tree, Multinomial, and Bernoulli Naive Bayes, SVM Linear, Sigmoid, and RBF. The experimental results based on ECML/PKDD 2007 and CSIC HTTP 2010 dataset showed that SVM RBF and Decision Tree classifiers achieved better performance in terms of Accuracy, Recall, Precision, F-value, FPR, and FNR than others.
更多查看译文
关键词
web intrusion detection,machine learning methods,machine learning
AI 理解论文
溯源树
样例
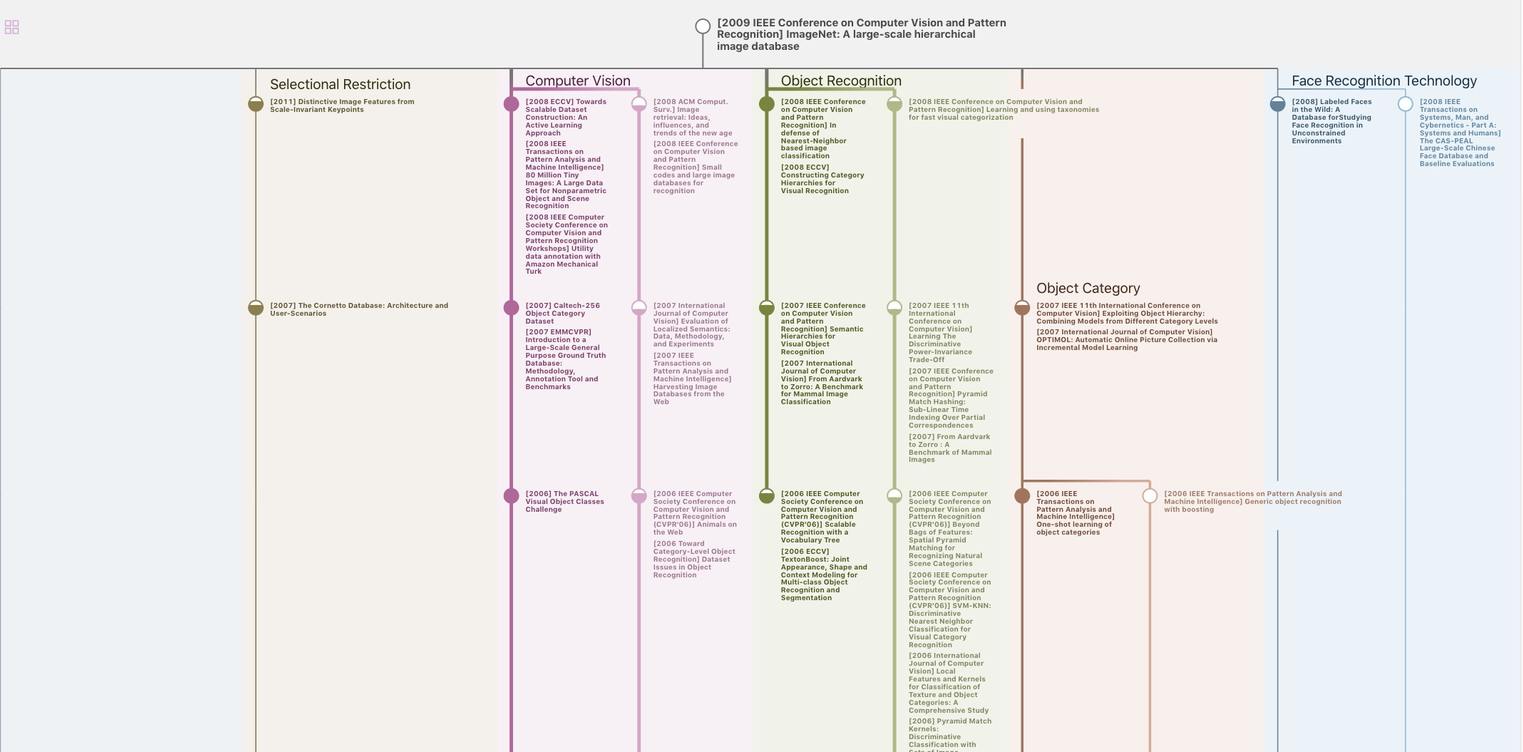
生成溯源树,研究论文发展脉络
Chat Paper
正在生成论文摘要