Prediction of environmental missing data time series by Support Vector Machine Regression and Correlation Dimension estimation
Environmental Modelling & Software(2022)
摘要
Environmental time series are often affected by missing data, namely data unavailability at certain time points. This paper presents the Iterated Imputation and Prediction algorithm, that allows the prediction of time series with missing data. The algorithm uses iteratively the Correlation Dimension Estimation of the underlying dynamic system generating the time series to fix the model order (i.e., how many past samples are required to model the time series accurately), and the Support Vector Machine Regression to estimate the skeleton of time series. Experimental validation of the algorithm on three environmental time series with missing data, expressing the concentration of Ozone in three European sites, shows a small average percentage prediction error for all time series on the test set.
更多查看译文
关键词
Missing data,Model order estimation,Grassberger-procaccia algorithm,Correlation dimension estimation,Hough transform,Support vector machine regression
AI 理解论文
溯源树
样例
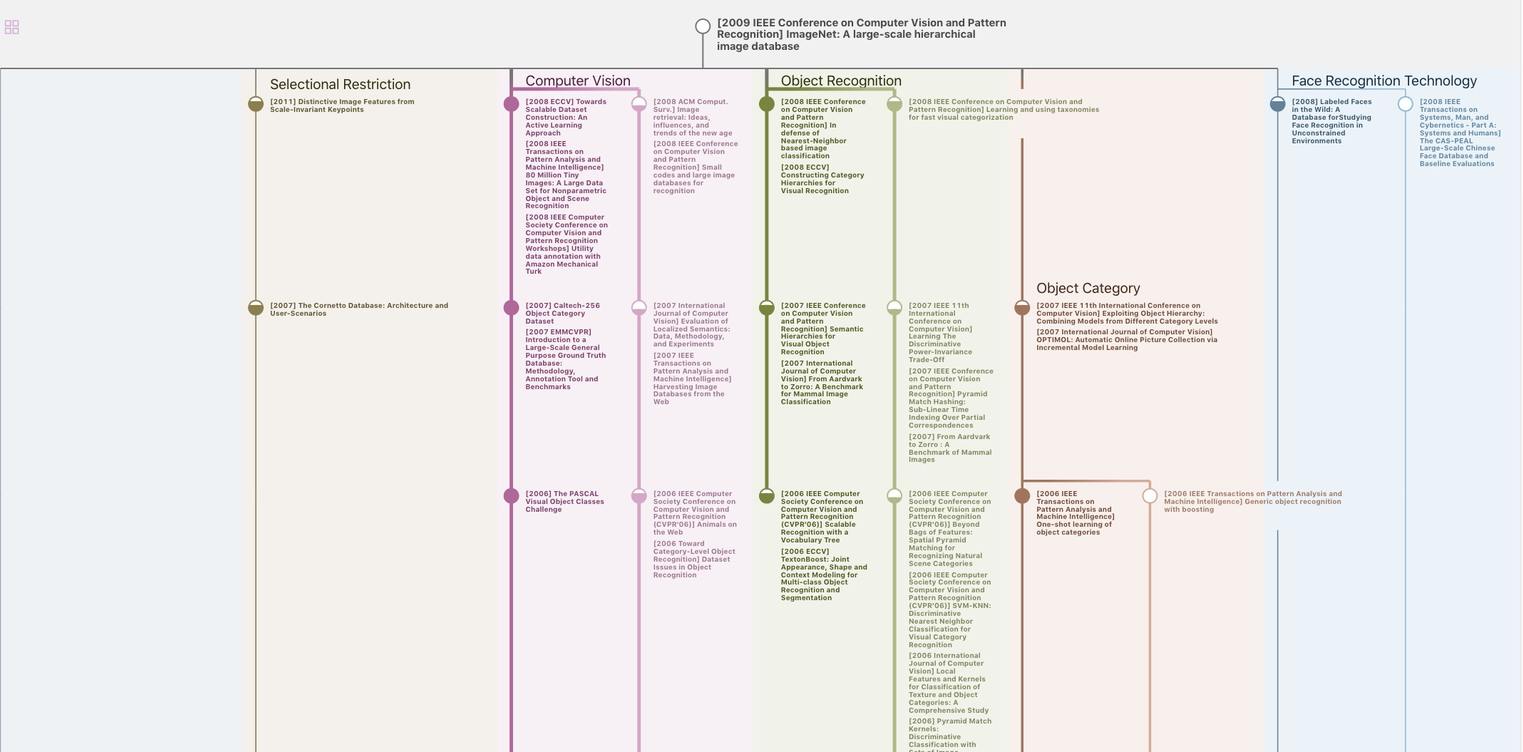
生成溯源树,研究论文发展脉络
Chat Paper
正在生成论文摘要